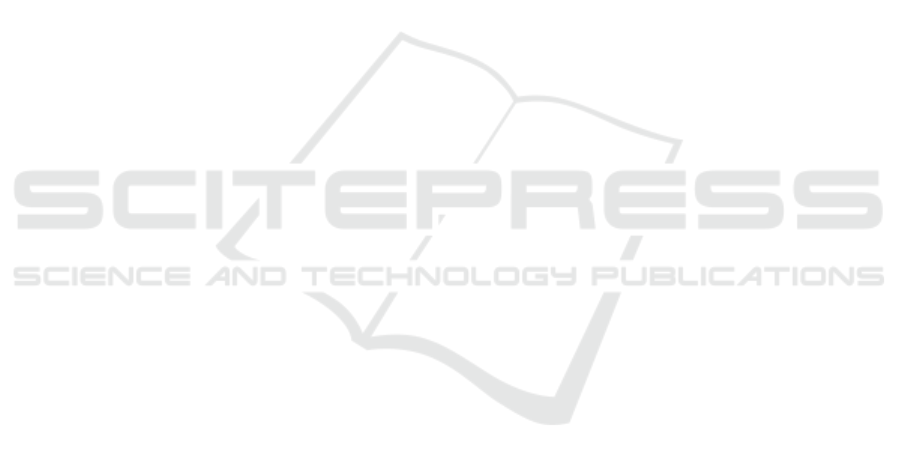
http://neurofeedback.visaduma.info/EmotivResearch.p
df.
Emotiv Systems Inc. viewed 2 May 2016
http://www.emotiv.com/
EN 12464-1, (2011). Light and lighting. Lighting of work
places. Part 1. Indoor work places. European Lighting
Standard.
Fakhruzzaman, M. N., Riksakomara, E., Suryotrisongko,
H. (2015). EEG Wave Identification in Human Brain
with Emotiv EPOC for Motor Imagery. Procedia
Computer Science. 72, 269-276.
Figueiro, M. G., Rea, M. S., (2010). The effects of red and
blue light on circadian variations in cortisol, alpha
amylase and melatonin. International Journal of
Endocrinology. 2010 (Article ID 829351),
doi:10.1155/2010/829351.
Giusti, A., Zocchi, C., Rovetta, A., 2009. A Noninvasive
System for Evaluating Driver Vigilance Level
Examining Both Physiological and Mechanical Data.
IEEE Trans. on Intelligent Transportation Systems.
10(1), 127-134.
Gola, M., Magnusk, M., Szumska, I., Wróbel, A. (2013).
EEG beta band activity is related to attention and
attentional deficits in the visual performance of elderly
subjects. International Journal of Psychophysiology,
89(3), 334-341.
Górnicka, G. B. (2008). Lighting at work: environmental
study of direct effects of lighting level and spectrum
on psychophysiological variables. PhD Thesis,
Technische Universiteit Eindhoven.
Hanifin J. P, Stewart K. T., Smith P., Tanner R., Rollag
M., Brainard, G. C., (2006). High-intensity red light
suppresses melatonin. Chronobiol Int. 23(1-2), 251-
68.
Horne, J. A. and Östberg, O. A. (1976). A self-assessment
questionnaire to determine morningness-eveningness
in human circadian rhythms. International Journal of
Chronobiology. 4, 97-110.
Ji, H., Li, J., Cao, L., Wang, D. (2012, Marz). A EEG-
Based Brain Computer Interface System towards
Applicable Vigilance Monitoring. In: Foundations of
Intelligent Systems. Chapter In: Advances in
Intelligent and Soft Computing. 122, 743-749.
Klimesch, W. (2012). Alpha-band oscillations, attention,
and controlled access to stored information. Trends in
cognitive sciences. 16(12), 606-617.
Lal, S. K. I. and Craig, A. (2001). A critical review of the
psychophysiology of driver fatigue. Biological
Psychology. 55(3), 173-194.
Li, J., Yan, C. G., Xia, B., Cao, L., Wang, D. M., Sun, Y.
R. (2010). EEG-based Brain Computer Interface for
Vigilance Analysis and Estimate. In: Proceedings of
Conference on Asia-Pacific Signal and Information
Processing Association, APSIPA ASC, Singapore, 14-
17 Dec. 2010, 189-192.
Okamoto, Y., Rea, M. S., Figueiro, M. G. (2014).
Temporal dynamics of EEG activity during short- and
long-wavelength light exposures in the early morning.
BMC Res Notes, 2014; 7:113.
Pham, T. D. and Tran, D. (2012). Emotion Recognition
Using the Emotiv EPOC Device. In: LNCS 7667, 394-
399, Springer-Verlag Berlin Heidelberg.
Rahman, S. A., Flynn-Evans, E. E., Aeschbach, D.,
Brainard, G. C., Czeisler, C. A., Lockley, S. W.
(2014). Diurnal spectral sensitivity of the acute
alerting effects of light. Sleep. 37(2), 271-281.
Ramirez, R. and Vamvakousis, Z. (2012). Detecting
Emotion from EEG Signals Using the Emotive Epoc
Device. In: LNCS 7670, 175-184. Springer-Verlag
Berlin Heidelberg.
Sahin, L. and Figueiro, M.G. (2013). Alerting effects of
short-wavelengths (blue) and long – wavelengths (red)
lights in the afternoon. Physiology & Behaviour. 116-
117(5), 1-7.
Sahin, L., Wood, B.M., Plitnick, B., Figueiro, M.G.
(2014). Daytime light exposure: effects on biomarkers,
measure of alertness and performance. Behavioural
Brain Research. 274(1 November), 176-185.
Stytsenko, K., Jablonskis, E., & Prahm, C. (2011, August).
Evaluation of consumer EEG device Emotiv EPOC.
In: Proceedings of MEi: CogSci Conference 2011.
Thapan, K., Arendt, J., Skene, D. (2001). An action
spectrum for melatonin suppression: Evidence for a
novel non-rod. non-cone photoreceptor system in
humans. J. Physiol. 535, 261-267.
Viola, A.U., James, L.M., Schlangen, L.J., Dijk, D.J.
(2008). Blue-enriched white light in the workplace
improves self-reported alertness, performance and
sleep quality. Scand J Work Environ Health. 34(4),
297-306.
Wolska, A. and Sawicki, D. (2015). Melanopic lux and
blue light under different lighting scenarios. In:
Proceedings of the 28th Session of the CIE, June 28 -
July 4, 2015, Manchester UK. Vol.1, Part 2, 1238-
1243.
Zhan, Z. and Mu, Z. (2013). Vigilance Degree Computing
based on EEG. Telkomnika. 11(9), 5409-5414.
NEUROTECHNIX 2016 - 4th International Congress on Neurotechnology, Electronics and Informatics
42