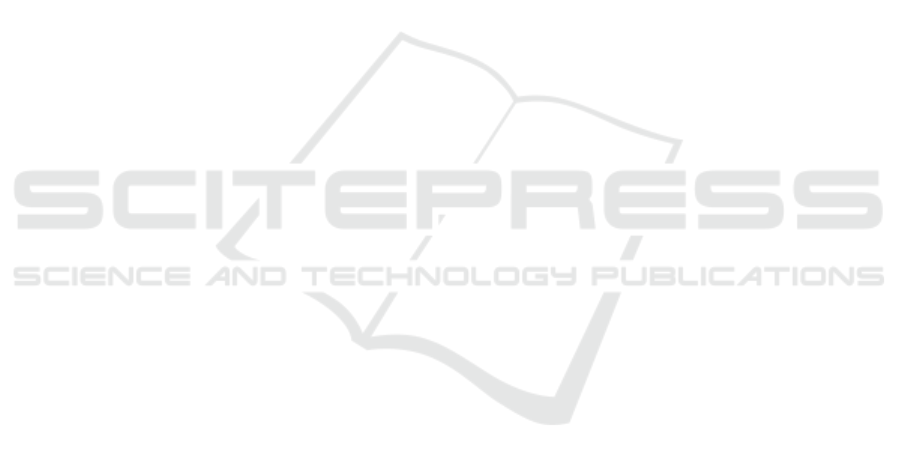
aLite. It also displays the performance results of
the multi-granularity approach using several mecha-
nisms.
Tasks for future work include an optimization of
the experiment management options (include setting
algorithm’s parameters, perform grid search, schedule
experiments), extend the work on visualization mech-
anisms (study other appropriate visualization rep-
resentations for the developed multi-granularity ap-
proach) and create a case study for online evaluation
to validate the assumptions stated in this work.
ACKNOWLEDGEMENTS
This work is financed by the ERDF Fund through
the Operational Programme for Competitiveness and
Internationalization - COMPETE 2020 of Portugal
2020 through the National Innovation Agency (ANI)
as part of the project 3506 and also through project
POCI-01-0145-FEDER-006961 via National Funds
through the FCT – Fundac¸
˜
ao para a Ci
ˆ
encia e a Tec-
nologia as part of project UID/EEA/50014/2013. The
research was also funded from the ECSEL Joint Un-
dertaking, the framework programme for research
and innovation horizon 2020 (2014-2020) under grant
agreement 662189-MANTIS-2014-1.
REFERENCES
Adomavicius, G. and Tuzhilin, A. (2005). Toward the
next generation of recommender systems: A survey
of the state-of-the-art and possible extensions. IEEE
Transactions on Knowledge and Data Engineering,
17(6):734–749.
Bagchi, S. (2015). Performance and Quality Assessment of
Similarity Measures in Collaborative Filtering Using
Mahout. Procedia Computer Science, 50:229–234.
Bobadilla, J., Ortega, F., Hernando, a., and Guti
´
errez, a.
(2013). Recommender systems survey. Knowledge-
Based Systems, 46:109–132.
Domingues, M. A., Leal, J. P., Jorge, A. M., Soares, C., and
Machado, P. (2008). A platform to support web site
adaptation & monitoring of its effects: a case study.
Proceedings of the 6th Workshop on intelligent tech-
niques for web personalization & recommender sys-
tems (ITWP 08), (Mdc):29–36.
Ekstrand, M., Ludwig, M., Konstan, J., and Riedl, J. (2011).
Rethinking the Recommender Research Ecosystem:
Reproducibility, Openness, and LensKit. In ACM
Conference on Recommender Systems, pages 133–
140.
F
´
elix, C., Soares, C., Jorge, A., and Vinagre, J. (2014).
Monitoring Recommender Systems: A Business In-
telligence Approach. Computational Science and Its
Applications–ICCSA 2014, pages 277–288.
Gantner, Z., Rendle, S., Freudenthaler, C., and Schmidt-
Thieme, L. (2011). MyMediaLite: A Free Recom-
mender System Library. In ACM Conference on Rec-
ommender Systems, pages 305–308.
Hahsler, M. (2011). recommenderlab: A Framework
for Developing and Testing Recommendation Algo-
rithms. Nov, pages 1–37.
Herlocker, J. L., Konstan, J. a., Terveen, L. G., and Riedl,
J. T. (2004). Evaluating collaborative filtering recom-
mender systems. ACM Transactions on Information
Systems, 22(1):5–53.
Hu, Y., Koren, Y., and Volinsky, C. (2008). Collaborative
Filtering for Implicit Feedback Datasets. In IEEE In-
ternational Conference on Data Mining, pages 263 –
272.
Koren, Y. (2010). Factor in the Neighbors: Scalable and
Accurate Collaborative Filtering. ACM Transactions
on Knowledge Discovery from Data, 4(1):1–24.
Owen, S., Anil, R., Dunning, T., and Friedman, E. (2011).
Mahout in Action. Manning Publications Co., Green-
wich, CT, USA.
Said, A. and Bellog
´
ın, A. (2014). RiVal – A Toolkit to Fos-
ter Reproducibility in Recommender System Evalua-
tion. ACM Conference on Recommender Systems.
Sarwar, B., Karypis, G., Konstan, J., and Riedl, J. (2001).
Item-Based Collaborative Filtering Recommendation
Algorithms. In International Conference on World
Wide Web, pages 285–295.
Su, X. and Khoshgoftaar, T. M. (2009). A Survey of Col-
laborative Filtering Techniques. Advances in Artificial
Intelligence, 2009:1–19.
Tintarev, N. and Masthoff, J. (2011). Recommender Systems
Handbook, volume 54.
Vanschoren, J., van Rijn, J. N., Bischl, B., and Torgo,
L. (2014). OpenML: networked science in machine
learning. ACM SIGKDD Explorations Newsletter,
15(2):49–60.
Wei, K., Huang, J., and Fu, S. (2007). A survey of e-
commerce recommender systems. In International
Conference on Service Systems and Service Manage-
ment, pages 734–738.
KDIR 2016 - 8th International Conference on Knowledge Discovery and Information Retrieval
140