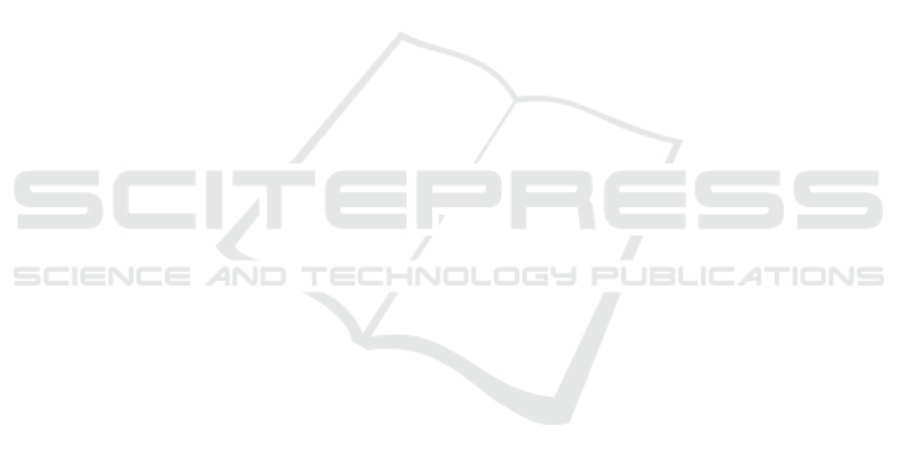
and balancing. International Journal of Forecasting,
28(1):224–238.
Davis, R., Edelman, D., and Gammerman, A. (1992).
Machine-learning algorithms for credit-card applica-
tions. IMA Journal of Management Mathematics,
4(1):43–51.
Dean, J. and Ghemawat, S. (2008). Mapreduce: simpli-
fied data processing on large clusters. Commun. ACM,
51(1):107–113.
Desai, V. S., Crook, J. N., and Overstreet, G. A. (1996).
A comparison of neural networks and linear scoring
models in the credit union environment. European
Journal of Operational Research, 95(1):24–37.
Donmez, P., Carbonell, J. G., and Bennett, P. N. (2007).
Dual strategy active learning. In Kok, J. N., Ko-
ronacki, J., de M
´
antaras, R. L., Matwin, S., Mladenic,
D., and Skowron, A., editors, Machine Learning:
ECML 2007, 18th European Conference on Machine
Learning, Warsaw, Poland, September 17-21, 2007,
Proceedings, volume 4701 of Lecture Notes in Com-
puter Science, pages 116–127. Springer.
Doumpos, M. and Zopounidis, C. (2014). Credit scoring.
In Multicriteria Analysis in Finance, pages 43–59.
Springer.
Faraggi, D. and Reiser, B. (2002). Estimation of the area un-
der the roc curve. Statistics in medicine, 21(20):3093–
3106.
Fensterstock, A. (2005). Credit scoring and the next step.
Business Credit, 107(3):46–49.
Fern
´
andez-Tob
´
ıas, I., Tomeo, P., Cantador, I., Noia, T. D.,
and Sciascio, E. D. (2016). Accuracy and diver-
sity in cross-domain recommendations for cold-start
users with positive-only feedback. In Sen, S., Geyer,
W., Freyne, J., and Castells, P., editors, Proceed-
ings of the 10th ACM Conference on Recommender
Systems, Boston, MA, USA, September 15-19, 2016,
pages 119–122. ACM.
Hand, D. J. (2009). Measuring classifier performance: a
coherent alternative to the area under the ROC curve.
Machine Learning, 77(1):103–123.
He, H. and Garcia, E. A. (2009). Learning from imbalanced
data. IEEE Trans. Knowl. Data Eng., 21(9):1263–
1284.
Henley, W. et al. (1997). Construction of a k-nearest-
neighbour credit-scoring system. IMA Journal of
Management Mathematics, 8(4):305–321.
Henley, W. and Hand, D. J. (1996). A k-nearest-neighbour
classifier for assessing consumer credit risk. The
Statistician, pages 77–95.
