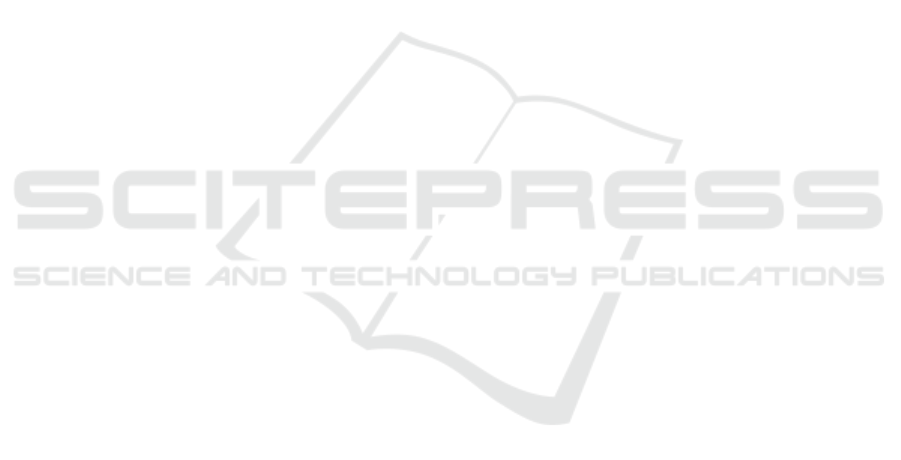
Ramachandran, A. and Feamster, N. (2006). Understanding
the network-level behavior of spammers. In ACM SIG-
COMM Computer Communication Review, volume 36,
pages 291–302.
Rao, J. M. and Reiley, D. H. (2012). The Economics of Spam.
Journal of Economic Perspectives, 26(3):87–110.
Rojas, R. (1996). Kohonen Networks. In Neural Networks:
A Systematic Introduction, pages 391–412. Springer-
Verlag, Berlin.
Sahami, M., Dumais, S., Heckerman, D., and Horvitz, E.
(1998). A Bayesian Approach to Filtering Junk E-
mail. AAAI Technical Report WS-98-05, Madison,
Wisconsin.
Santos, I., Sanz, B., Laorden, C., Brezo, F., and Bringas, P. G.
(2011). Computational Intelligence in Security for In-
formation Systems: 4th International Conference, CI-
SIS 2011, Held at IWANN 2011. Torremolinos-Málaga,
Spain.
Sculley, D., Wachman, G., and Brodley, C. E. (2006). Spam
Filtering Using Inexact String Matching in Explicit
Feature Space with On-Line Linear Classifiers. In
TREC.
Shunli, Z. and Qingshuang, Y. (2010). Personal Spam Filter
by Semi-supervised Learning. In Proceedings of the
Third International Symposium on Com Puter Science
and Computational Technology (ISCSCT ’10), pages
171–174, Jiaozuo, P. R. China.
Skillicorn, D. (2013). Other versions of the Enron data
(preprocessed).
Slaby, A. (2007). ROC Analysis with Matlab. In Infor-
mation Technology Interfaces, 2007. ITI 2007. 29th
International Conference on, pages 191–196. IEEE.
Spammer-X, Posluns, J., and Sjouwerman, S. (2004). Inside
the SPAM Cartel. Syngress - Elsevier, 1 edition.
Sprengers, M. and Heskes, T. T. (2009). The Effects of Dif-
ferent Bayesian Poison Methods on the Quality of the
Bayesian Spam Filter ‘SpamBayes’. Bachelor thesis,
Radboud University Nijmegen.
Statista (2016). Global spam volume as percentage of total
e-mail traffic from 2007 to 2014.
Styler, W. (2011). The EnronSent Corpus. Technical Report
01-2011, University of Colorado at Boulder Institute
of Cognitive Science.
Suárez Araujo, C. P., García Báez, P., and Hernández Tru-
jillo, Y. (2010). Neural Computation Methods in the
Determination of Fungicides. In Fungicides. INTECH
Open Access Publisher, odile carisse edition.
Subramaniam, T., Jalab, H. A., and Taqa, A. Y.
