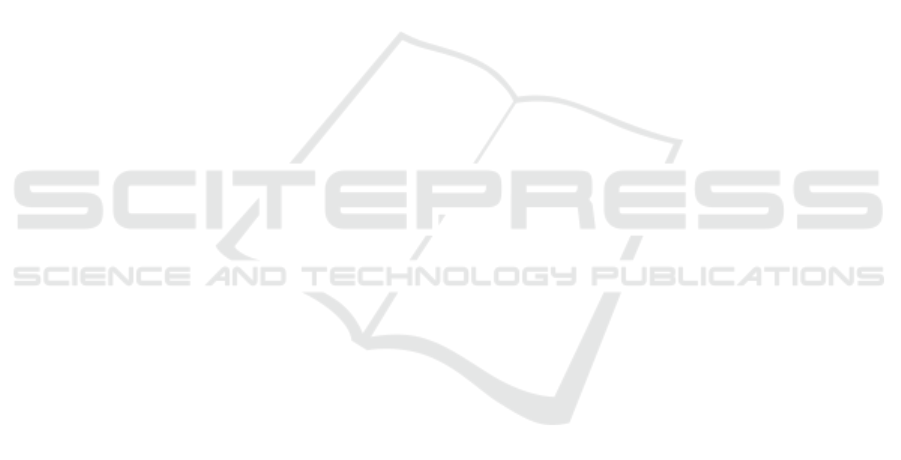
Figure 6 shows very small rises for three SM’s
keyphrases (“social networking”, “internet users,
and “social media users”). Only two keyphrases
“social media” and “social networks” show
relatively nice rises. It is interesting to point that
four out of the five keyphrases include the word
“social”, which is also the dominate word included
in the domain name. The relatively low values of all
the five keyphrases are compatible with the low
scores of this domain in Figure 1.
6 SUMMARY AND FUTURE
WORK
In this paper, we present a methodology (including a
detailed algorithm, various development measures,
and suitable stopword lists) for measuring the
development of domains and keyphrases. The
experimental results suggest that development trends
of domains and keyphrases can be efficiently
measured using measure3.
The main findings are: (1) The investigation of
the five NLP sub-domains found that three domains:
LR, SA&OM, and especially MT are much more
popular especially over the last years, while DH and
SM are significantly less explored; (2) Top bigrams
and trigram(s) are enough to identify general trends
in NLP domains while unigrams are noisy and
therefore were avoided; and (3) As expected the
name of the domain was one of the top keyphrases
in each one of the tested domain.
Future research proposals are: (1) Use extended
definitions of keyphrases (not only bigrams and
trigrams) and apply more sophisticated methods to
automatically learn and extract keyphrases (e.g.,
HaCohen-Kerner et al, 2005; HaCohen-Kerner et al,
2007); (2) Apply additional keyphrases’ measures,
which are more complex and informative such as
PWI “probability-weighted amount of information’’
and TF-IDF ‘‘Term frequency–inverse document
frequency’’; (3) Perform additional experiments on
other kinds of intervals of years (e.g., every one
year, every five years); (4) Apply this development
model to other types of NLP domains and
conferences as well as to other domains in other
fields; and (5) Investigation of additional concepts
regarding development of domains and concepts
such as merge of two concepts (domains) to one
concept (domain), and split of one concept (domain)
to several concepts (domains).
REFERENCES
Anderson, A., McFarland, D., Jurafsky, D., 2012. Towards
a computational history of the ACL: 1980-2008. In
Proceedings of the ACL-2012 Special Workshop on
Rediscovering 50 Years of Discoveries (pp. 13-21).
Association for Computational Linguistics.
Blei, D. M., Ng, A. Y., Jordan, M. I., 2003. Latent
dirichlet allocation. The Journal of machine Learning
research, 3, 993-1022.
Daudaravičius, V., 2012. Applying collocation
segmentation to the ACL Anthology Reference
Corpus. In Proceedings of the ACL-2012 Special
Workshop on Rediscovering 50 Years of Discoveries
(pp. 66-75). Association for Computational
Linguistics.
Dietz, L., Bickel, S., Scheffer, T., 2007. Unsupervised
prediction of citation influences. In Proc. of ICML.
Garfield, E. 1965. Can citation indexing be automated? In
Statistical association methods for mechanical
documentation, Symposium Proceedings, Washington
edited by M. Stevens. (National Bureau of Standards,
Miscellaneous Publication 269, Dec 1964, 15, 1965).
Gerrish, S., Blei, D. M., 2010. A language-based approach
to measuring scholarly impact. In Proc. of ICML.
Griffiths, T. L, Steyvers. M., 2004. Finding scientific
topics. Proc. of the National Academy of Sciences of
the United States of America, 101(Suppl 1):5228.
HaCohen-Kerner, Y., Gross, Z., Masa, A., 2005.
Automatic extraction and learning of keyphrases from
scientific articles. In Proc. of CICLing (pp. 657-669).
Springer Berlin Heidelberg.
HaCohen-Kerner, Y., Stern, I., Korkus, D., Fredj, E.,
2007. Automatic machine learning of keyphrase
extraction from short html documents written in
Hebrew. Cybernetics and Systems: An International
Journal, 38(1), 1-21.
Hall, D., Jurafsky, D., Manning, C. D., 2008. Studying the
history of ideas using topic models. In Proc. of
EMNLP.
Mann, G. S., Mimno. D., McCallum, A., 2006.
Bibliometric impact measures leveraging topic
analysis. In Proc. of the 6
th
ACM/IEEE-CS joint
conference on Digital libraries. ACM, 2006. p. 65-74.
Omodei, E., Guo, Y., Cointet, J. P., Poibeau, T., 2014A.
Social and semantic diversity: Socio-semantic
representation of a scientific corpus. EACL 2014, 71.
Omodei, E., Cointet, J-P., Poibeau, T., 2014B. Mapping
the natural language processing domain: experiments
using the ACL anthology. LREC 2014, the Ninth
International Conference on Language Resources and
Evaluation, May 2014, Reykjavik, Iceland. ELRA, pp.
2972-2979.
Radev, D., Abu-Jbara, A., 2012. Rediscovering ACL
discoveries through the lens of ACL anthology
network citing sentences. In Proc. of the ACL-2012
Special Workshop on Rediscovering 50 Years of
Discoveries (pp. 1-12). Association for Computational
Linguistics.
KDIR 2016 - 8th International Conference on Knowledge Discovery and Information Retrieval
382