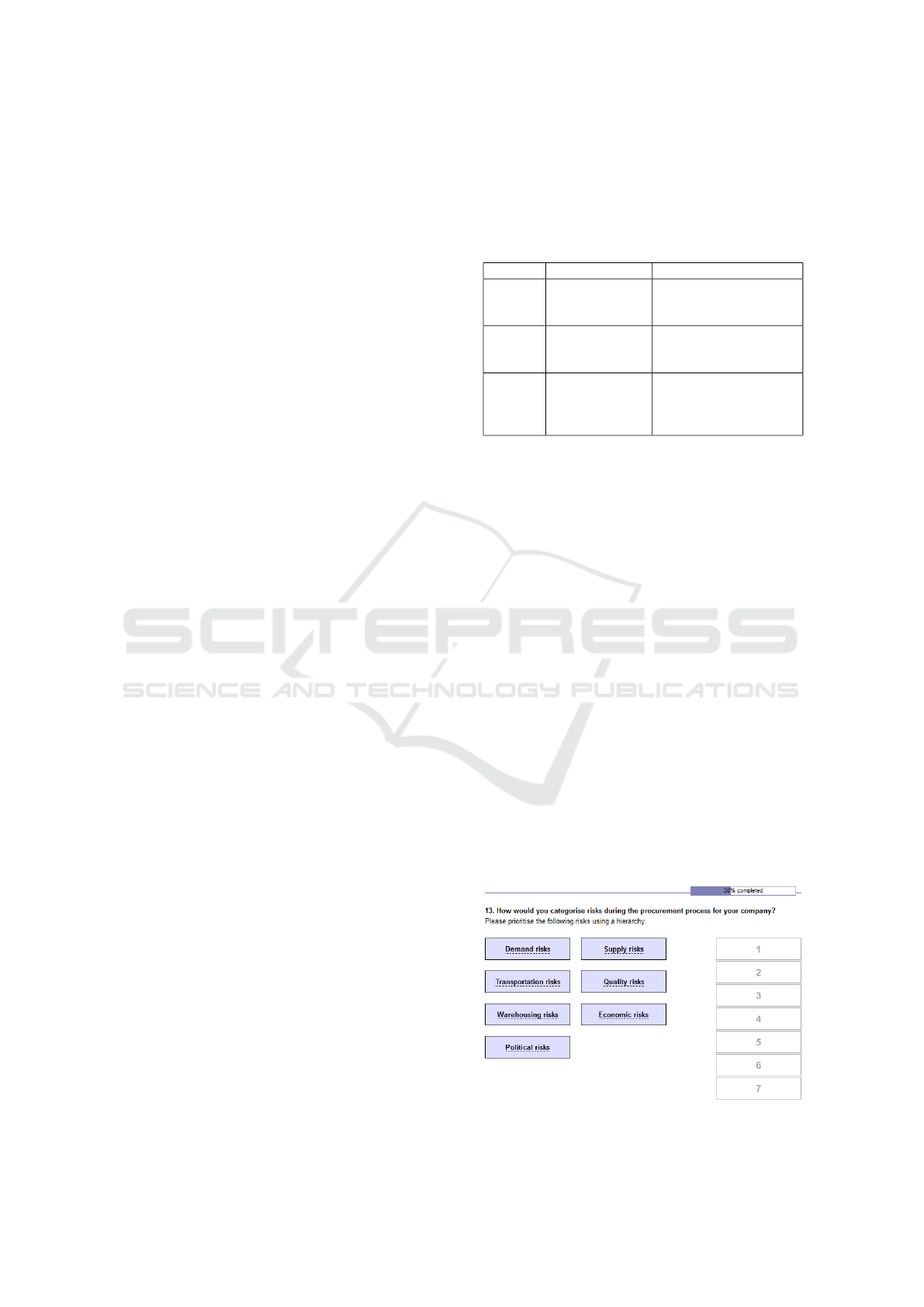
the current practices of SMEs with respect to supply
chain risks:
• What are the risks perceived by companies ?
• How do they rank them in terms of importance,
taking into account both likelihood and impact ?
• How do they manage such risks in terms of people
and tools ?
• What do they require of methods and tools in or-
der to integrate them into their business?
The paper is structured as follows: section 2 pro-
vides an overview regarding risk classification and
risk management methods, Section 3 presents the
survey process, Section 4 provides an overview of
the main characteristics of the participating compa-
nies, Section 5 considers specific procurement as-
pects, Section 6 describes risk perception, Section 7
looks at the current tools and collects the require-
ments necessary for better tool support. Finally sec-
tion 8 concludes with an answer to the identified
SMEs needs.
2 LITERATURE REVIEW
According to ISO 31000, risk is defined as the im-
pact on uncertainty to objectives (ISO, 2009). The ob-
jectives to be assessed are, for instance, strategic, or-
ganisational, and related to projects, products or pro-
cesses. However, Heckmann pointed out that there
is no common definition of SCRM (Heckmann et al.,
2015). This literature review provides an overview
of several classes of risks. In order to reduce com-
plexity, an aggregation of three risk classes was per-
formed, which considers the transportation and ware-
house risks in the context of manufacturing. Quantity
risks are related to how a lack or excess of materi-
als (from raw materials to produces) can affect the
manufacturing process. Quality risks are related to
the good or bad conditioning of materials as well as
the respect of specifications for internal quality. Fi-
nally, Delay risks concern the time aspects, especially
for the supplying of materials, the processing time
and the transport from/to warehouses. Those classes
are shown in Table 1, and they are widely reported
in the literature (Blackhurst et al., 2008; Chopra and
Sodhi, 2004; Mangla et al., 2015; Manuj and Mentzer,
2008; Oke and Gopalakrishnan, 2009; Punniyamoor-
thy et al., 2013; Sodhi and Lee, 2007; Sodhi and Tang,
2012; Thun and Hoenig, 2011).
The quantitative assessment of supply chain risks
is evaluated using the probability of risk and its ex-
pected impact. For instance, Ziegenbein extended
the approach to the number of suppliers and interrup-
tion time. However, this approach is a mathemati-
cal model. There is no connection to the process and
value added chain (Ziegenbein, 2006).
Table 1: Risk classification.
Risk class Definition Root cause
Quantity risks leading to de-
viations in the dis-
posed quantity
insolvency, storage, order cy-
cle, sourcing strategy, sup-
plier, order strategy
Quality risks regarding the
quality of supplied
goods
processing, sourcing strategy,
supplier, logistics
Delay risks causing
unscheduled devia-
tions
processing, logistics, delivery
time, transportation capacity,
number of brokers / transfer
points
3 SURVEY PROCESS
The survey was carried out between October 2014 and
mid-2015. It was based on a trilingual form (French,
German, English) that was distributed to companies in
Wallonia and Germany via different communication
channels, such as dedicated mailing lists and social
networks. The geographical factors were determined
by the collaborative SimQRi project, which involved
different industrial partners and focused on risk as-
sessment (Printz et al., 2014).
The survey was composed of about 40 questions
in total and had different sections: one to understand
the company size and business, one to understand the
importance of the procurement process, another to
identify the current way in which risks are managed,
and finally one to determine the requirements neces-
sary for better tool support. Figure 2 illustrates a typi-
cal question, designed to be simple to understand and
answer. The indicative time needed to answer the sur-
vey is about 15 minutes. The survey was available
through a dedicated website for surveys.
Figure 2: Example of question.
SIMULTECH 2016 - 6th International Conference on Simulation and Modeling Methodologies, Technologies and Applications
328