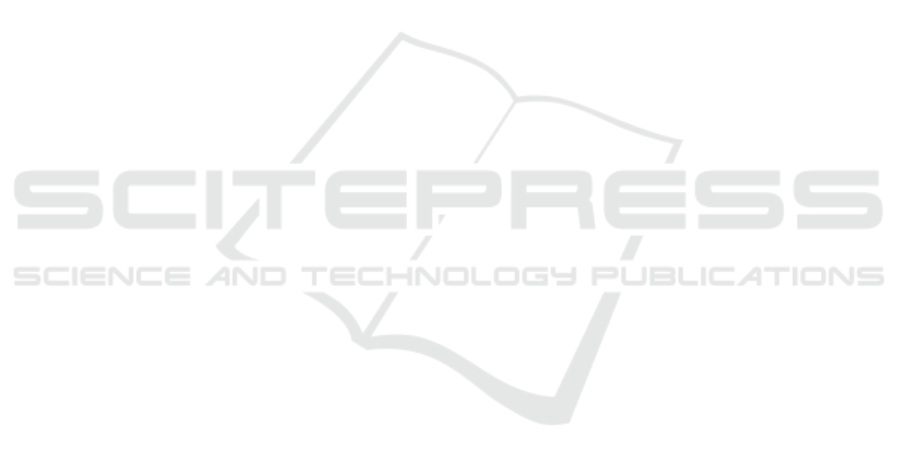
Our experiments have shown that by using only gy-
roscopes as and when needed, and employing the ad-
ditional data from obstacle avoidance sensors, the lo-
cation accuracy is improved significantly. It is seen
that since environments usually have obstacles, and
robots have to navigate around them, using that as
a parameter is an effective approach for localization.
Our approach provides good accuracies at a low over-
head. However when the turns are very sharp, and
acute, our approach suffers. While our approach ac-
counts for gyroscope drift errors, more work needs to
be done to reduce it further for longer distances.
REFERENCES
(2015). bstem developer kit & integrated robotics platform:
www.braincorporation.com.
Ahn, H.-S. and Yu, W. (2007). Indoor mobile robot and
pedestrian localization techniques. In Control, Au-
tomation and Systems, 2007. ICCAS’07. International
Conference on, pages 2350–2354. IEEE.
Borenstein, J. and Feng, L. (1994). Umbmark: a method for
measuring, comparing, and correcting dead-reckoning
errors in mobile robots.
Choi, B.-S., Lee, J.-W., Lee, J.-J., and Park, K.-T. (2011). A
hierarchical algorithm for indoor mobile robot local-
ization using rfid sensor fusion. Industrial Electronics,
IEEE Transactions on, 58(6):2226–2235.
DeSouza, G. N. and Kak, A. C. (2002). Vision for mobile
robot navigation: A survey. Pattern Analysis and Ma-
chine Intelligence, IEEE Transactions on, 24(2):237–
267.
Easton, A. and Cameron, S. (2006). A gaussian error model
for triangulation-based pose estimation using noisy
landmarks. In Robotics, Automation and Mechatron-
ics, 2006 IEEE Conference on, pages 1–6. IEEE.
Elmenreich, W. (2002). Sensor fusion in time-triggered sys-
tems.
Erinc, G. (2013). Appearance-based navigation, localiza-
tion, mapping, and map merging for heterogeneous
teams of robots.
Granados-Cruz, M., Pomarico-Franquiz, J., Shmaliy, Y. S.,
and Morales-Mendoza, L. J. (2014). Triangulation-
based indoor robot localization using extended
fir/kalman filtering. In Electrical Engineering, Com-
puting Science and Automatic Control (CCE), 2014
11th International Conference on, pages 1–5. IEEE.
Guran, M., Fico, T., Chovancova, A., Duchon, F., Hubinsky,
P., and Dubravsky, J. (2014). Localization of irobot
create using inertial measuring unit. In Robotics in
Alpe-Adria-Danube Region (RAAD), 2014 23rd Inter-
national Conference on, pages 1–7. IEEE.
Ibrahim Zunaidi, Norihiko Kato, Y. N. and Matsui, H.
(2006). Positioning system for 4wheel mobile robot:
Encoder, gyro and accelerometer data fusion with er-
ror model method. In CMU Journal, volume 5.
Jensfelt, P. (2001). Approaches to mobile robot localization
in indoor environments. PhD thesis, RoyalInstitute of
Technology, Stockholm, Sweden.
Kam, M., Zhu, X., and Kalata, P. (1997). Sensor fusion
for mobile robot navigation. Proceedings of the IEEE,
85(1):108–119.
Kelley, K. J. (2015). Wi-fi location determination for se-
mantic locations. The Hilltop Review, 7(1):9.
Krishnan, P., Krishnakumar, S., Seshadri, R., and Bala-
subramanian, V. (2014). A robust environment adap-
tive fingerprint based indoor localization system. In
In Proceedings of the 13th International Conference
on Ad Hoc Networks and Wireless (ADHOC-NOW-
2014), volume 8487, pages 360–373.
Li, J. (2012). Characterization of wlan location fingerprint-
ing systems. Master’s thesis, School of Informatics,
University of Edinburgh.
Liu, H., Darabi, H., Banerjee, P., and Liu, J. (2007). Sur-
vey of wireless indoor positioning techniques and
systems. Systems, Man, and Cybernetics, Part C:
Applications and Reviews, IEEE Transactions on,
37(6):1067–1080.
Surmann, H., N
¨
uchter, A., and Hertzberg, J. (2003). An au-
tonomous mobile robot with a 3d laser range finder for
3d exploration and digitalization of indoor environ-
ments. Robotics and Autonomous Systems, 45(3):181–
198.
Widodo Budiharto, D. P. and Jazidie, A. (2011). A robust
obstacle avoidance for service robot using bayesian
approach. International Journal of Advanced Robotic
Systems, 8:52–60.
Xiao, Z., Wen, H., Markham, A., and Trigoni, N. (2014).
Lightweight map matching for indoor localisation us-
ing conditional random fields. In Information Pro-
cessing in Sensor Networks, IPSN-14 Proceedings of
the 13th International Symposium on, pages 131–142.
Zhang, H., Chen, J. C., and Zhang, K. (2014). Rfid-based
localization system for mobile robot with markov
chain monte carlo. In American Society for Engineer-
ing Education (ASEE Zone 1), 2014 Zone 1 Confer-
ence of the, pages 1–6. IEEE.
A Robust Approach for Improving the Accuracy of IMU based Indoor Mobile Robot Localization
445