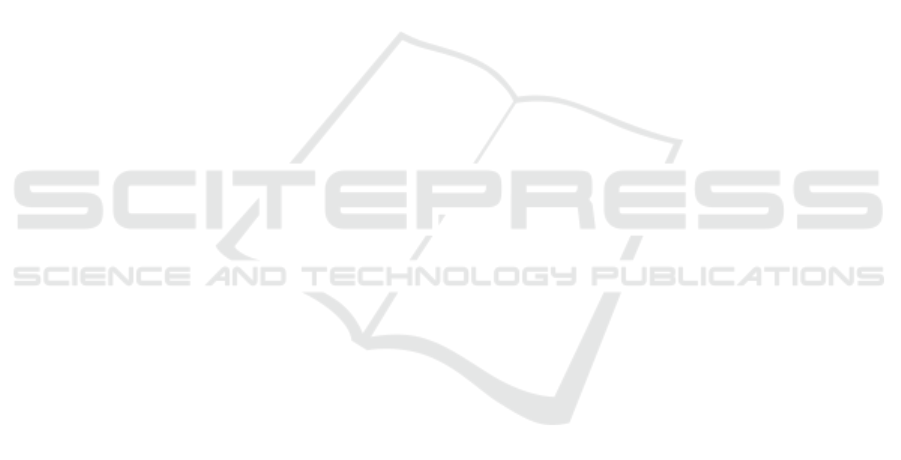
5 CONCLUSION AND FUTURE
WORK
This study used an imaginary scene classification
problem as a testing case to investigate the capability
of heterogeneous ensembles built with the ruls that
consider either accuracy of individual models or di-
versity, or both.Three rules are devised specifically
using accuracy of individual models and the diver-
sity measurements among these models for an en-
semble.The results for HES are much better than the
previous studies (Oliva and Torralba, 2001) that used
individual models for imaginary scene classification
and the state-of-the-art for the homogeneous ensem-
ble, which used all base classifiers used in HES. The
increasing diversity among the models selected for
the ensemble was found to be advantageous, lead-
ing to more stable and reliable results. Our research
found that increasing the number of models also af-
fects the ensembles results. This indicated that diver-
sity is more effective when used with a higher number
of models selected for the ensemble. It can therefore
be concluded that combining models results in high
accuracy and diversity for an ensemble has consider-
able advantages in terms of the ensemble’s accuracy.
Various questions for future work emerge from
this paper. First, this research covered only the anno-
tations part of the dataset. It could be useful to involve
the images part directly. Second, only three rules were
used in this experiment; future work should consider
more rules with different measures for ensemble se-
lecting models. Third, more experiments will be con-
ducted by using more datasets.
REFERENCES
Bosch, A., Zisserman, A., and Mu
˜
noz, X. (2006). Scene
classification via plsa. In Computer Vision–ECCV
2006, pages 517–530. Springer.
Brown, G., Wyatt, J., Harris, R., and Yao, X. (2005). Di-
versity creation methods: a survey and categorisation.
Information Fusion, 6(1):5–20.
Caruana, R., Niculescu-Mizil, A., Crew, G., and Ksikes, A.
(2004). Ensemble selection from libraries of models.
In Proceedings of the twenty-first international con-
ference on Machine learning, page 18. ACM.
Dietterich, T. G. (2000). Ensemble methods in machine
learning, pages 1–15. Springer.
Giacinto, G. and Roli, F. (2001). Design of effective neural
network ensembles for image classification purposes.
Image and Vision Computing, 19(9):699–707.
Grauman, K. and Darrell, T. (2005). The pyramid match
kernel: Discriminative classification with sets of im-
age features. In Computer Vision, 2005. ICCV 2005.
Tenth IEEE International Conference on, volume 2,
pages 1458–1465. IEEE.
Hofmann, T. (2001). Unsupervised learning by probabilis-
tic latent semantic analysis. Machine learning, 42(1-
2):177–196.
Hotta, K. (2008). Scene classification based on multi-
resolution orientation histogram of gabor features. In
Computer Vision Systems, pages 291–301. Springer.
Ke, Y. and Sukthankar, R. (2004). Pca-sift: A more
distinctive representation for local image descriptors.
In Computer Vision and Pattern Recognition, 2004.
CVPR 2004. Proceedings of the 2004 IEEE Computer
Society Conference on, volume 2, pages II–506. IEEE.
Lazebnik, S., Schmid, C., and Ponce, J. (2006). Beyond
bags of features: Spatial pyramid matching for rec-
ognizing natural scene categories. In Computer Vi-
sion and Pattern Recognition, 2006 IEEE Computer
Society Conference on, volume 2, pages 2169–2178.
IEEE.
Lertampaiporn, S., Thammarongtham, C., Nukoolkit, C.,
Kaewkamnerdpong, B., and Ruengjitchatchawalya,
M. (2013). Heterogeneous ensemble approach with
discriminative features and modified-smotebagging
for pre-mirna classification. Nucleic acids research,
41(1):e21–e21.
Liu, Y., Yao, X., and Higuchi, T. (2000). Evolutionary en-
sembles with negative correlation learning. Evolution-
ary Computation, IEEE Transactions on, 4(4):380–
387.
Lowe, D. G. (2004). Distinctive image features from scale-
invariant keypoints. International journal of computer
vision, 60(2):91–110.
Mikolajczyk, K. and Schmid, C. (2004). Scale & affine in-
variant interest point detectors. International journal
of computer vision, 60(1):63–86.
Oliva, A. and Torralba, A. (2001). Modeling the shape
of the scene: A holistic representation of the spatial
envelope. International journal of computer vision,
42(3):145–175.
Partridge, D. and Krzanowski, W. (1997). Software di-
versity: practical statistics for its measurement and
exploitation. Information and software technology,
39(10):707–717.
Siagian, C. and Itti, L. (2007). Rapid biologically-inspired
scene classification using features shared with visual
attention. Pattern Analysis and Machine Intelligence,
IEEE Transactions on, 29(2):300–312.
Wallraven, C., Caputo, B., and Graf, A. (2003). Recognition
with local features: the kernel recipe. In Computer
Vision, 2003. Proceedings. Ninth IEEE International
Conference on, pages 257–264. IEEE.
Wang, H., Fan, W., Yu, P. S., and Han, J. (2003). Mining
concept-drifting data streams using ensemble classi-
fiers. In Proceedings of the ninth ACM SIGKDD in-
ternational conference on Knowledge discovery and
data mining, pages 226–235. ACM.
Wang, W. (2008). Some fundamental issues in ensem-
ble methods. In Neural Networks, 2008. IJCNN
2008.(IEEE World Congress on Computational In-
Heterogeneous Ensemble for Imaginary Scene Classification
203