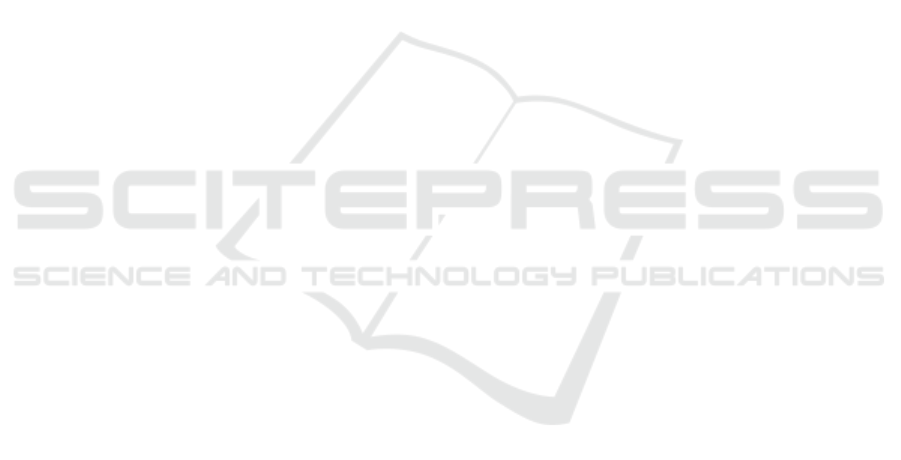
rials: Applications to Brake Friction and Thermoset
Matrix Composites. Woodhead Publishing.
Alonso, C. L., Montana, J. L., and Borges, C. E. (2009).
Evolution strategies for constants optimization in ge-
netic programming. In 21st International Conference
on Tools with Artificial Intelligence, ICTAI ’09, pages
703–707.
Berger, E. (2002). Friction modeling for dynamic system
simulation. Applied Mechanics Reviews, 55(6):535–
577.
Breiman, L. (2001). Random forests. Machine learning,
45(1):5–32.
Castro, J. and Delgado, M. (1996). Fuzzy systems with
defuzzification are universal approximators. IEEE
Transactions on Systems, Man and Cybernetics, part
B: Cybernetics, 26(1):149–152.
Cernuda, C., Lughofer, E., Maerzinger, W., and Kasberger,
J. (2011). NIR-based quantification of process param-
eters in polyetheracrylat (PEA) production using flex-
ible non-linear fuzzy systems. Chemometrics and In-
telligent Laboratory Systems, 109(1):22–33.
Chiu, S. (1994). Fuzzy model identification based on cluster
estimation. Journal of Intelligent and Fuzzy Systems,
2(3):267–278.
De Wit, C. C., Olsson, H., Astrom, K. J., and Lischinsky,
P. (1995). A new model for control of systems with
friction. Automatic Control, IEEE Transactions on,
40(3):419–425.
Deb, K., Pratap, A., Agarwal, S., and Meyarivan, T. (2002).
A fast and elitist multiobjective genetic algorithm:
NSGA-II. Evolutionary Computation, IEEE Transac-
tions on, 6(2):182–197.
Friedman, J. H. (2001). Greedy function approximation: a
gradient boosting machine. Annals of statistics, pages
1189–1232.
Gray, R. (1984). Vector quantization. IEEE ASSP Maga-
zine, 1(2):4–29.
Haenlein, M. and Kaplan, A. (2004). A beginner’s guide
to partial least squares (PLS) analysis. Understanding
Statistics, 3(4):283–297.
Hastie, T., Tibshirani, R., and Friedman, J. (2009). The Ele-
ments of Statistical Learning: Data Mining, Inference
and Prediction - Second Edition. Springer, New York
Berlin Heidelberg.
Hastie, T., Tibshirani, R., and Friedman, J. (2010). Regu-
larized paths for generalized linear models via coordi-
nate descent. Journal of Statistical Software, 33(1).
Hosenfeldt, T., Schulz, E., Glerl, J., and Steinmetz, S.
