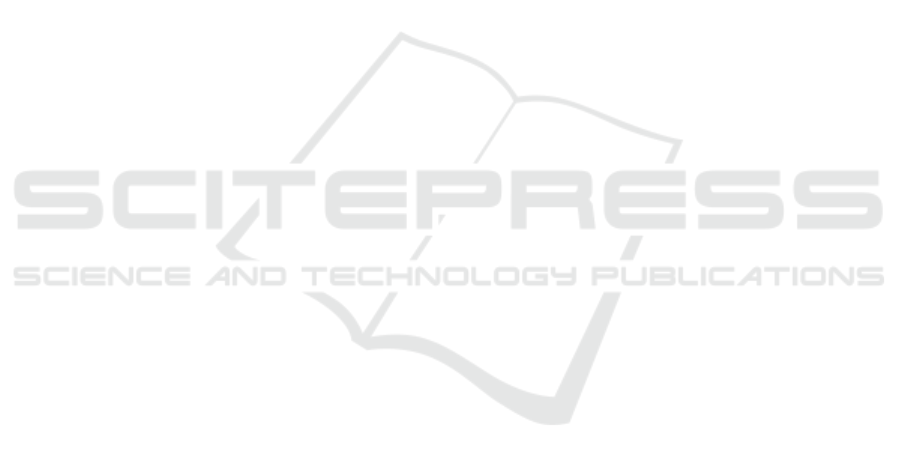
5 CONCLUSION
In this work, we have achieved a first automatic recog-
nition system of Fongbe phonemes starting from the
continuous speech segmentation to the phonetic iden-
tity recognition of the units contained in the speech
signal. We offer a complete recipe algorithms using
fuzzy logic for segmentation, classification, identity
recognition. The output of this complete recipe is a
set of DBN models trained individually on 2307 sen-
tences of the training set and according to the con-
figuration of each subclass. Unlike most phoneme
recognition systems, we first performed a recognition
of plosives, fricatives and nasal consonants for con-
sonant phonemes and recognition of oral and nasal
for vowel phonemes. This yields a better recogni-
tion accuracy, decreasing especially the phone error
rate. In this way working with the subclasses by re-
ducing phoneme set increased approximately with 4%
the recognition accuracy. Another important finding
of this work is that we achieved very good Fongbe
phoneme recognition accuracy with 512 units as hid-
den layer sizes in our DBN models.
REFERENCES
Anapathy, S., Thomas, S., and Hermansky, H. (2009). Mod-
ulation frequency features for phoneme recognition in
noisy speech. J. Acoust. Soc. Am, 125(1):EL8–EL1.
Baghdasaryan, A. G. and Beex, A. A. (2011). Automatic
phoneme recognition with segmental hidden markov
models. In Signals, Systems and Computers (ASILO-
MAR), 2011 Conference Record of the Forty Fifth
Asilomar Conference on, pages 569–574.
chwarz, P., Matejka, P., and Cernocky, J. (2006). Hierarchi-
cal structures of neural networks for phoneme recog-
nition. In 2006 IEEE International Conference on
Acoustics Speech and Signal Processing Proceedings.
Huang, X., Acero, A., and Hon, H.-W. (2001). Spoken
language processing, a guide to theory, algorithm and
system development. Prentice Hall.
Laleye, F. A. A., Ezin, E. C., and Motamed, C. (2015a).
Adaptive decision-level fusion for fongbe phoneme
classification using fuzzy logic and deep belief net-
works. In Proceedings of the 12th International Con-
ference on Informatics in Control, Automation and
Robotics, Volume 1, Colmar, Alsace, France, 21-23
July, pages 15–24.
Laleye, F. A. A., Ezin, E. C., and Motamed, C. (2015b). An
algorithm based on fuzzy logic for text-independent
fongbe speech segmentation. In 11th International
Conference on Signal-Image Technology & Internet-
Based Systems, SITIS 2015, Bangkok, Thailand,
November 23-27, pages 1–6.
Lefebvre, C. and Brousseau., A. (2001). A grammar of
fonge, de gruyter mouton. page 608.
marani, S., Raviram, P., and Wahidabanu, R. (2009). Im-
plementation of hmm and radial basis function for
speech recognition. In Int. Conf. on Intelligent Agent
and Multi-Agent Systems, 2009 (IAMA 2009), Chen-
nai, pages 1–4.
Palaz, D., Collobert, R., and Magimai.-Doss, M. (2013).
End-to-end phoneme sequence recognition using con-
volutional neural networks. Idiap-RR.
Solera-Urena, R., Martin-Iglesias, D., Gallardo-Antolin, A.,
Pelaez-Moreno, C., and Diaz-de Maria, F. (2007). Ro-
bust asr using support vector machines. Speech Com-
munication, 49(4):253–267.
Trentin, E. and Gori, M. (2007). A survey of hybrid
ann/hmm models for automatic speech recognition.
Neurocomputing, 37(1):91–126.
Young, S. (2008). Hmms and related speech recognition
technologies. Springer Handbook of Speech Process-
ing, Springer-Verlag Berlin Heidelberg, pages 539–
557.
Yousafzai, J., Cvetkovic, Z., and Sollich, P. (2009). Tun-
ing support vector machines for robust phoneme clas-
sification with acoustic waveforms. In 10th Annual
conference of the International Speech communica-
tion association, pages 2359 – 2362, England. ISCA-
INST SPEECH COMMUNICATION ASSOC.
Automatic Fongbe Phoneme Recognition From Spoken Speech Signal
109