Henley, W. E. (1994). Statistical aspects of credit scoring.
PhD thesis, Open University.
Hsieh, N.-C. (2005). Hybrid mining approach in the design
of credit scoring models. Expert Systems with Appli-
cations, 28(4):655–665.
Japkowicz, N. and Stephen, S. (2002). The class imbal-
ance problem: A systematic study. Intell. Data Anal.,
6(5):429–449.
Lee, T.-S. and Chen, I.-F. (2005). A two-stage hybrid
credit scoring model using artificial neural networks
and multivariate adaptive regression splines. Expert
Systems with Applications, 28(4):743–752.
Lessmann, S., Baesens, B., Seow, H., and Thomas, L. C.
(2015). Benchmarking state-of-the-art classifica-
tion algorithms for credit scoring: An update of re-
search. European Journal of Operational Research,
247(1):124–136.
Lika, B., Kolomvatsos, K., and Hadjiefthymiades, S.
(2014). Facing the cold start problem in recommender
systems. Expert Syst. Appl., 41(4):2065–2073.
Marqu
´
es, A. I., Garc
´
ıa, V., and S
´
anchez, J. S. (2013). On the
suitability of resampling techniques for the class im-
balance problem in credit scoring. JORS, 64(7):1060–
1070.
Mester, L. J. et al. (1997). Whats the point of credit scoring?
Business review, 3:3–16.
Moler, C. B. (2004). Numerical computing with MATLAB.
SIAM.
Morrison, J. (2004). Introduction to survival analysis
in business. The Journal of Business Forecasting,
23(1):18.
Ong, C.-S., Huang, J.-J., and Tzeng, G.-H. (2005). Build-
ing credit scoring models using genetic programming.
Expert Systems with Applications, 29(1):41–47.
Powers, D. M. (2011). Evaluation: from precision, recall
and f-measure to roc, informedness, markedness and
correlation.
Pozzolo, A. D., Caelen, O., Borgne, Y. L., Waterschoot, S.,
and Bontempi, G. (2014). Learned lessons in credit
card fraud detection from a practitioner perspective.
Expert Syst. Appl., 41(10):4915–4928.
Quah, J. T. S. and Sriganesh, M. (2008). Real-time credit
card fraud detection using computational intelligence.
Expert Syst. Appl., 35(4):1721–1732.
Reichert, A. K., Cho, C.-C., and Wagner, G. M. (1983).
An examination of the conceptual issues involved in
developing credit-scoring models. Journal of Business
& Economic Statistics, 1(2):101–114.
Salzberg, S. (1997). On comparing classifiers: Pitfalls
to avoid and a recommended approach. Data Min.
Knowl. Discov., 1(3):317–328.
Son, L. H. (2016). Dealing with the new user cold-start
problem in recommender systems: A comparative re-
view. Inf. Syst., 58:87–104.
Thanuja, V., Venkateswarlu, B., and Anjaneyulu, G. (2011).
Applications of data mining in customer relationship
management. Journal of Computer and Mathematical
Sciences Vol, 2(3):399–580.
Vinciotti, V. and Hand, D. J. (2003). Scorecard construc-
tion with unbalanced class sizes. Journal of Iranian
Statistical Society, 2(2):189–205.
Wang, G., Hao, J., Ma, J., and Jiang, H. (2011). A compar-
ative assessment of ensemble learning for credit scor-
ing. Expert Syst. Appl., 38(1):223–230.
Wang, G., Ma, J., Huang, L., and Xu, K. (2012). Two credit
scoring models based on dual strategy ensemble trees.
Knowl.-Based Syst., 26:61–68.
Zhu, J., Wang, H., Yao, T., and Tsou, B. K. (2008). Active
learning with sampling by uncertainty and density for
word sense disambiguation and text classification. In
Scott, D. and Uszkoreit, H., editors, COLING 2008,
22nd International Conference on Computational Lin-
guistics, Proceedings of the Conference, 18-22 August
2008, Manchester, UK, pages 1137–1144.
KDIR 2016 - 8th International Conference on Knowledge Discovery and Information Retrieval
120