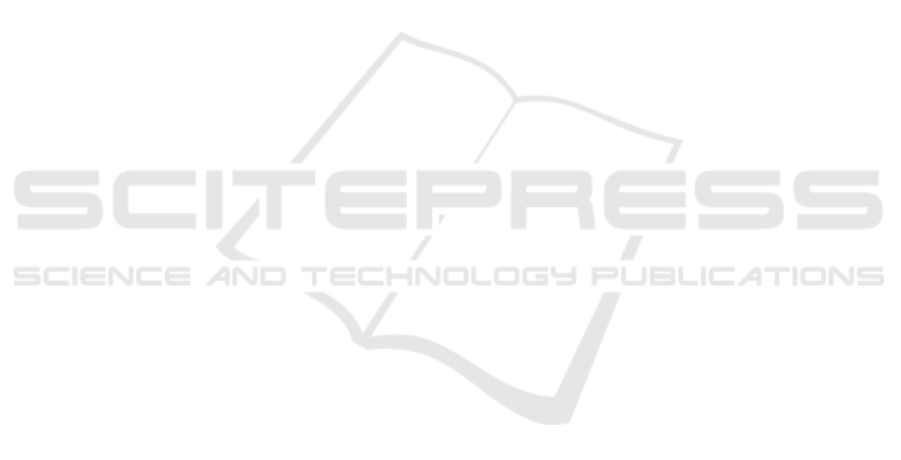
thus very versatile estimator capable of high quality
self-assessment
6 CONCLUSIONS
The paper dealt with the Bayesian state estimation of
nonlinear stochastic dynamic systems and specifically
with the stochastic integration filter, which is based
on a stochastic integration rule. Within the iterative
algorithm of the SIF an instrument for measuring the
nonlinearity was discovered. The instrument uses a
quantity, which is a byproduct of the SIR, which has
been used in the stopping condition so far. Such in-
formation provides an information of how much the
nonlinearity in the system violates the Gaussian as-
sumption of the SIF. The paper also provided a re-
lation that can be used to predict the number of SIR
iterations required to achieve the accuracy requested
by the user.
Further, in the paper a method to calculate im-
proved estimates of the MSE was developed. It was
shown that the MSE contains additional terms besides
the conditional variance matrix provided by the stan-
dard GFs. By utilizing the SIR byproducts the addi-
tional terms can be estimated by interval estimate. By
including the additional term estimates the SIF is able
to provide more accurate information about its state
estimates. Both the new nonlinearity measure and the
improved MSE were illustrated using simple numeri-
cal examples.
The interesting part of the discoveries made in this
paper is that it opens wide area for a future work. The
SIF can be further improved in terms of saving com-
putational demands, when the measurement function
is almost linear, or when the function is strongly non-
linear an execution of the algorithm can be paused and
for example Gaussian sum approach can be adopted
to improve estimate quality.
ACKNOWLEDGEMENTS
This work was supported by the Czech Science Foun-
dation, project no. GA 16-19999J.
REFERENCES
Arasaratnam, I. and Haykin, S. (2009). Cubature Kalman
filters. IEEE Transactions on Automatic Control,
54(6):1254–1269.
Arasaratnam, I., Haykin, S., and Elliott, R. J. (2007).
Discrete-time nonlinear filtering algorithms using
Gauss–Hermite quadrature. Proceedings of the IEEE,
95(5):953–977.
Bates, D. M. and Watts, D. G. (1988). Nonlinear Regression
Analysis and Its Applications. John Wiley & Sons.
Doucet, A., De Freitas, N., and Gordon, N. (2001). Se-
quential Monte Carlo Methods in Practice, chapter
An Introduction to Sequential Monte Carlo Methods.
Springer. (Ed. Doucet A., de Freitas N., and Gordon
N.).
Dun
´
ık, J., Straka, O., and
ˇ
Simandl, M. (2013a). Nonlinear-
ity and non-Gaussianity measures for stochastic dy-
namic systems. In Proceedings of the 16th Interna-
tional Conference on Information Fusion, Istanbul.
Dun
´
ık, J., Straka, O., and
ˇ
Simandl, M. (2013b). Stochas-
tic integration filter. IEEE Transactions on Automatic
Control, 58(6):1561–1566.
Genz, A. and Monahan, J. (1998). Stochastic integration
rules for infinite regions. SIAM Journal on Scientific
Computing, 19(2):426–439.
Ito, K. and Xiong, K. (2000). Gaussian filters for nonlinear
filtering problems. IEEE Transactions on Automatic
Control, 45(5):910–927.
Julier, S. J. and Uhlmann, J. K. (2004). Unscented filtering
and nonlinear estimation. IEEE Review, 92(3):401–
421.
Kramer, S. C. and Sorenson, H. W. (1988). Recursive
Bayesian estimation using piece-wise constant ap-
proximations. Automatica, 24(6):789–801.
Li, X. R. (2012). Measure of nonlinearity for stochastic sys-
tems. In Proceedings of the 15th International Con-
ference on Information Fusion, Singapore.
Mallick, M. (2004). Differential geometry measures of non-
linearity with applications to ground target tracking.
In Proceedings of the 7th International Conference on
Information Fusion, Stockholm, Sweden.
Nørgaard, M., Poulsen, N. K., and Ravn, O. (2000). New
developments in state estimation for nonlinear sys-
tems. Automatica, 36(11):1627–1638.
Ristic, B., Arulampalam, S., and Gordon, N. (2004). Be-
yond the Kalman Filter: Particle Filters for Tracking
Applications. Artech House.
Sorenson, H. W. (1974). On the development of practical
nonlinear filters. Information Sciences, 7:230–270.
On Nonlinearity Measuring Aspects of Stochastic Integration Filter
361