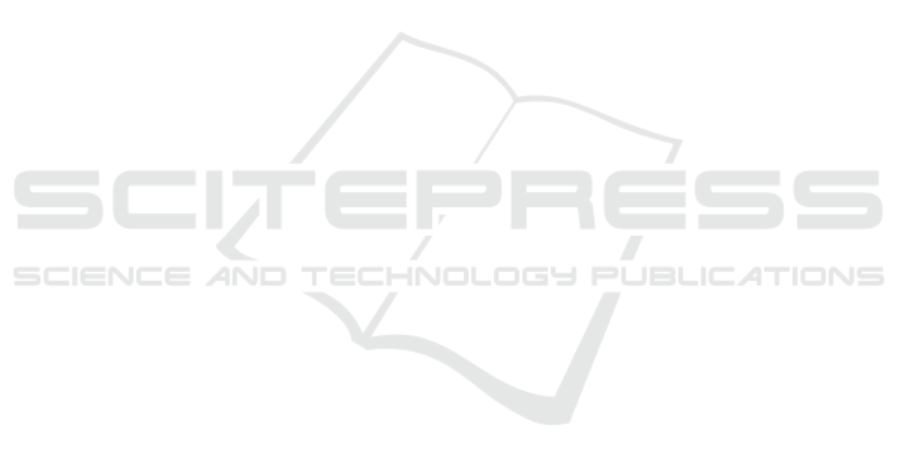
increased feedback control. SM and MD increased
their NIV and therefore seemed to focus more on
shape constraints during the intervention. TK and SB
decreased their NIV and possibly relied less on feed-
back during later stages. The Variance in partly
contradicting NIV and velocity values can be
explained as subjects are seeking the optimal solution
for a speed-accuracy trade-off during learning.
A clear progress was visible for RP, however.
Lower SD indicates the formation of increasingly
stable attractors, which supports the idea that writing
can be modelled by updating RP, amplitude and
frequency on a piecewise manner to spare neural
resources (Andre et al., 2014). Semi-permanent stable
RP would support automation as well. However, well
learned letters exhibit more than one stable phase
angle and are therefore not normally distributed. The
transition between those angles is of special interest
and can be further investigated by means of pattern or
time series analyses.
We planned to reveal cortical adaptations by
examining spectral maps for all participants. Perfetti
et al. (2011) demonstrated that enhanced gamma
activity in right parietal regions is associated with
initial learning. We found that all subjects had
increased power in this area, as well as in other areas
in the task condition. Gamma activity remained
enhanced shortly after the task, indicating that
memory formation processes were still active.
Increased activity over the whole cortex indicates
engagement of a wide-spread network during grapho-
motor learning. High-density EEG could help
localizing involved areas with a better resolution.
Wong et al. (2014) revealed that theta and gamma
activities in the frontal cortex are having a negative
relationship with task familiarity. In this line, we
revealed lower frontal theta activities in session 9 for
four subjects, while theta power was increased in
other areas (especially occipital) during the task. This
finding supports the idea that subjects need less
attention with higher task familiarity.
Our aim was to gather insight about motor and
neural parameters during eight weeks of learning. We
found inter-individual differences for both, which
could reflect using different strategies or learning
with different speeds. Additionally, we discovered
common features for RP as well as gamma and theta
activities. Future studies could correlate behavioural
with high-density EEG data (e.g. NIV with frontal
activity) to reveal coherent adaptations or possible
strategy-related differences in the neural network.
REFERENCES
André, G., Kostrubiec, V., Buisson, J.-C., Albaret, J.-M., &
Zanone, P.-G. (2014). A parsimonious oscillatory
model of handwriting. Biological cybernetics, 108(3),
321–336.
Athenes, S., Sallagoïty, I., Zanone, P.-G., & Albaret, J.-M.
(2004). Evaluating the coordination dynamics of
handwriting. Human movement science, 23(5), 621–
641.
Bar, R. J., & DeSouza, J. F. X. (2016). Tracking plasticity:
effects of long-term rehearsal in expert dancers
encoding music to movement. PloS one, 11(1),
e0147731.
Danna, J., & Velay, J.-L. (2015). Basic and supplementary
sensory feedback in handwriting. Frontiers in
psychology, 6.
Delorme, A., & Makeig, S. (2004). EEGLAB: an open
source toolbox for analysis of single-trial EEG
dynamics including independent component analysis.
Journal of neuroscience methods, 134(1), 9–21.
Haken, H., Kelso, J. S., & Bunz, H. (1985). A theoretical
model of phase transitions in human hand movements.
Biological cybernetics, 51(5), 347–356.
Hollerbach, J. M. (1981). An oscillation theory of
handwriting. Biological cybernetics, 39(2), 139–156.
Jasper, H. (1958). Report of the committee on methods of
clinical examination in electroencephalography.
Electroencephalogr Clin Neurophysiol, 10, 370–375.
Mai, N., & Marquardt, C. (1998). Registrierung und
Analyse von Schreibbewegungen: Fragen an den
Schreibunterricht: na.
Marquardt, C., & Mai, N. (1994). A computational
procedure for movement analysis in handwriting.
Journal of neuroscience methods, 52(1), 39–45.
Patel, R., Spreng, R. N., & Turner, G. R. (2013). Functional
brain changes following cognitive and motor skills
training a quantitative meta-analysis. Neurorehabilita-
tion and neural repair, 27(3), 187–199.
Perfetti, B., Moisello, C., Landsness, E. C., Kvint, S.,
Lanzafame, S., Onofrj, M., et al. (2011). Modulation of
gamma and theta spectral amplitude and phase
synchronization is associated with the development of
visuo-motor learning. The Journal of Neuroscience,
31(41), 14810–14819.
Razali, N. M., & Wah, Y. B. (2011). Power comparisons of
shapiro-wilk, kolmogorov-smirnov, lilliefors and
anderson-darling tests. Journal of statistical modeling
and analytics, 2(1), 21–33.
Ritter, F. E., & Schooler, L. J. (2001). The learning curve.
International encyclopedia of the social and behavioral
sciences, 13, 8602–8605.
Schöllhorn, W. I., Eekhoff, A., & Hegen, P. (2015).
Systemdynamik und differenzielles Lernen.
Sportwissenschaft, 45(3), 127–137.
Wong, S. W. H., Chan, R. H. M., & Mak, J. N. (2014).
Spectral modulation of frontal EEG during motor skill
acquisition: a mobile EEG study. International Journal
of Psychophysiology, 91(1), 16–21.
Motor and Neural Adaptation during an Eight-Week Writing Training with the Non-Preferred Hand
13