(2014). Friction tailored to your requirements. In KG,
S. T. G. . C., editor, Solving the Powertrain Puzzle -
10th Schaeffler Symposium, chapter 23, pages 331 –
344. Springer Vieweg.
Keijzer, M. (2003). Improving symbolic regression with
interval arithmetic and linear scaling. In Ryan, C.,
Soule, T., Keijzer, M., Tsang, E., Poli, R., and Costa,
E., editors, Genetic Programming, Proceedings of Eu-
roGP’2003, volume 2610 of LNCS, pages 70–82, Es-
sex. Springer-Verlag.
Kommenda, M., Kronberger, G., Affenzeller, M., Win-
kler, S. M., and Burlacu, B. (2016). Evolving sim-
ple symbolic regression models by multi-objective ge-
netic programming. In Genetic Programming Theory
and Practice XIII (not yet published). Springer.
Kommenda, M., Kronberger, G., Winkler, S., Affenzeller,
M., and Wagner, S. (2013). Effects of constant op-
timization by nonlinear least squares minimization in
symbolic regression. In Proceedings of the 15th an-
nual conference companion on Genetic and evolution-
ary computation, pages 1121–1128. ACM.
Koza, J. R. (1992). Genetic Programming: On the Pro-
gramming of Computers by Means of Natural Selec-
tion. MIT Press, Cambridge, MA, USA.
Kronberger, G. (2011). Symbolic Regression for Knowledge
Discovery – Bloat, Overfitting, and Variable Interac-
tion Networks. Number 64 in Johannes Kepler Uni-
versity, Linz, Reihe C. Trauner Verlag+Buchservice
GmbH.
Langdon, W., Soule, T., Poli, R., and Foster, J. (1999). The
evolution of size and shape. In Advances in Genetic
Programming, volume 3, chapter 8, pages 163–190.
MIT Press.
Lemos, A., Caminhas, W., and Gomide, F. (2011). Mul-
tivariable gaussian evolving fuzzy modeling system.
IEEE Transactions on Fuzzy Systems, 19(1):91–104.
Loh, W.-Y., Basch, R. H., Li, D., and Sanders, P. (2000).
Dynamic modeling of brake friction coefficients.
Technical report, SAE Technical Paper.
Lughofer, E. (2008). FLEXFIS: A robust incremental learn-
ing approach for evolving TS fuzzy models. IEEE
Transactions on Fuzzy Systems, 16(6):1393–1410.
Lughofer, E. (2011). Evolving Fuzzy Systems — Methodolo-
gies, Advanced Concepts and Applications. Springer,
Berlin Heidelberg.
Lughofer, E. (2013). On-line assurance of interpretability
criteria in evolving fuzzy systems — achievements,
new concepts and open issues. Information Sciences,
251:22–46.
Lughofer, E., Cernuda, C., Kindermann, S., and Pratama,
M. (2015). Generalized smart evolving fuzzy systems.
Evolving Systems, 6(4):269–292.
Lughofer, E. and Sayed-Mouchaweh, M. (2015). Au-
tonomous data stream clustering implementing incre-
mental split-and-merge techniques — towards a plug-
and-play approach. Information Sciences, 204:54–79.
Mann, H. and Whitney, D. (1947). On a test of whether one
of two random variables is stochastically larger than
the other. Annals of mathematical Statistics, 18:50–
60.
Mukherjee, S. and Eppstein, M. J. (2012). Differential
evolution of constants in genetic programming im-
proves efficacy and bloat. In Rodriguez, K. and Blum,
C., editors, GECCO 2012 Late breaking abstracts
workshop, pages 625–626, Philadelphia, Pennsylva-
nia, USA. ACM.
Nelles, O. (2001). Nonlinear System Identification.
Springer, Berlin.
Poli, R. (2003). A simple but theoretically-motivated
method to control bloat in genetic programming. In
Genetic programming, pages 204–217. Springer.
Poli, R., Langdon, W. B., and McPhee, N. F. (2008).
A field guide to genetic programming. Published
FCTA 2016 - 8th International Conference on Fuzzy Computation Theory and Applications
62