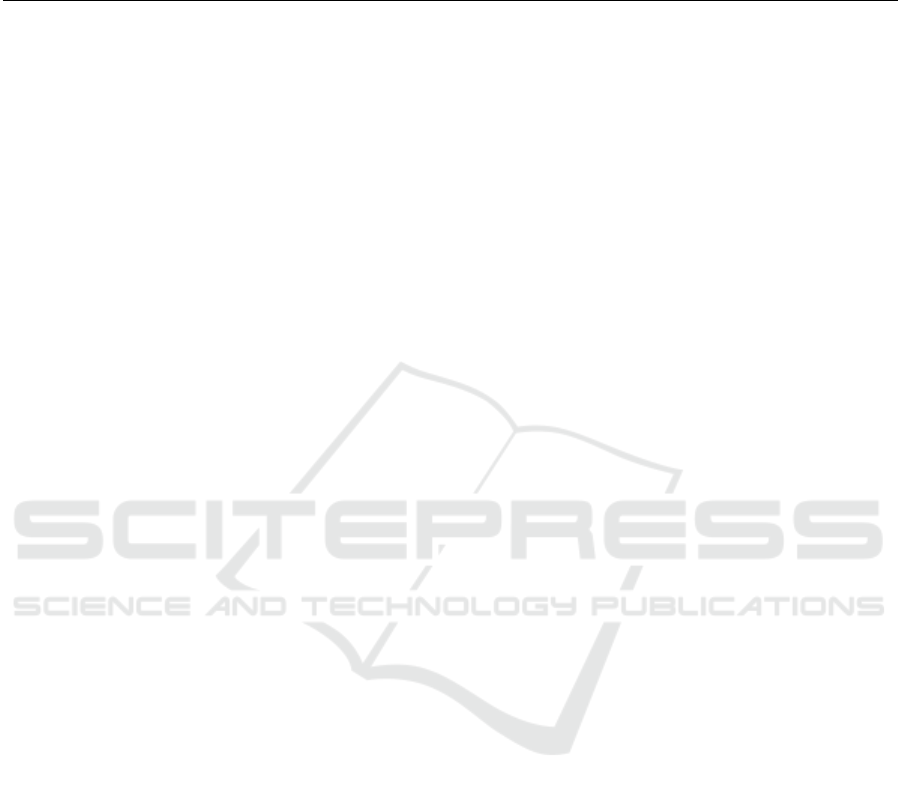
Table 1: Data refinement results with real forestry machine operation data.
Stems excluded
Mach Feed speed Pos. error (felling diam Stems in Long spruces Long pines
ID Stems Logs outlier (logs) outlier (logs) <15 cm) context (in context) (in context)
1 11,000 27,000 4.0% 0.33% 54% 1,400 40% 52%
2 6,300 19,000 1.8% 1.1% 23% 1,200 60% 26%
3 14,000 39,000 4.1% 0.93% 36% 2,500 61% 22%
4 6,600 18,000 3.9% 0.56% 48% 1,100 61% 5.6%
5 5,900 18,000 2.9% 0.27% 31% 1,000 60% 8.7%
6 7,800 26,000 5.1% 0.36% 30% 1,100 75% 9.1%
7 8,000 27,000 1.6% 0.39% 26% 1,400 72% 7.9%
8 10,000 28,000 4.9% 0.76% 32% 2,000 33% 33%
9 12,000 38,000 4.9% 1.4% 34% 1,600 64% 20%
10 6,800 25,000 9.7% 0.93% 18% 1,100 55% 4.2%
11 6,500 20,000 4.9% 1.0% 29% 1,400 62% 13%
cific XML format for some configuration items. Also,
derived variables can only be Boolean values – nu-
meric values are not currently supported though they
would offer significantly more potential for various
uses cases.
7 CONCLUSION
This paper introduces a software concept for centrally
manageable data refinement run locally in the ma-
chines of an arbitrarily large fleet. As machine data is
utilised locally in end-user applications (such as feed-
back generation to improve machine operation and
productivity), it is beneficial if the required data pre-
processing is configurable and managed on the fleet
level. Configurability covers multiple actions: outlier
checks detect erroneous sensor output, derived vari-
ables can be calculated from original data, and data
sets are categorised according to predefined condi-
tions. Further, determining the operating context is
also configurable. Context awareness is utilised as the
context may affect how data should be interpreted.
A functional prototype has been implemented.
Utilising externally defined configurations, it pro-
cesses operational data retrieved from an interface
similar to a physical production machine. The solu-
tion showed its potential as a part of an added-value
data refinement concept by enabling centralised man-
agement.
As the current prototype does not cover all con-
cept aspects, a few future tasks remain. A concrete
solution for the delivery of configuration data from
office to machines should be designed. Also, the cur-
rent context recognition method appeared to be too
simple.
REFERENCES
Banerjee, T. P. and Das, S. (2012). Multi-sensor data fusion
using support vector machine for motor fault detec-
tion. Information Sciences, 217:96 – 107.
Basir, O. and Yuan, X. (2007). Engine fault diagnosis based
on multi-sensor information fusion using dempster-
shafer evidence theory. Information Fusion, 8(4):379
– 386.
Choudhury, T., Consolvo, S., Harrison, B., Hightower, J.,
Lamarca, A., Legrand, L., Rahimi, A., Rea, A., Bor-
dello, G., Hemingway, B., Klasnja, P., Koscher, K.,
Landay, J., Lester, J., Wyatt, D., and Haehnel, D.
(2008). The mobile sensing platform: An embed-
ded activity recognition system. Pervasive Comput-
ing, IEEE, 7(2):32–41.
Duan, L. and Xu, L. D. (2012). Business intelligence for
enterprise systems: A survey. Industrial Informatics,
IEEE Transactions on, 8(3):679–687.
Favela, J., Tentori, M., Castro, L. A., Gonzalez, V. M.,
Moran, E. B., and Mart
´
ınez-Garc
´
ıa, A. I. (2007). Ac-
tivity recognition for context-aware hospital applica-
tions: Issues and opportunities for the deployment of
pervasive networks. Mob. Netw. Appl., 12(2-3):155–
171.
Fountas, S., Sorensen, C., Tsiropoulos, Z., Cavalaris, C.,
Liakos, V., and Gemtos, T. (2015). Farm machin-
ery management information system. Computers and
Electronics in Agriculture, 110:131–138.
Golparvar-Fard, M., Heydarian, A., and Niebles, J. C.
(2013). Vision-based action recognition of earthmov-
ing equipment using spatio-temporal features and sup-
port vector machine classifiers. Advanced Engineer-
ing Informatics, 27(4):652 – 663.
Hodge, V. and Austin, J. (2004). A survey of outlier de-
tection methodologies. Artificial Intelligence Review,
22(2):85–126.
Hou, L. and Bergmann, N. (2012). Novel industrial wire-
less sensor networks for machine condition monitor-
ing and fault diagnosis. Instrumentation and Measure-
ment, IEEE Transactions on, 61(10):2787–2798.
Enabling Centralised Management of Local Sensor Data Refinement in Machine Fleets
29