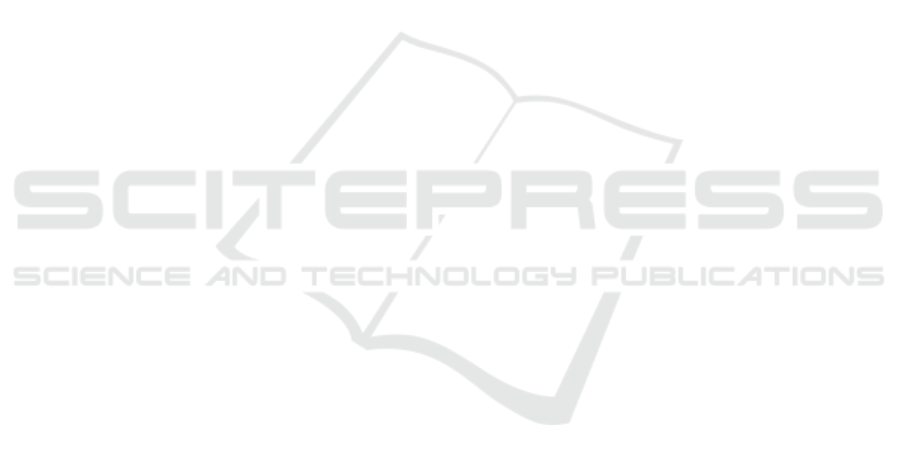
Ghosh, A., Li, G., Veale, T., Rosso, P., Shutova, E., Barn-
den, J., and Reyes, A. (2015a). Semeval-2015 task
11: Sentiment analysis of figurative language in twit-
ter. In Proceedings of the 9th International Workshop
on Semantic Evaluation, pages 470–478.
Ghosh, D., Guo, W., and Muresan, S. (2015b). Sarcas-
tic or not: Word embeddings to predict the literal or
sarcastic meaning of words. In Proceedings of the
2015 Conference on Empirical Methods in Natural
Language Processing, pages 1003–1012.
Gonz
´
alez-Ib
´
anez, R., Muresan, S., and Wacholder, N.
(2011). Identifying sarcasm in twitter: a closer look.
In Proceedings of the 49th Annual Meeting of the As-
sociation for Computational Linguistics: Human Lan-
guage Technologies: short papers-Volume 2, pages
581–586. Association for Computational Linguistics.
Hern
´
andez-Far
´
ıas, I., Bened
´
ı, J.-M., and Rosso, P. (2015).
Applying basic features from sentiment analysis for
automatic irony detection. In Pattern Recognition and
Image Analysis, pages 337–344. Springer.
Huang, E. H., Socher, R., Manning, C. D., and Ng, A. Y.
(2012). Improving word representations via global
context and multiple word prototypes. In Proceed-
ings of the 50th Annual Meeting of the Association
for Computational Linguistics: Long Papers-Volume
1, pages 873–882. Association for Computational Lin-
guistics.
Jijkoun, V., de Rijke, M., and Weerkamp, W. (2010). Gen-
erating focused topic-specific sentiment lexicons. In
Proceedings of the 48th Annual Meeting of the Associ-
ation for Computational Linguistics, pages 585–594.
Association for Computational Linguistics.
Jo, Y. and Oh, A. H. (2011). Aspect and sentiment unifi-
cation model for online review analysis. In Proceed-
ings of the 4th ACM International Conference on Web
Search and Data Mining, WSDM ’11, pages 815–824,
New York, NY, USA. ACM.
Kaji, N. and Kitsuregawa, M. (2007). Building lexicon
for sentiment analysis from massive collection of html
documents. In Proceedings of the 2007 Joint Confer-
ence on Empirical Methods in Natural Language Pro-
cessing and Computational Natural Language Learn-
ing, pages 1075–1083, Prague, Czech Republic. As-
sociation for Computational Linguistics.
Katz, A. N., Colston, H., and Katz, A. (2005). Dis-
course and sociocultural factors in understanding non-
literal language. In Figurative language comprehen-
sion: Social and cultural influences, pages 183–207.
