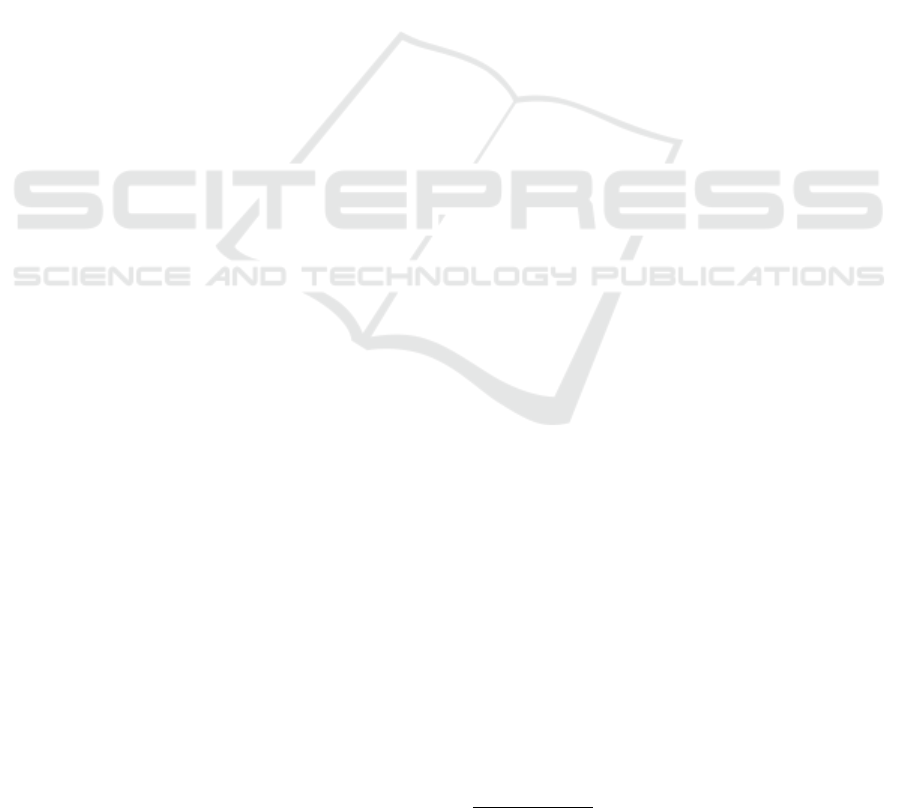
selected for the sentiment analysis results presented in
this paper and are listed here along with the words and
word stems (represented by “*”) which are associated
with those sentiment categories:
• Positive Emotion: words such as love, nice,
sweet, fantastic, heal, decent, honest, hope, word
stems such as ecsta* (ecstatic), encourag* (en-
courage), magnific* (magnificent), and emoticons
associated with positive emotion such as :), (:.
• Negative Emotion: words and word stems such
as agony, destruct, pain, resent, ignorant, dis-
satisf* (dissatisfaction), outrag* (outrage), vul-
nerab* (vulnerable) and suffering, and emoticons
associated with negative emotions such as :( are
also included in the negative emotion category.
• Anger: words and word stems such as hate, kill,
brutal, hostil* (hostile), rude, sinister, rape, prej-
udic* (prejudice), beaten, aggressive.
• Anxiety: words and word stems such as wor-
ried, feared, nervous, worry, anxious, afraid, em-
barras* (embarrassed), paranoi* (paranoid), sus-
pico* (suspicious).
LIWC also contains a mean score for each of the
above listed categories for both English and German
languages. These means are available for different
data sources, one of which is Twitter for the English
language (Pennebaker et al., 2015b) and general data
for the German language. We use these means as a
baseline to compare the sentiment scores of each ex-
periment as outlined in the LIWC 2015 and 2007 doc-
umentation guides (Pennebaker et al., 2015a) (Pen-
nebaker et al., 2007). We will refer to these means as
“LIWC mean” in the results. In addition, we also cal-
culate our own mean score based only on our dataset.
We refer to this mean as “Calculated mean” in the re-
sults.
The tweet object creation date was used to or-
der all tweet objects resulting from the pre-processing
stage. For each language, and for each day, the tweet
text contained within each tweet object for that day
was extracted and stored in a single file. Each text file
representing each day and language was then passed
to the LIWC tool. A category score for each of the
LIWC categories was calculated per day, per lan-
guage. LIWC generates this score based on the total
number of words present within the tweets that match
words, word stems, emoticons and expressions cate-
gorized within the specified categories of the English
and German internal LIWC dictionaries.
In addition to using the LIWC scores for the four
emotion categories, the analysis of two significant
events is supported by the generation of weighted
word maps. These were produced using the top-30
words (with stop words removed) found per day and
graphically represented using the wordclouds tool
2
.
4 RESULTS AND DISCUSSION
Section 4.1 presents the LIWC results focusing on the
comparison across sentiment categories and popula-
tions. Section 4.2 also considers the same LIWC re-
sults, in addition to word maps, but focuses on only
two days in particular: 13th September 2015 and 31st
December 2015.
For all sentiment category graphs showing LIWC
scores over the time range of the study, the X axis rep-
resents the day in the time range and the Y axis con-
tains the daily scores produced by LIWC for a partic-
ular emotion category. Each sentiment category graph
contains two means per language: the LIWC mean is
the average LIWC mean (independent of the refugee
crisis data) and is unique for each category as already
discussed. The “calculated” mean represents the cal-
culated average score using the results produced by
the LIWC tool for the refugee crisis data gathered in
this study. Using this “calculated” mean the standard
deviation was also calculated for each category to al-
low the significance of the LIWC results for each day
to be discussed. As is evident in the sentiment cate-
gory graphs in the results section, the results fluctuate,
over time, for each day, demonstrating, for example,
an increase or decrease in a sentiment category.
4.1 Sentiment Category Results: per
Population and per Day
This section will present and discuss the LIWC results
in detail comparing both English and German results
together, per sentiment category: Negative Emotion,
Anger, Anxiety and Positive Emotion. Recall that
each tweet contains at least one of the search terms
relating to the refugee crisis, as outlined in Section
3.1.
• Negative Emotion. The LIWC results for neg-
ative emotion are illustrated in Figure 1 for both
English and German. The calculated means for
the English and German sets of results are both
above the LIWC mean. This suggests that, over
the entire datasets, for each day and in each lan-
guage, tweets relating to the refugee crisis show
a higher negative emotion level compared to the
LIWC averages. It can be seen in Figure 1 that
there are very few days that are below the LIWC
negative emotion mean. Also notable is the lack
2
Free online wordcloud generator.
KDIR 2016 - 8th International Conference on Knowledge Discovery and Information Retrieval
302