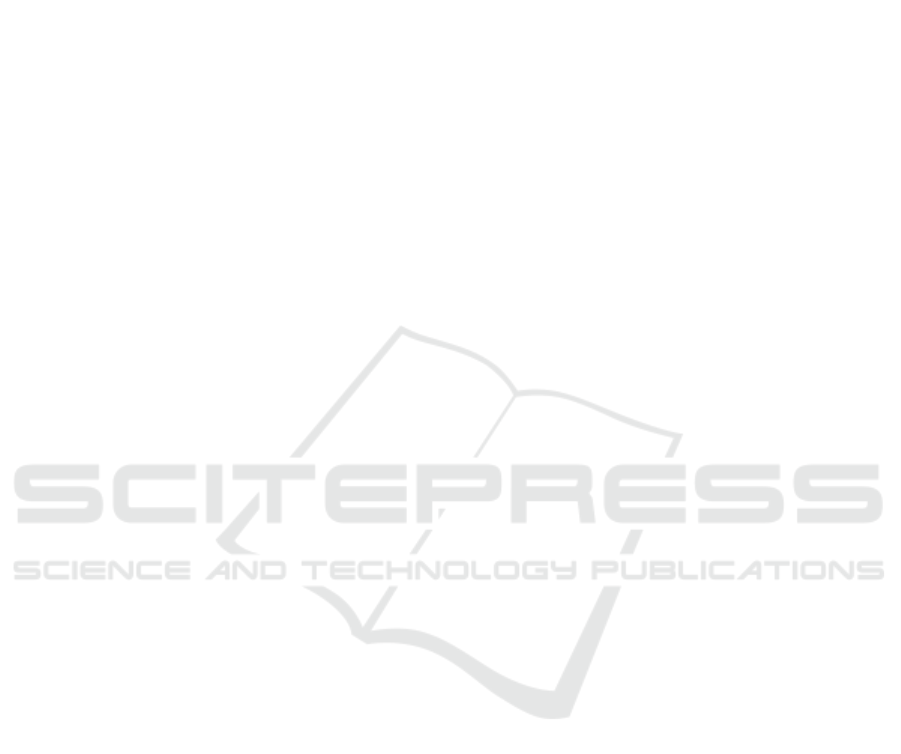
Mobile Data Store Platforms: Test Case based Performance Evaluation
Karim Kussainov and Bolatzhan Kumalakov
School of Science and Technology, Nazarbayev University, Kabanbay batyr 53, Astana, Republic of Kazakhstan
Keywords:
Mobile Database, SQLite, Realm, SnappyDB, Performance Evaluation.
Abstract:
Mobile applications are an important tool in knowledge management, as they collect and process massive
amount of user data. Day-to-day usage of mobile services has rocketed by factors over the last decade. Average
mobile device user installs multiple social network, messaging, professional and leisure applications. Saving
and retrieving associated data becomes a challenging task in the light of the growing number of applications on
a single device. While industry offers several well established platforms, such as BerkeleyDB and UnQLite,
we examine comparatively poorly examined Realm and SnappyDB against industry standard - SQLite. In
particular we are interested in performance and code maintainability, and use a test case in order to asses
them. Results revile that SQLite shows the poorest performance, while Realm provides the most intuitive way
of matching data to the application logic due to its object-oriented nature.
1 INTRODUCTION
The number of mobile services has rocketed by fac-
tors over the last decade. Average handheld device
user installs multiple social network and messaging
applications, apart from work related and, possibly,
gaming software. Storing state variables, user prefer-
ences and other data segments puts pressure on mo-
bile database engines, which have to cope with the
workload in order to support desired user experience.
Industry offers a range of solutions. SQLite is
a widely adopted mobile database management sys-
tem (Kreibich, 2010). It implements relational data
model, and natively supports industry standard - SQL
language specification. All major mobile operat-
ing systems in the market support it, while Android
comes with the pre-installed version by default.
Major players in the market offer at least a dozen
alternative solutions, such as BerkeleyDB and Un-
QLite. Most of them are well tested and positioned
against each other. NoSQL movement, however, also
offers emerging, but promising SnappyDB (Hachicha,
2016) and Realm (Realm, 2016) database engines.
SnappyDB is an open source “key-value” database
management system for Android OS. It is based on
Googles LevelDB storage library, which was initially
designed to overcome relational data model perfor-
mance boundaries by eliminating notions of relation
and referential integrity. It also makes advanced use
of compression algorithms, thus, attempts to optimize
disc space usage as well.
Realm, on the other hand, is a cross-platform,
object-oriented database engine that can be deployed
on Android and iOS devices. It requires storable ob-
jects to extend RealmObject class and define its ac-
cess methods. The data is stored encapsulated, and
access operations are performed in an object-oriented
manner.
This paper presents comparative performance
evaluation of SQLite, SnappyDB and Realm database
engines. Results revile that SQLite shows the poorest
performance, while Realm provides the most intuitive
way of matching data to the application logic due to
its object-oriented nature.
Remainder of the paper is structured as follows:
Section 2 defines research design, including test
cases, evaluation criteria and tools. Section 3 presents
experimental results and corresponding discussion,
while Section 4 concludes the paper.
2 RESEARCH DESIGN
Mobile databases are local storages, which operate on
machines with limited computing and storage capa-
bilities. In order to ensure realistic evaluation results,
test runs are performed on the same mobile device,
one after another. They do not overlap, but run on top
of a realistic set of common user applications, such as
WhatApp, Instagram and Telegram.
Kussainov, K. and Kumalakov, B.
Mobile Data Store Platforms: Test Case based Performance Evaluation.
DOI: 10.5220/0006032300950099
In Proceedings of the 8th International Joint Conference on Knowledge Discovery, Knowledge Engineering and Knowledge Management (IC3K 2016) - Volume 3: KMIS, pages 95-99
ISBN: 978-989-758-203-5
Copyright
c
2016 by SCITEPRESS – Science and Technology Publications, Lda. All rights reserved
95