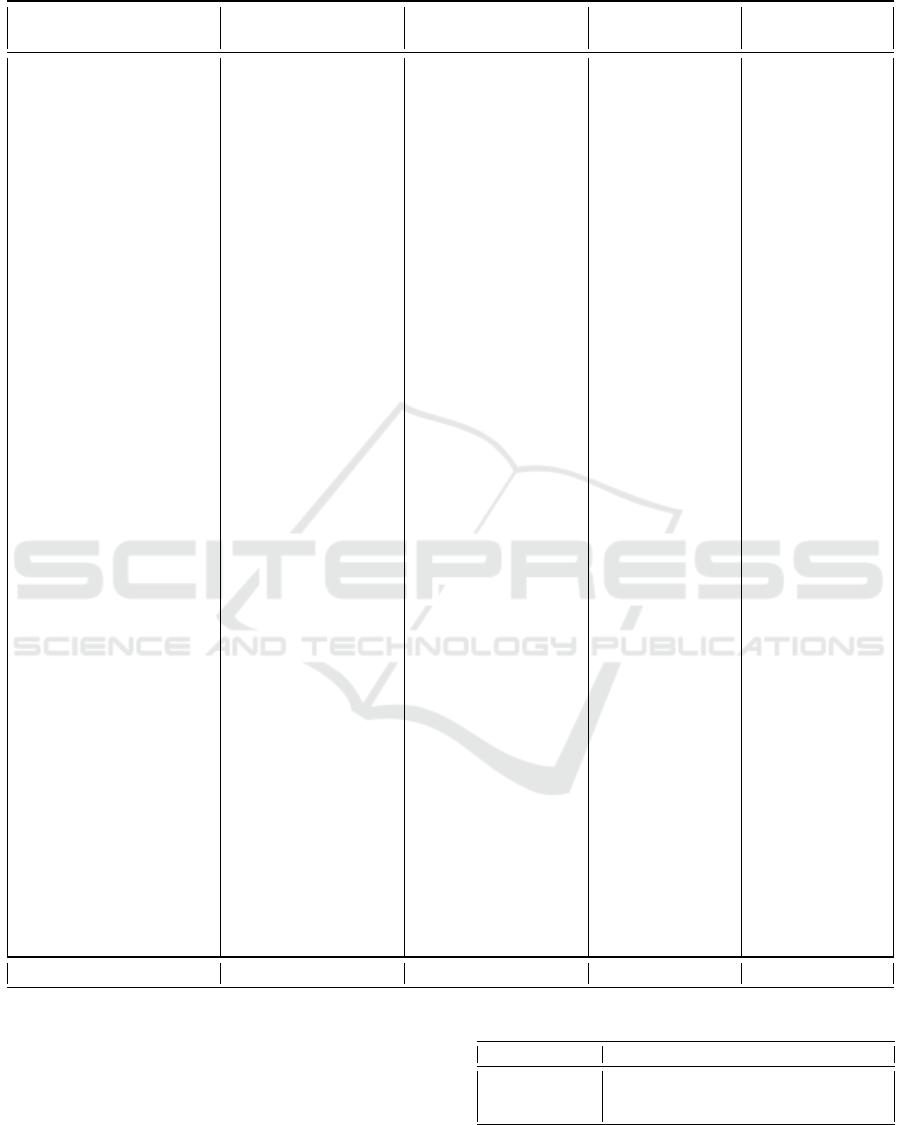
Table 2: The best value for the parameters with the corresponding classification error percentage for LCSS, CID-LCSS,
dLCSS and CID-dLCSS.
