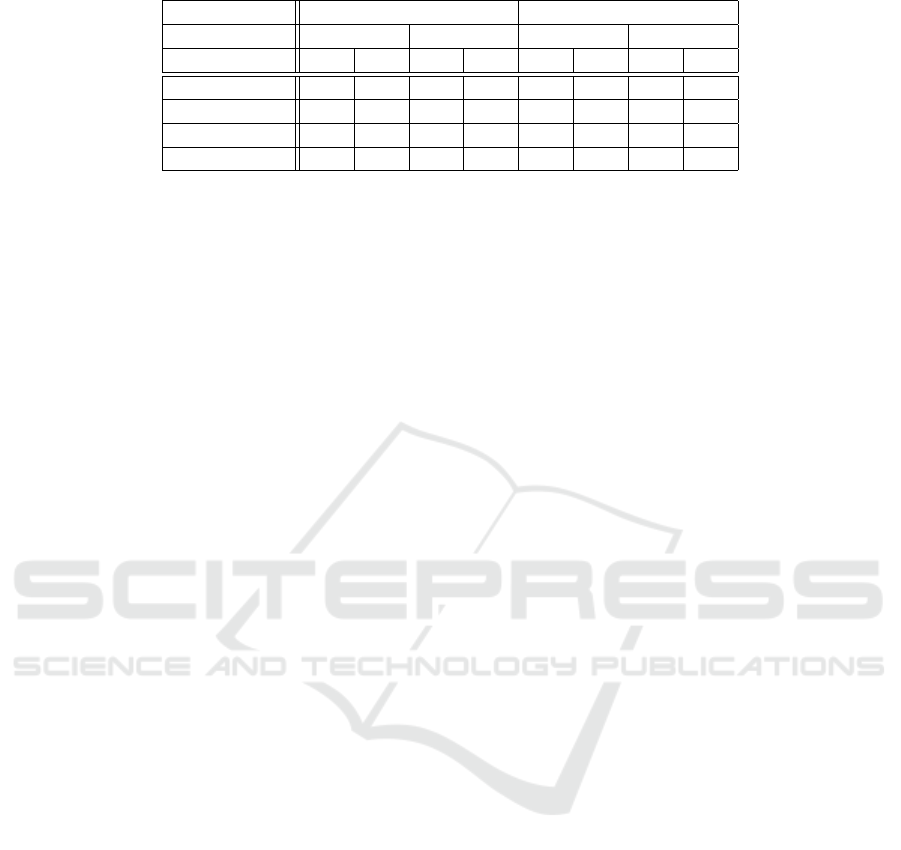
Table 2: Efficiency of prediction methods, considering the situation.
environment Indoor Outdoor
transitions Det. Stoc. Det. Stoc.
hesitation no yes no yes no yes no yes
Force ++ ++ ++ ++ ++ ++ ++ ++
Learn ++ ++ ++ + − − − −
Dist + + ++ − ++ − − −
TAMER&RL + + − − + + − −
method who learns mistakes and take a lot of time
before learning. TAMER&RL is much stable with
deterministic actions than ”Force” and ”Learn” meth-
ods, but less efficient. In stochastic transition cases,
TAMER&RL is outperformed by every other method.
In outdoor environment, ”Dist” method seems to be
much adapted, contrary to ”Learn” method.
In short-term, we will integrate our methods in a
multi-robot system, developed in a national project,
on the sensitive site surveillance example where a
robot is tele-operated by a professional operator and
the other should predict its policy and compute a co-
ordinated policy to head the same destination.
ACKNOWLEDGEMENT
We would like to thank the DGA (General Direction
of Arming), Dassault-Aviation and Nexter Robotics
for their financial participation for these results.
REFERENCES
Abdel-Illah Mouaddib, L. J. and Zilberstein, S. (2015).
Handling advice in mdps for semi-autonomous sys-
tems. In ICAPS Woskhop on Planning and Robotics
(PlanRob), pages 153–160.
