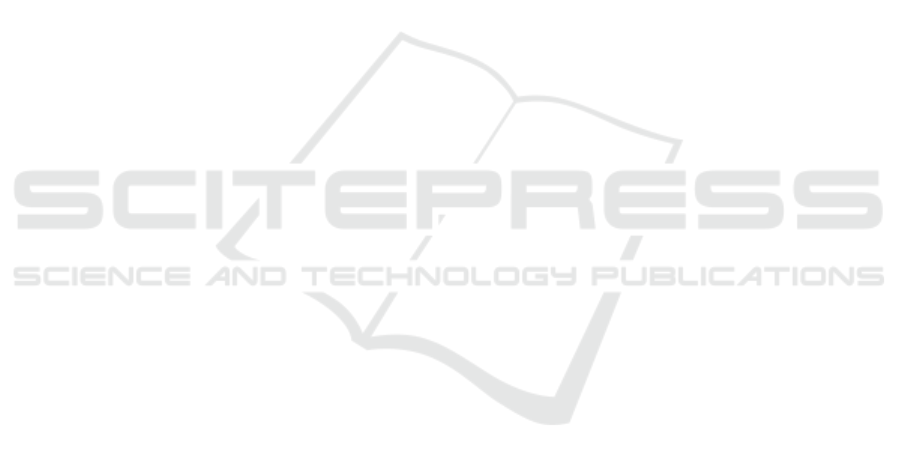
until the end and the total race times improved for all
subjects compared to their own paced rides.
To answer the third question the subjects per-
formed ride IV. The feedback in ride IV implied a
power output constantly above the power output of
ride II. Since ride II was until exhaustion, it should
have not been possible to maintain the proposed
power output until the end of the ride.
Three out of six subjects confirmed this assump-
tion. They were not able to follow the feedback given
in ride IV in the last part of the race. Nevertheless the
other three subjects were able to maintain the strategy
until the end. This indicates that the feedback itself
motivated them to access more energy resources than
in their self paced ride.
Therefore question three cannot be answered
clearly. Feedback alone enabled most subjects to im-
prove their race times, even if they were not able to
follow it until the end. But the three subjects that
could not follow the feedback in ride IV until the end
clearly showed that there is a definite advantage using
the optimal strategy.
In order to answer the third question satisfacto-
rily and distinguish between improvements due to the
strategy and improvements due to the pace maker, a
larger set of participants would be needed to be able
to apply statistical methods and provide an adequate
quantitative justification.
5 CONCLUSIONS
Our experiment showed that the calculated optimal
strategy is feasible in a way that all athletes were able
to follow it until the end. Furthermore, it provides an
advantage over the strategy the athletes chose on their
own.
Even though external feedback itself already en-
abled most subjects to improve their performance, a
well chosen strategy like the calculated optimal strat-
egy is required to ensure that the athlete can finish the
race properly and enhance the total race time.
The next step to get closer to real racing conditions
is to perform a similar experiment in the field. There-
fore a feedback device has to be developed, which
incorporates a pace maker based on GPS measure-
ments.
Another major challenge arising with field tests is
to consider wind conditions along the track and to
provide a corresponding real-time adaptation of the
optimal strategy.
REFERENCES
Dahmen, T. and Brosda, F. (2016). Robust computation
of minimum-time pacing strategies on realistic road
cycling tracks. In Sportinformatik 2016, 11. Sym-
posium der dvs-Sektion Sportinformatik, Magdeburg,
Germany. accepted.
Dahmen, T., Byshko, R., Saupe, D., R
¨
oder, M., and
Mantler, S. (2011). Validation of a model and a simu-
lator for road cycling on real tracks. Sports Engineer-
ing, 14(2-4):95–110.
Dahmen, T., Wolf, S., and Saupe, D. (2012). Applications
of mathematical models of road cycling. IFAC Pro-
ceedings Volumes, 45(2):804–809.
Gordon, S. (2005). Optimising distribution of power during
a cycling time trial. Sports Engineering, 8(2):81–90.
Martin, J. C., Milliken, D. L., Cobb, J. E., McFadden, K. L.,
and Coggan, A. R. (1998). Validation of a mathemat-
ical model for road cycling power. Journal of applied
biomechanics, 14:276–291.
Monod, H. and Scherrer, J. (1965). The work capacity of a
synergic muscular group. Ergonomics, 8(3):329–338.
Morton, R. H. (1986). A three component model of hu-
man bioenergetics. Journal of mathematical biology,
24(4):451–466.
Morton, R. H. (1996). A 3-parameter critical power model.
Ergonomics, 39(4):611–619.
Patterson, M. A. and Rao, A. V. (2014). Gpops-ii: A mat-
lab software for solving multiple-phase optimal con-
trol problems using hp-adaptive gaussian quadrature
collocation methods and sparse nonlinear program-
ming. ACM Transactions on Mathematical Software
(TOMS), 41(1):1.
Sundstr
¨
om, D., Carlsson, P., and Tinnsten, M. (2014). Com-
paring bioenergetic models for the optimisation of
pacing strategy in road cycling. Sports Engineering,
17(4):207–215.
icSPORTS 2016 - 4th International Congress on Sport Sciences Research and Technology Support
114