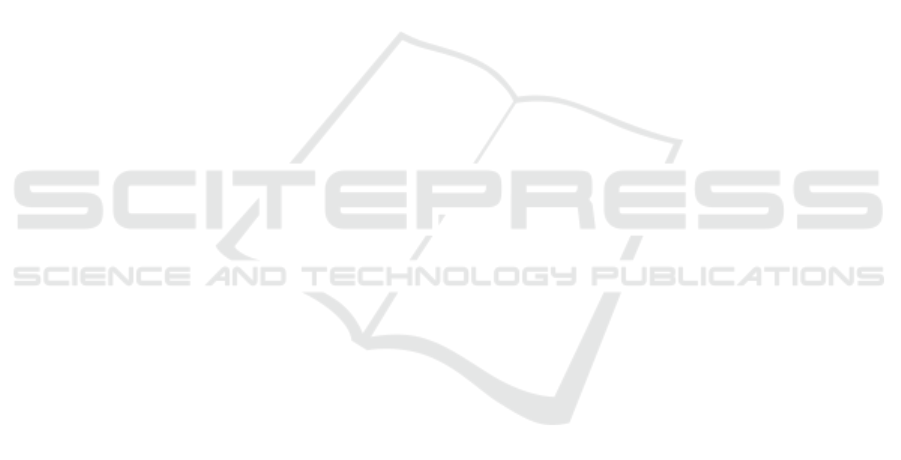
controller remains engaged to a qualitative scale rep-
resenting only realistic rates of change that can be
achieved by each indicator per unit of time. More-
over, the same rate can be used as an alternative scale
in terms of monetary value, since all ratio values can
be translated to production costs and therefore, all es-
timations made by the fuzzy controller can be easily
translated into currency investments.
The Climate Resilience Fuzzy Controller will use
fuzzy logic rules to relate input variables to those
changes that would need to be made over resilience
indicators in order to achieve a particular resilience
level. Controller’s rules are in some how different
from the previous mentioned fuzzy inference rules
since the aim now is to design a controlled perfor-
mance over output variables. Therefore the main
structure of a control rule relates conditions to con-
trol actions, in this sense if conditions are in the form
of linguistic variables representing two process state
variables, a control rule implemented by a fuzzy im-
plication, will lead into an action over control vari-
ables.
As we mentioned before, once the changes over
output variables have been established, they will be
used again as inputs for the aforementioned Climate
Resilience Fuzzy Inference model in order to achieve
final resilience estimations. Working together, the
Fuzzy Inference model and the Resilience Fuzzy Con-
troller can establish a loop-based framework suitable
to perform resilience estimations while maintaining
control actions over indicators performance. It is im-
portant to mention that although each indicator aims
to describe a particular system’s feature or condi-
tion, not all of these features will be controllable
and in any case, the relevance of each indicator to
influence a potential resilience level will be related
with the type of climate impact under scope (whether
droughts, floods, etc.) Therefore the proposed con-
trolling scheme will be based on a careful selection
of which variables/indicators are potentially control-
lable and may influence as well a possible outcome.
For example, in a drought event, the control actions
would be related with indicators depicting food secu-
rity and water resources, while in the case of a climate
impact in the form of floods, the settlement / infras-
tructure sensitivity indicator would be more suitable
to be used as a guide to implement control actions.
Once the Fuzzy Resilience model has been com-
pleted, we intent to implement it over at least two
cities by following a scenario-building approach.
Therefore, in order to define a set of different sce-
narios we will use explicits combinations of values
belonging to constrains variables, along with the po-
tential responses of official bodies in terms of: bud-
get allocation and the political willingness to embrace
and implement adaptation-resilience strategies. Oth-
ers types of variables, not described by the aforemen-
tioned set of indicators but critical to describe partic-
ular social conditions (such as: social development,
risk perceptions, learning capacities, communication
between stakeholders, etc.) can be included in this
stage.
In this way, applying the final resilience controller
would consist in first defining a particular target re-
silience level and then selecting those input param-
eters aimed to describe a particular user’s condition,
either social or political. The target resilience level
would be completely configurable and will be de-
pending on those particular goals requested by the fi-
nal user. Since the time lapse to achieve this level de-
pends entirely on real indicator’s rates of changes, and
a direct cost of the experiment can be automatically
obtained, a final user could re-configure if needed,
whether input parameters or the final resilience level
in order to be consequent with his own resources and
or particular resilience strategic planning.
The whole scheme of the planned Resilience In-
ference Model, working together with the set of Fuzzy
Controllers can be seen in Figure 2.
5 CONCLUSIONS
In this study, we propose a decision making assistance
tool in the form of fuzzy controllers. This tool will es-
timate the amount of time needed to recover a certain
resilience level related to the actions of climate im-
pacts and global change while considering a coerced
social and political landscape. We propose the use of
climate resilience indicators, vastly reported in liter-
ature. By following a resilience framework aimed to
measure both, vulnerability and adaptive capacities,
our intention is to estimate first an initial resilience
level by means of fuzzy composite indices, where in-
dicators aggregation is made assuming a non-linear
interdependence among selected indicators, contrary
to more traditional composite indices methodologies.
This resilience level can then be used as initial input
of a set of fuzzy controllers that implements control
actions over indicators’ performance ratios in order to
achieve a resilience level that can be selected by the fi-
nal user. At the same time that the so called resilience
target level is achieved, an estimation of the required
investment costs to achieve such level is calculated,
considering the rate of production reported for each
indicator.
Resilience fuzzy controller’s configuration op-
tions allows manageable projections considering di-
A Proposal for Climate Change Resilience Management through Fuzzy Controllers
381