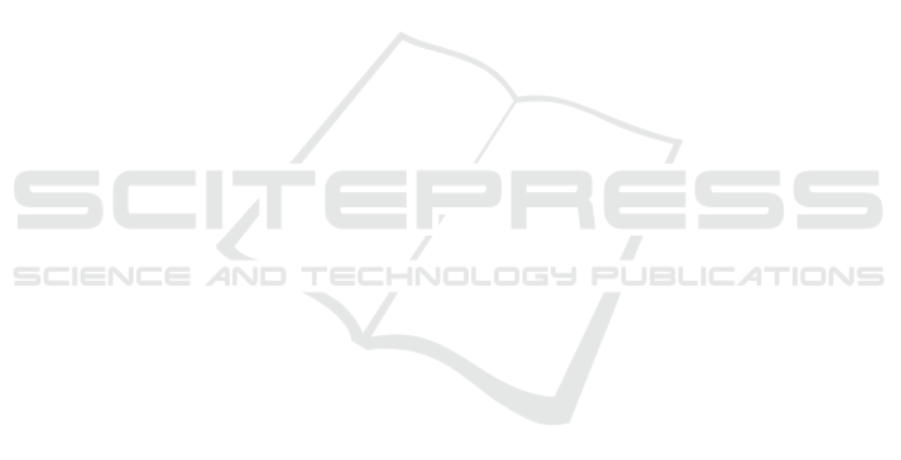
5 CONCLUSION AND FUTURE
WORK
Compared to laboratory studies, the presented traipor
concept yields comparable results with similar fitting
accuracy using the Fitness-Fatigue model.
Since this model is based on a convolution with
an exponential function, a straight line as it results by
replacing all measurements within one period (e.g.,
month) by the maximum value can generally not be
approximated. Changing this concept should there-
fore be considered. Other approaches using differ-
ent filters should be analyzed. Using a moving maxi-
mum function might also reduce leaps between differ-
ent performance measurements. This way, unrealistic
performance values near to a training break might be
avoided or reduced at least.
Predicting future performance based on a given
training plan is an interesting application of train-
ing models, e.g., to generate training plans to reach
a certain goal. Using the described method, it is
possible to predict training effects for the upcoming
month with similar accuracy as achieved in fitting
(RMSE = 16.56). Even predicting six month into
the future yields acceptable results (RMSE = 20.62)
in all 11 subjects. Since in prediction preload plays
an important role (i.e., accumulated strain at T = 0)
special treatment of initial performance p
∗
was nec-
essary. Further research will be required to examine
the influence of preload as it should generally be con-
sidered in model identification.
Analysis of further performance metrics, espe-
cially for submaximal performances as these are more
common in non-athletes, would be promising by en-
abling the utilization of training models in mass sports
and training devices. To verify accuracy results, fur-
ther experiments with more subjects, even less ambi-
tious cyclists and additional laboratory control exper-
iments have to be conducted.
ACKNOWLEDGEMENT
This work was supported by a funding of the state
North Rhine-Westphalia, Germany.
REFERENCES
Balmer, J., Davison, R. R., and Bird, S. R. (2000). Peak
power predicts performance power during an outdoor
16.1-km cycling time trial. Medicine and Science in
Sports and exercise, 32(8):1485–1490.
Busso, T., Candau, R., and Lacour, J.-R. (1994). Fatigue
and fitness modelled from the effects of training on
performance. European journal of applied physiology
and occupational physiology, 69(1):50–54.
Busso, T., Carasso, C., and Lacour, J.-R. (1991). Adequacy
of a systems structure in the modeling of training ef-
fects on performance. Journal of Applied Physiology,
71(5):2044–2049.
Busso, T., Denis, C., Bonnefoy, R., Geyssant, A., and La-
cour, J.-R. (1997). Modeling of adaptations to phys-
ical training by using a recursive least squares algo-
rithm. Journal of applied physiology, 82(5):1685–
1693.
Calvert, T. W., Banister, E. W., Savage, M. V., and Bach, T.
(1976). A systems model of the effects of training on
physical performance. IEEE Transactions on Systems,
Man and Cybernetics, (2):94–102.
Hellard, P., Avalos, M., Lacoste, L., Barale, F., Chatard, J.-
C., and Millet, G. P. (2006). Assessing the limitations
of the banister model in monitoring training. Journal
of sports sciences, 24(05):509–520.
Krebs, P. and Duncan, D. T. (2015). Health app use among
us mobile phone owners: A national survey. JMIR
mHealth and uHealth, 3(4).
Lee, J.-M., Kim, Y., and Welk, G. J. (2014). Validity of
consumer-based physical activity monitors. Med Sci
Sports Exerc, 46(9):1840–8.
Mujika, I., Busso, T., Lacoste, L., Barale, F., Geyssant, A.,
and Chatard, J.-C. (1996). Modeled responses to train-
ing and taper in competitive swimmers. Medicine and
science in sports and exercise, 28(2):251–258.
Perl, J. (2000). Antagonistic adaptation systems: An exam-
ple of how to improve understanding and simulating
complex system behaviour by use of meta-models and
on line-simulation. 16th IMACS Congress.
Pfeiffer, M. (2008). Modeling the relationship between
training and performance-a comparison of two antag-
onistic concepts. International journal of computer
science in sport, 7(2):13–32.
Schaefer, D., Asteroth, A., and Ludwig, M. (2015). Train-
ing plan evolution based on training models. In
2015 International Symposium on Innovation in In-
telligent SysTems and Applications (INISTA) Proceed-
ings, pages 141–148.
Shrout, P. E. and Fleiss, J. L. (1979). Intraclass correla-
tions: uses in assessing rater reliability. Psychological
bulletin, 86(2):420.
Tan, F. H. and Aziz, A. R. (2005). Reproducibility of out-
door flat and uphill cycling time trials and their per-
formance correlates with peak power output in moder-
ately trained cyclists. J Sports Sci Med, 4(3):278–284.
Yang, R., Shin, E., Newman, M. W., and Ackerman, M. S.
(2015). When fitness trackers don’t ’fit’: End-user dif-
ficulties in the assessment of personal tracking device
accuracy. In Proceedings of the 2015 ACM Interna-
tional Joint Conference on Pervasive and Ubiquitous
Computing, UbiComp ’15, pages 623–634, New York,
NY, USA. ACM.
icSPORTS 2016 - 4th International Congress on Sport Sciences Research and Technology Support
82