(2010). Overview of textual anti-spam filtering tech-
niques. International Journal of the Physical Science,
5(12):1869–1882.
Tan, H. S. and George, S. E. (2004). Investigating Learning
Parameters in a Standard 2-D SOM Model to Select
Good Maps and Avoid Poor Ones. In Australasian
Joint Conference on Artificial Intelligence, pages 425–
437. Springer.
The Apache SpamAssassin Project (2013). Index of the
SpamAssassin’s Public Corpus.
The Apache SpamAssassin Project (2014). SpamAssassin
v3.3.x: Tests Performed to Determine Spaminess and
Haminess of a Message.
Uemura, T., Ikeda, D., and Arimura, H. (2008). Unsuper-
vised spam detection by document complexity estima-
tion. In Discovery Science, pages 319–331.
Vesanto, J., Himberg, J., Alhoniemi, E., and Parhankangas, J.
(2000). SOM Toolbox for Matlab 5. Technical Report
Report A57, Helsinki University of Technology.
Vrusias, B. L. and Golledge, I. (2009a). Adaptable Text
Filters and Unsupervised Neural Classifiers for Spam
Detection. In Proceedings of the International Work-
shop on Computational Intelligence in Security for
Information Systems CISIS’08, volume 53 of Advances
in Soft Computing, pages 195–202. Springer Berlin
Heidelberg.
Vrusias, B. L. and Golledge, I. (2009b). Online Self-
Organised Map Classifiers as Text Filters for Spam
Email Detection. Journal of Information Assurance
and Security (JIAS), 4(2):151–160.
Wang, D., Irani, D., and Pu, C. (2013). A Study on Evolu-
tion of Email Spam Over Fifteen Years. pages 1–10,
Atlanta, Georgia (USA). IEEE.
Wang, D. and Zhang, H. (2013). Inverse-Category-
Frequency Based Supervised Term Weighting Schemes
for Text Categorization. Journal of Information Sci-
ence & Engineering, 29(2):209–225.
Wittel, G. L. and Wu, S. F. (2004). On Attacking Statistical
Spam Filters. In CEAS.
Xie, C., Ding, L., and Du, X. (2009). Anti-spam Filters
Based on Support Vector Machines. In Advances in
Computation and Intelligence. 4th International Sym-
posium, ISICA 2009, volume 5821 of Lecture Notes
in Computer Science, pages 349–357. Springer Berlin
Heidelberg, Huangshi, China.
Xu, J.-M., Fumera, G., Roli, F., and Zhou, Z.-H. (2009).
Training SpamAssassin with Active Semi-supervised
Learning. In Proceedings of the 6th Conference on
Email and Anti-Spam (CEAS’09), pages 1–8. Citeseer.
Yerazunis, W., Kato, M., Kori,, M., Shibata, H., and Hacken-
berg, K. (2010). Keeping the Good Stuff In: Confiden-
tial Information Firewalling with the CRM114 Spam
Filter & Text Classifier. White Paper for Black Hat
USA, pages 1–18.
Zeimpekis, D., Kontopoulou, E. M., and Gallopoulos, E.
(2011). Text to Matrix Generator (TMG).
Zhang, Y. (2012). Lecture for Chapter 2 - Data Preprocess-
ing.
Zhou, D., Bousquet, O., Lal, T. N., Weston, J., and
Schölkopf, B. (2004). Learning with local and global
consistency. Advances in neural information process-
ing systems, 16:321–328.
Zhou, D., Burges, C. J. C., and Tao, T. (2007). Transductive
link spam detection. In Proceedings of the 3rd Interna-
tional Workshop on Adversarial Information Retrieval
on the Web, pages 21–28.
Žliobait
˙
e, I., Pechenizky, M., and Gama, J. (2015). An
overview of concept drift applications. In Japkowicz,
N. and Stefanowski, J., editors, Big Data Analysis:
New Algorithms for a New Society, volume 16 of Stud-
ies in Big Data, pages 91–114. Springer International
Publishing.
NCTA 2016 - 8th International Conference on Neural Computation Theory and Applications
32