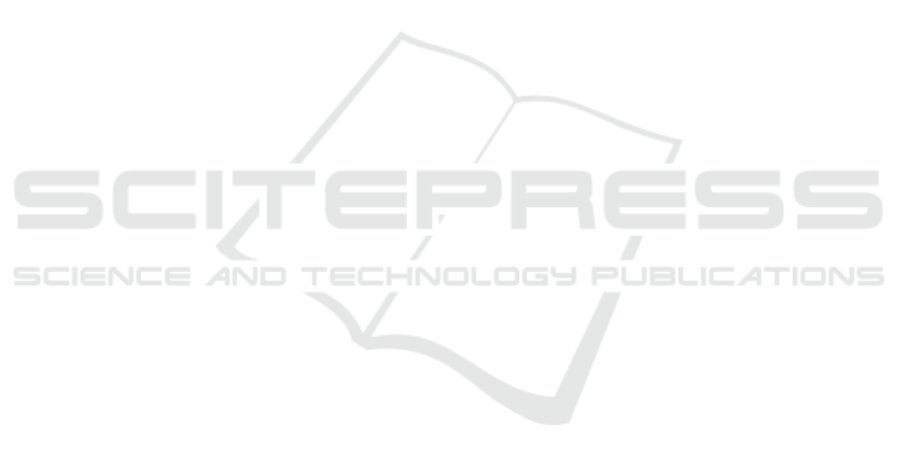
An example is illustrated in Fig. 2I that shows
a diagram of a typical adaptation experiment to
demonstrate after-effects in action perception. First,
an unambiguous adaptation stimulus (TOWARDS or
AWAY) is presented to participants, where the dura-
tion of the adaptor (2, 6, 10, 14, 18 or 22 gait cycles)
was varied over different blocks of the experiment.
After this stimulus (and a fixed Inter-stimulus Inter-
val of 2.8 s) an ambiguous test stimulus (SILHOU-
ETTE) is presented for 3 gait cycles, asking for the
perceived walking direction. The predicted results for
such an experiment (from 20 repeated simulations)
are presented in Fig. 2J, which shows the probabil-
ities of the percept for the ambiguous test stimulus
(which was identical in all cases). With increasing
the duration of the adaptor stimulus the probability
that participants perceive the test stimulus as walking
in the same direction as the adaptor decreases. A sig-
nificant decrease of the percept probability (from 0.5
without adaptator presentation) is already perceived
for the shortest adaptor duration of 2 gait cycles, and
we observed a further decrease with longer adaptor
durations (where 1 gait cycle corresponds to 1.4 sec-
onds of stimulus duration). This behavior is consis-
tent with after-effects, as investigated previously for
many modalities (motion, lightness, etc) in low-level
vision. Such after-effects for action perception with a
similar time course have been shown for other types
of action stimuli in the literature (see (Barraclough
and Jellema, 2011), (de la Rosa et al., 2014)), and we
are presently running psychophysical experiments to
verify this prediction of the model in detail.
A further set of experiments that we are presently
running, and for which the model provides quantita-
tive predictions, investigates the interdependence of
the stability of action percepts and the switching times
between the different percepts (which depend on the
mean-first passage times of the corresponding attrac-
tors). This extends studies that have been made for
muti-stability of low-level motion perception (Hock
et al., 1993) to the domain of action perception.
5 CONCLUSIONS
To our knowledge, we have described the first
biologically-inspired neural model that accounts si-
multaneously for the following properties of body
motion perception: (i) perceptual multi-stability and
switching, (ii) switching time statistics and (iii) the in-
fluence of shading information on the perceptual dy-
namics. We showed that the model reproduces the
psychophysically observed phenomenology and dis-
tributions of the percept times. Since the model is
based on learned templates, these results would trans-
fer trivially to other action patterns with the similar
form of bistability in the view domain.
It is important to stress that the goal of this pa-
per was the modeling of the perceptual dynamics, and
neither the proposal of novel deep shape or action
recognition architecture, nor the claim that the pro-
posed two-pathway architecture is significantly bet-
ter for shape recognition. Testing this claim would
require additional experiments with larger data sets,
and was not the focus of this paper. Also it remains to
be shown whether any of the popular recurrent deep
architectures reproduce the details of the human per-
ceptual dynamics.
Future work will have to extend the model for
more stimuli and include more accurate fits of exper-
imental data.
ACKNOWLEDGEMENTS
The first author thanks Tjeerd Dijkstra for his insight-
ful commentary on the analysis of the Amari field
behavior. Funded by: BMBF, FKZ: 01GQ1002A,
ABC PITN-GA-011-290011, CogIMon H2020 ICT-
644727; HBP FP7-604102; Koroibot FP7-611909,
DFG GZ: KA 1258/15-1.
REFERENCES
Amari, S. (1977). Dynamics of pattern formation in lateral
inhibition type neural fields. Biological Cybernetics.
Andersen, R. and Bradley, D. (1998). Perception of three-
dimensional structure from motion. Trends in Cogni-
tive Sciences.
Barraclough, N. and Jellema, T. (2011). Visual aftereffects
for walking actions reveal underlying neural mecha-
nisms for action recognition. Psychological Science.
Blake, R. and Logothesis, N. (2001). Visual competition.
Nature Review Neuroscience.
de la Rosa, S., Streuber, S., Giese, M., Buelthoff, H., and
Curio, C. (2014). Putting actions in context: Visual
action adaptation aftereffects are modulated by social
contexts. PLOS One.
Edwards, M., Deng, J., and Xie, X. (2016). From pose to
activity. In Computer Vision and Image Understand-
ing. Elsevier Science Inc.
Escobar, M. and Kornprobst, P. (2008). Action recogni-
tion with a bioinspired feedforward motion processing
model: the richness of center-surround interactions.
In ECCV’08. 10th European Conference on Computer
Vision. Springer Berlin Heidelberg.
Fleischer, F., Caggiano, V., Thier, P., and Giese, M. (2013).
Physiologically inspired model for the visual recog-
Neural Model for the Influence of Shading on the Multistability of the Perception of Body Motion
75