Lawrence Erlbaum Associates, Inc. Mahwah, NJ.
Lin, C. and He, Y. (2009). Joint sentiment/topic model for
sentiment analysis. In Proceedings of the 18th ACM
Conference on Information and Knowledge Manage-
ment, pages 375–384. ACM.
Lu, Y., Castellanos, M., Dayal, U., and Zhai, C. (2011).
Automatic construction of a context-aware sentiment
lexicon: An optimization approach. In Proceedings
of the 20th International Conference on World Wide
Web, pages 347–356. ACM.
McDonald, S. (1999). Exploring the process of inference
generation in sarcasm: A review of normal and clini-
cal studies. Brain and Language, 68(3):486–506.
Mei, Q., Ling, X., Wondra, M., Su, H., and Zhai, C. (2007).
Topic sentiment mixture: modeling facets and opin-
ions in weblogs. In Proceedings of the 16th Inter-
national Conference on World Wide Web, pages 171–
180. ACM.
Mikolov, T., Chen, K., Corrado, G., and Dean, J. (2013).
Efficient estimation of word representations in vector
space. CoRR, abs/1301.3:1–12.
Mohammad, S., Dunne, C., and Dorr, B. (2009). Generat-
ing high-coverage semantic orientation lexicons from
overtly marked words and a thesaurus. In Proceedings
of the 2009 Conference on Empirical Methods in Nat-
ural Language Processing: Volume 2-Volume 2, pages
599–608. Association for Computational Linguistics.
Pedersen, T., Patwardhan, S., and Michelizzi, J. (2004).
Wordnet::similarity: Measuring the relatedness of
concepts. In Demonstration Papers at HLT-NAACL
2004, HLT-NAACL–Demonstrations ’04, pages 38–
41, Stroudsburg, PA, USA. Association for Computa-
tional Linguistics.
Pt
´
a
ˇ
cek, T., Habernal, I., and Hong, J. (2014). Sarcasm
detection on czech and english twitter. In Proceed-
ings of the 25th International Conference on Com-
putational Linguistics: Technical Papers, pages 213–
223, Dublin, Ireland. Dublin City University and As-
sociation for Computational Linguistics.
Rajadesingan, A., Zafarani, R., and Liu, H. (2015). Sarcasm
detection on twitter: A behavioral modeling approach.
In Proceedings of the 8th ACM International Confer-
ence on Web Search and Data Mining, pages 97–106.
ACM.
Rao, D. and Ravichandran, D. (2009). Semi-supervised po-
larity lexicon induction. In Proceedings of the 12th
Conference of the European Chapter of the Associ-
ation for Computational Linguistics, pages 675–682.
Association for Computational Linguistics.
Reyes, A. and Rosso, P. (2014). On the difficulty of au-
tomatically detecting irony: beyond a simple case
of negation. Knowledge and Information Systems,
40(3):595–614.
Reyes, A., Rosso, P., and Veale, T. (2013). A multidimen-
sional approach for detecting irony in twitter. Lan-
guage resources and evaluation, 47(1):239–268.
Riloff, E., Qadir, A., Surve, P., De Silva, L., Gilbert, N.,
and Huang, R. (2013). Sarcasm as contrast between a
positive sentiment and negative situation. In Proceed-
ings of the 2013 Conference on Empirical Methods in
Natural Language Processing, pages 704–714. Asso-
ciation for Computational Linguistics.
Rumelhart, D. E., Hinton, G. E., and Williams, R. J. (1986).
Learning representations by back-propagating errors.
Nature, 323:533–536.
Turian, J., Ratinov, L., and Bengio, Y. (2010). Word rep-
resentations: a simple and general method for semi-
supervised learning. In Proceedings of the 48th An-
nual Meeting of the Association for Computational
Linguistics, pages 384–394. Association for Compu-
tational Linguistics.
Weitzel, L., Prati, R. C., and Aguiar, R. F. (2016). The
Comprehension of Figurative Language: What Is the
Influence of Irony and Sarcasm on NLP Techniques?,
pages 49–74. Springer International Publishing.
KDIR 2016 - 8th International Conference on Knowledge Discovery and Information Retrieval
76