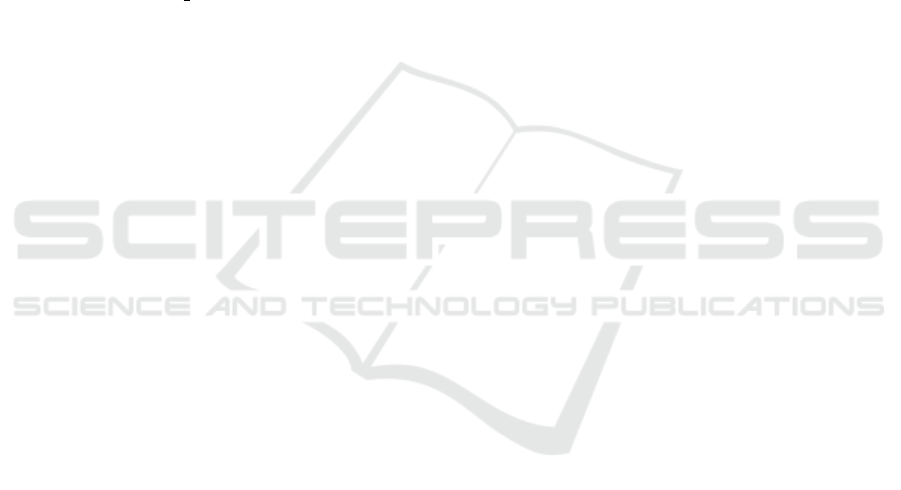
4 CONCLUSION AND FUTURE
WORK
Clustering high dimensional data is still a challeng-
ing problem for fuzzy clustering algorithms because
of the concentration of distance phenomenon. Noise
and outliers in data sets additionally make the parti-
tioning of data difficult because they affect the com-
putation of cluster centers. In this work, we analyzed
two fuzzy clustering algorithms for high dimensional
data from the literature and two possibilistic versions
of the MFCM algorithm in terms of correct determin-
ing final cluster prototypes in presence of noise. Our
experiments showed that MFCM produced the most
accurate cluster centers as long as data items had real
values in few features while its possibilistic versions
PMFCM and PMFCM HDD produced quite accurate
final cluster centers independently from the number
of features in that noise points had real values.
Although the performance results for PMFCM
seem to be promising, before applying this method
on real data sets, we plan to analyze the performance
of fuzzy clustering algorithms in terms of sensitivity
to different initializations because usually we do not
have any a priori knowledge about the distribution of
data in practical applications. In our future work, we
also plan to apply other possibilistic clustering models
to MFCM to make it less sensitive to outliers. Further-
more, we aim to apply fuzzy clustering algorithms for
clustering text and image data and compare their per-
formance with common crisp clustering algorithms.
REFERENCES
Beyer, K. S., Goldstein, J., Ramakrishnan, R., and Shaft,
U. (1999). When is ”nearest neighbor” meaningful?
In Proceedings of the 7th International Conference on
Database Theory, ICDT ’99, pages 217–235, London,
UK, UK. Springer-Verlag.
Bezdek, J. C. (1981). Pattern Recognition with Fuzzy Ob-
jective Function Algorithms. Kluwer Academic Pub-
lishers, Norwell, MA, USA.
Dave, R. N. and Krishnapuram, R. (1997). Robust cluster-
ing methods: A unified view. IEEE Transactions on
Fuzzy Systems, 5(2):270–293.
Dunn, J. C. (1973). A fuzzy relative of the isodata process
and its use in detecting compact well-separated clus-
ters. Journal of Cybernetics, 3(3):32–57.
Himmelspach, L. and Conrad, S. (2016). A possibilistic
multivariate fuzzy c-means clustering algorithm. In
Proceedings of the 10th International Conference on
Scalable Uncertainty Management, SUM 2016, pages
338–344.
Keller, A. and Klawonn, F. (2000). Fuzzy clustering with
weighting of data variables. International Journal
of Uncertainty, Fuzziness and Knowledge-Based Sys-
tems, 8(6):735–746.
Klawonn, F. (2013). What can fuzzy cluster analysis con-
tribute to clustering of high-dimensional data? In
Proceedings of the 10th International Workshop on
Fuzzy Logic and Applications, WILF2013, Genoa,
Italy, November 19–22, 2013., pages 1–14.
Klawonn, F., Kruse, R., and Winkler, R. (2015). Fuzzy clus-
tering: More than just fuzzification. Fuzzy Sets and
Systems, 281:272–279.
Kriegel, H., Kr
¨
oger, P., Schubert, E., and Zimek, A. (2009).
Outlier detection in axis-parallel subspaces of high di-
mensional data. In Proceedings of the 13th Pacific-
Asia Conference on Advances in Knowledge Discov-
ery and Data Mining, PAKDD 2009, pages 831–838.
Krishnapuram, R. and Keller, J. M. (1993). A possibilistic
approach to clustering. IEEE Transactions on Fuzzy
Systems, 1(2):98–110.
MacQueen, J. (1967). Some methods for classification and
analysis of multivariate observations. In Proceed-
ings of the Fifth Berkeley Symposium on Mathematical
Statistics and Probability, Volume 1: Statistics, pages
281–297, Berkeley. University of California Press.
Pal, N. R., Pal, K., Keller, J. M., and Bezdek, J. C. (2005).
A possibilistic fuzzy c-means clustering algorithm.
IEEE Transactions on Fuzzy Systems, 13(4):517–530.
Pimentel, B. A. and de Souza, R. M. C. R. (2013). A multi-
variate fuzzy c-means method. Applied Soft Comput-
ing, 13(4):1592–1607.
Rehm, F., Klawonn, F., and Kruse, R. (2007). A novel ap-
proach to noise clustering for outlier detection. Soft
Computing, 11(5):489–494.
Winkler, R., Klawonn, F., and Kruse, R. (2011). Fuzzy c-
means in high dimensional spaces. International Jour-
nal of Fuzzy System Applications, 1(1):1–16.
FCTA 2016 - 8th International Conference on Fuzzy Computation Theory and Applications
108