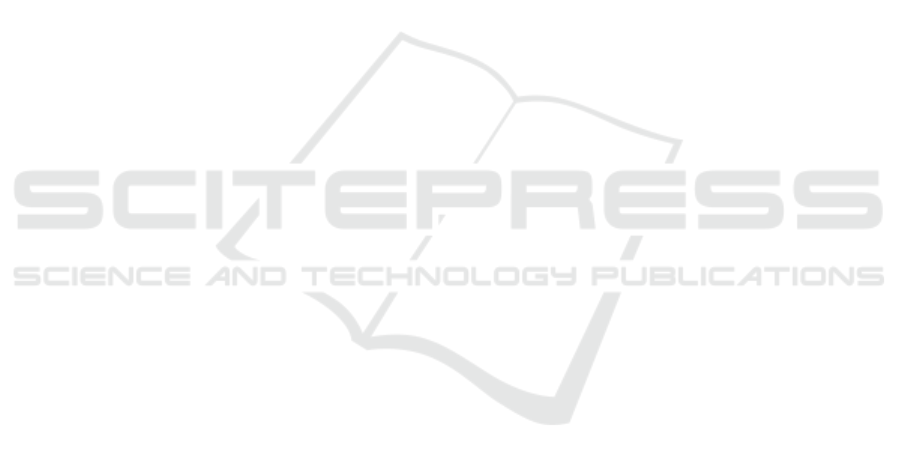
drones. Connecting to the platform will be all one
needs to do to have a professional, bespoke drone
service, customized for any necessity or location.
The platform will make it possible to immediately
find qualified solutions in the complex drone
industry, where aerial specialists coexist with
professionals coming from many different fields.
The target of the system will mainly be the owners
of remotely piloted aircraft systems of any kind,
specialised peripheral devices, and professional
services related to drones. These people could display
their offers through this shared channel. The benefits
to be gained from this would be remarkable, as an
operator could acquire new customers, interact with
colleagues and users, and increase their profits by
developing new offer plans.
Thanks to a wider, more competitive, and more
collaborative offer, aerial services operators will see
an exponential growth in opportunities, and will be
encouraged to continuously propose new solutions.
On the other hand, users could benefit from new
opportunities by saving or by forming purchasing
groups. These actors will be able to use a tool specific
to the drone domain. It is an industry with such
particular characteristics that it needs a bespoke
platform to set offers and create interactions that
would have been ineffective in general demand/offer
matching tools.
REFERENCES
Boyle, M. J. (2015). The race for drones. In Orbis, Vol. 5,
Issue 9.1, pp. 76-94.
Chen, N., Hoi, S., Li, S., Xiao, X. (2016). Mobile App
Tagging. In Proceedings of the Ninth ACM
International Conference on Web Search and Data
Mining. ACM, pp. 63-72.
Donsbach, A., Gregov, A., Few, J. D., Lehman, J. D.,
Brownell, J. T. (2012). Recommendations based on item
tagging activities of users. U.S. Patent No. 8,122,020,
Google Patents.
Floreano, D., Wood, R. J. (2015). Science, technology and
the future of small autonomous drones. In Nature, Vol.
521, Issue 7553, pp. 460-466.
Heidinger, C., Buchmann, E., Böhm, K. (2010).
Collaborative data privacy for the web. In Proceedings
of the 2010 EDBT/ICDT Workshops, ACM.
Ibba, S., Pani, F. E. (2016), The Challenge of Integrating
Instagram with a Taxonomy for Content Management.
Future Internet, 8.2: 16, 2016. DOI: 10.3390/fi8020016.
Koçak, Ö., Hannan, M. T., Hsu, G. (2013). Emergence of
market orders: Audience interaction and avanguard
influence. Organization Studies, Sage Publications.
Mathes, A. (2004). Folksonomies - Cooperative
Classification and Communication Through Shared
Metadata. In: Computer Mediated Communication –
LIS590CMC, Graduate School of Library and
Information Science, University of Illinois
Urbana-Champaign.
Mika, P. (2005). Ontologies are us: A unified model of
social networks and semantics. In International
semantic web conference, Springer Berlin Heidelberg,
pp. 522-536.
Saoud, Z., Kechid, S. (2016). Integrating social profile to
improve the source selection and the result merging
process in distributed information retrieval. In:
Information Sciences, Vol. 336, pp. 115-128.
Sarwar, B., Karypis, G., Konstan, J., & Riedl, J. (2000,
October). Analysis of recommendation algorithms for e-
commerce. In Proceedings of the 2nd ACM conference
on Electronic commerce, ACM, pp. 158-167.
Tseng, W. M., Frank, M. R., Goldstein, M. L. (2012). U.S.
Patent No. 8,103,614, Google Patents.
Unmanned Aerial Vehicle Market Profile and Forecase
(2013). Executive Summary, Teal Group.
Vander Wal, T. (2007). Folksonomy Coinage and
Definition. http://www.vanderwal.net/folksonomy.html
Xie, H., Li, X., Wang, T., Lau, R. Y. K., Wong, T., Chen,
L., Wang, F. L. Li, Q. (2016). Incorporating sentiment
into tag-based user profiles and resource profiles for
personalized search in folksonomy. In Information
Processing & Management, Vol. 52, Issue 1, pp. 61-72.
Categorization and Matching for Drone-based Services
227