LCSS CID-LCSS dLCSS CID-dLCSS
Datasets R ε Error R ε Error R |A| Error R |A| Error
50words 10 0,2 21,11 10 0,2 20,44 10 32 22,22 10 16 21,33
Adiac 1 0,05 38,71 1 0,05 35,12 1 256 49,23 1 256 47,69
Beef 2 0,1 46,66 2 0,1 53,33 1 32 46,67 1 32 53,33
CBF 1 0,1 3,33 1 0,2 0 6 512 0 1 8 0
ChlorineConcentration 0 0,2 27,83 0 0,2 28,9 0 32 30,41 0 16 31,91
CinCECGtorso 1 0,8 10 2 0,8 7,5 2 16 12,5 1 16 12,5
Coffee 4 0,1 0 1 0,2 0 1 32 0 1 32 0
CricketX 9 0,4 22,56 5 0,4 20,51 9 16 23,59 6 16 21,03
CricketY 9 0,4 23,33 6 0,4 27,94 9 8 29,74 5 8 29,49
CricketZ 8 0,4 23,84 5 0,4 22,56 10 8 23,59 9 8 20,51
DiatomSizeReduction 1 0,00625 6,25 1 0,00625 6,25 1 32 6,25 1 32 6,25
ECG200 4 0,4 12 2 0,2 12 4 16 12 4 16 10
ECGFiveDays 8 0,0125 8,69 0 0,00625 8,69 0 8 17,39 0 8 17,39
FaceAll 6 0,8 2,14 9 0,4 1,78 6 8 1,79 5 8 2,32
FaceFour 1 0,2 4,16 1 0,2 8,33 1 8 4,17 6 8 0
FacesUCR 10 0,4 3,5 7 0,4 4 9 8 3 9 16 2,5
fish 7 0,05 13,14 5 0,025 13,14 4 32 14,86 3 64 16
GunPoint 1 0,2 2 2 0,2 2 3 32 0 2 32 0
Haptics 1 0,4 46,45 8 0,4 43,87 5 8 48,39 2 32 47,1
InlineSkate 5 0,2 48 9 0,00625 44 8 64 50 9 64 47
ItalyPowerDemand 5 0,1 7,46 5 0,025 2,98 5 64 4,48 5 8 5,97
Lighting2 1 0,1 16,66 3 0,1 16,66 9 32 16,67 1 16 21,67
Lighting7 10 0,4 27,14 1 0,1 24,28 1 16 31,43 2 32 31,43
MALLAT 1 0,4 1,81 1 0,4 1,81 1 8 9,09 3 64 5,46
MedicalImages 8 0,2 30,97 4 0,2 29,92 5 32 29,13 9 32 26,51
MoteStrain 4 0,0125 10 2 0,8 15 3 128 10 2 32 20
OliveOil 1 0,00625 6,66 1 0,00625 6,66 1 512 10 1 512 10
OSULeaf 10 0,2 18 10 0,2 19,5 10 32 18 10 16 19
SonyAIBORobotSurface1 2 0,4 10 0 0,00625 0 2 16 10 0 16 0
SonyAIBORobotSurface2 8 0,05 7,4 2 0,05 7,4 2 8 7,41 2 8 7,41
StarLightCurves 10 0,1 10,9 1 0,025 5 5 32 12,2 1 512 5,1
SwedishLeaf 4 0,2 10,8 3 0,2 10,6 10 16 15,2 10 16 13,4
Symbols 6 0,2 4 10 0,025 0 10 16 0 1 64 0
SyntheticControl 7 0,8 2,66 4 0,8 3 4 8 5,67 5 32 3
Trace 3 0,2 2 0 0,4 0 4 32 1 4 32 1
TwoLeadECG 7 0,2 4,34 7 0,1 4,34 3 32 4,35 7 32 4,35
TwoPatterns 9 0,8 0 10 0,2 0 7 16 0 8 8 0
uWaveGestureLibraryX 6 0,4 23,99 7 0,2 21,54 10 16 25 7 16 22,77
uWaveGestureLibraryY 4 0,4 28,01 8 0,4 25,44 7 32 27,68 6 32 26,23
uWaveGestureLibraryZ 8 0,4 26,56 8 0,4 24,44 8 16 28,35 9 32 27,01
wafer 1 0,2 0,1 2 0,2 0 1 4 0 1 4 0
WordsSynonyms 9 0,4 22,47 8 0,2 23,97 9 32 24,35 9 8 24,35
yoga 8 0,05 12 10 0,05 11,66 8 128 13 10 128 14
AVERAGE 15,06 14,29 16,25 15,69
datasets (Chen et al., 2015) and we apply the hold-
one-out validation i.e. one instance is used for testing
and the rest of the instances are used for training the
classifier.
The best values for the parameters are searched in
the intervals presented in Table 1. The chosen values
for the parameters and the corresponding classifica-
tion error percentage are shown in the Table 2.
Table 1: The tested values for the distances parameters.
Parameters Tested values
radius (R) 1%, 2%, 3%, 4%, 5%, 6%, 7%, 8%, 9%, 10%
alphabet size (|A|) 4, 8, 16, 32, 64, 128, 256, 512
epsilon (ε) 0.00625, 0.0125, 0.025, 0.05, 0.1, 0.2, 0.4, 0.8
Table 2 that for 36 from 43 datasets it is better
to apply the complexity invariant factor (CID) and
among them there are 15 cases where the results are
KDIR 2016 - 8th International Conference on Knowledge Discovery and Information Retrieval
340