He, H., Eisner, J., and Daume, H. (2012). Imitation learning
by coaching. In Pereira, F., Burges, C., Bottou, L., and
Weinberger, K., editors, Advances in Neural Informa-
tion Processing Systems 25, pages 3149–3157. Curran
Associates, Inc.
H
¨
uttenrauch, H. and Severinson Eklundh, K. (2006). Be-
yond usability evaluation: Analysis of human-robot
interaction at a major robotics competition. Interac-
tion Studies, 7(3):455–477.
Knox, W. and Stone, P. (2008). Tamer: Training an agent
manually via evaluative reinforcement. In Develop-
ment and Learning, 2008. ICDL 2008. 7th IEEE In-
ternational Conference on, pages 292–297.
Knox, W. B. and Stone, P. (2010). Combining manual
feedback with subsequent MDP reward signals for re-
inforcement learning. In Proc. of 9th Int. Conf. on
Autonomous Agents and Multiagent Systems (AAMAS
2010).
Knox, W. B. and Stone, P. (2012). Reinforcement learning
with human and mdp reward. In Proceedings of the
11th International Conference on Autonomous Agents
and Multiagent Systems (AAMAS 2012).
Monderer, D. and Shapley, L. S. (1996). Potential games.
Games and economic behavior, 14(1):124–143.
Nair, R., Tambe, M., Yokoo, M., Pynadath, D. V., and
Marsella, S. (2003). Taming decentralized pomdps:
Towards efficient policy computation for multiagent
settings. In IJCAI-03, Proceedings of the Eighteenth
International Joint Conference on Artificial Intelli-
gence, Acapulco, Mexico, August 9-15, 2003, pages
705–711.
Panagou, D. and Kumar, V. (2014). Cooperative Visibility
Maintenance for Leader-Follower Formations in Ob-
stacle Environments. Robotics, IEEE Transactions on,
30(4):831–844.
Paruchuri, P., Pearce, J. P., Marecki, J., Tambe, M., Or-
donez, F., and Kraus, S. (2008). Playing games
for security: An efficient exact algorithm for solving
bayesian stackelberg games. In Proceedings of the 7th
International Joint Conference on Autonomous Agents
and Multiagent Systems - Volume 2, AAMAS ’08,
pages 895–902, Richland, SC. International Founda-
tion for Autonomous Agents and Multiagent Systems.
Pashenkova, E., Rish, I., and Dechter, R. (1996). Value it-
eration and policy iteration algorithms for markov de-
cision problem. In AAAI’96: Workshop on Structural
Issues in Planning and Temporal Reasoning. Citeseer.
Puterman, M. L. (1994). Markov Decision Processes: Dis-
crete Stochastic Dynamic Programming. John Wiley
& Sons, Inc., New York, NY, USA, 1st edition.
Shiomi, M., Sakamoto, D., Kanda, T., Ishi, C. T., Ishiguro,
H., and Hagita, N. (2008). A semi-autonomous com-
munication robot: a field trial at a train station. In
Proceedings of the 3rd ACM/IEEE International Con-
ference on Human Robot Interaction, pages 303–310,
New York, NY, USA. ACM.
Sigaud, O. and Buffet, O. (2010). Markov Decision Pro-
cesses in Artificial Intelligence. Wiley-ISTE.
Sutton, R. S. and Barto, A. G. (1998). Introduction to Re-
inforcement Learning. MIT Press, Cambridge, MA,
USA.
Vorobeychik, Y., An, B., and Tambe, M. (2012). Adver-
sarial patrolling games. In Proceedings of the 11th
International Conference on Autonomous Agents and
Multiagent Systems - Volume 3, AAMAS ’12, pages
1307–1308, Richland, SC. International Foundation
for Autonomous Agents and Multiagent Systems.
Watkins, C. and Dayan, P. (1992). Q-learning. Machine
Learning, 8(3-4):279–292.
ECTA 2016 - 8th International Conference on Evolutionary Computation Theory and Applications
200