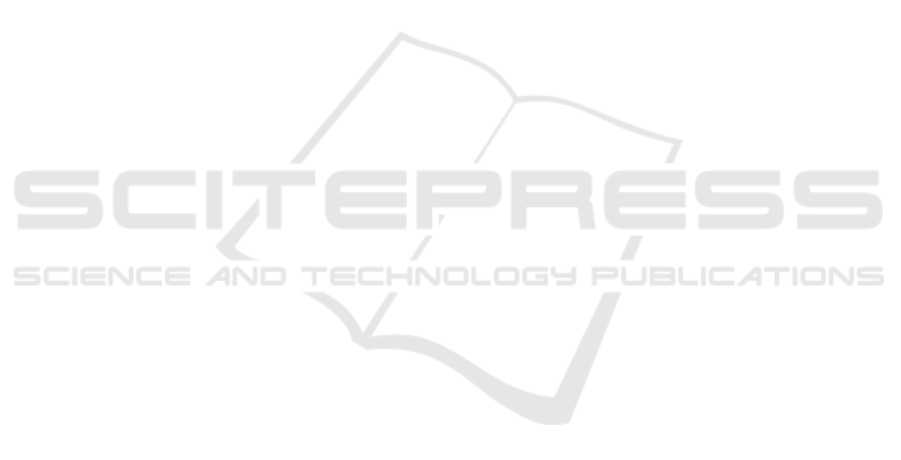
fication method, we estimated easily these evaluations
in the fuzzy sense, considering furthermore the sam-
pling weights in real databases. The signed distance
calculations are made with triangular isosceles fuzzy
numbers.
Moreover, in order to help using our method in
empirical research, we applied these evaluation meth-
ods on a real dataset coming from a survey of the
financial place of Zurich (Switzerland). This empir-
ical part should give confidence that the individual
and global assessments are easily implementable, and
could provide interesting results.
From another side, the non-normality of distribu-
tions is one of the most appealing topics in statisti-
cal inference. Yet, we examined the normality of the
output distributions and we found that the individual
evaluations with the signed distance method tend to
be normally distributed. This result can be profit-
making in terms of adequacy of data in further sta-
tistical analysis. Applying individual evaluation will
enable us to assess easily topics in linguistic question-
naires per observation through fuzzy logic.
Finally, future work certainly encompasses the
understanding of the effect of normality and sym-
metry of such distributions in different statistical
modellings, investigating in particular databases with
missing values.
ACKNOWLEDGEMENT
The authors would like to thank the Office of Econ-
omy of the canton of Zurich (Switzerland) which pro-
vided the permission to use the database.
REFERENCES
BAK (November 2010). Finanzplatz z
¨
urich 2010, monitor-
ing, impact-analyse und ausblick. Technical report,
Amt fur Wirtschaft und Arbeit (AWA) des Kantons
Z
¨
urich.
Berkachy, R. and Donz
´
e, L. (2015). Linguistic question-
naire evaluation: global and individual assessment
with the signed distance defuzzification method. In
Advances in Computational Intelligence, Proceedings
of the 16th International Conference on Fuzzy Systems
FS’15, Rome, Italy, volume 34, pages 13–20.
Berkachy, R. and Donz
´
e, L. (2016a). Linguistic question-
naire evaluation: an application of the signed distance
defuzzification method on different fuzzy numbers.
the impact on the skewness of the output distributions.
International Journal of Fuzzy Systems and Advanced
Applications, 3:12 – 19.
Berkachy, R. and Donz
´
e, L. (2016b). Statistical characteris-
tics of distributions obtained using the signed distance
defuzzification method compared to other methods.
In Proceedings of the International Conference on
Fuzzy Management Methods ICFMSquare, Fribourg,
Switzerland, pages 48–58.
Lin, L. and Lee, H. (2009). Fuzzy assessment method on
sampling survey analysis. Expert Systems with Appli-
cations, 36(3):59555961.
Lin, L. and Lee, H. (2010). Fuzzy assessment for sampling
survey defuzzification by signed distance method. Ex-
pert Systems with Applications, 37(12):78527857.
R Core Team (2015). R: A Language and Environment for
Statistical Computing. R Foundation for Statistical
Computing, Vienna, Austria.
Runkler, T. A. (1997). Selection of appropriate defuzzifi-
cation methods using application specific properties.
IEEE Transactions on Fuzzy Systems.
Wagner, C., Miller, S., and Garibaldi, J. M. (2011). A fuzzy
toolbox for the r programming language. In IEEE In-
ternational Conference on Fuzzy Systems, Taipei, Tai-
wan, pages 1185–1192. IEEE.
Yager, R. R. (1996). Knowledge-based defuzzification.
Fuzzy Sets and Systems, 80(2):177–185.
Yao, J. and Wu, K. (2000). Ranking fuzzy numbers
based on decomposition principle and signed distance.
Fuzzy sets and Systems, 116(2):275–288.
Zadeh, L. (1996). Fuzzy Sets, Fuzzy Logic, and Fuzzy Sys-
tems: Selected Papers of Lotfi A. Zadeh, G. J. Klir and
B. Yuan, Eds., Advances in Fuzzy Systems – Applica-
tions and Theory, volume 6. World Scientific.
Zimmermann, H. (2010). Fuzzy set theory. Wiley Interdisci-
plinary Reviews: Computational Statistics, 2(3):317–
332.
FCTA 2016 - 8th International Conference on Fuzzy Computation Theory and Applications
80