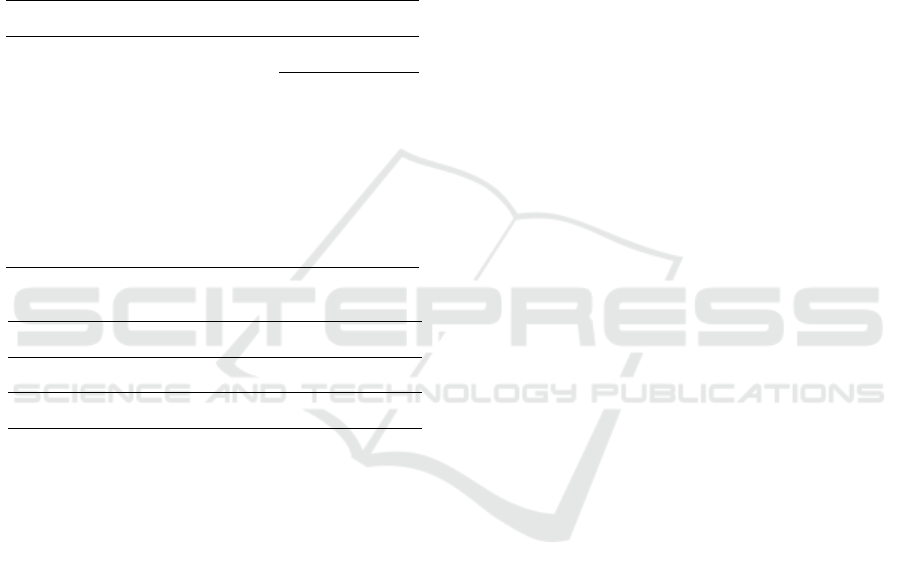
rules generated by the labeled fuzzy rough set (LFRS)
and the fuzzy flow graph (FFG) approaches. Two ad-
ditional rules R
7
and R
8
, obtained with the fuzzy flow
graph approach, are denoted by ´ in column LFRS.
As we can see, the rules R
7
and R
8
are composed of
such linguistic values that do not form linguistic la-
bels determined with the labeled fuzzy rough set ap-
proach. On the other hand, the decision rule R
6
has a
low value of strength. This rule would be discarded,
when we set a slightly higher threshold of the strength
of rules.
Table 4: Decision rules.
Decision rule LFRS FFG
strength [%] cer
R
1
: pC
11
, C
21
, C
31
q Ñ pD
11
q ` 6.94 0.92
R
2
: pC
11
, C
21
, C
32
q Ñ pD
11
q ` 10.76 0.88
R
3
: pC
12
, C
22
, C
31
q Ñ pD
12
q ` 11.52 0.85
R
4
: pC
13
, C
23
, C
33
q Ñ pD
13
q ` 17.31 0.92
R
5
: pC
12
, C
22
, C
32
q Ñ pD
13
q ` 6.55 0.79
R
6
: pC
13
, C
21
, C
32
q Ñ pD
13
q ` 1.73 0.75
R
7
: pC
11
, C
22
, C
31
q Ñ pD
12
q ´ 4.14 0.70
R
8
: pC
13
, C
23
, C
33
q Ñ pD
13
q ´ 3.63 0.89
Table 5: Approximation quality of the information system.
Removed attribute
none c
1
c
2
c
3
0.75 0.64 0.77 0.56
4 CONCLUSIONS
Both the labeled fuzzy rough set approach and the
fuzzy flow graph method generate comparable results.
Due to a new way of determination of fuzzy similar-
ity classes, which helps to reduce the computational
complexity of the standard fuzzy rough set algorithm,
the labeled fuzzy rough set approach is a preferable
method. It is also less computationally demanding in
comparison with the fuzzy flow graph approach. By
acting like a human expert, who takes into account
only the most significant features of the observed phe-
nomenon, a system of fuzzy decision rules can be
easy obtained. The presented example of analysis
of a simple information system with fuzzy attributes
confirms the effectiveness of the developed method.
Nevertheless, due to a detailed analysis of informa-
tion system with a fuzzy flow graph representation,
all possible decision rules can be taken into consid-
eration, which can help to refine the fuzzy inference
system. However, selecting suitable threshold values
for the factors of certainty and strength of rules can
be problematic. In future work, another variants of
parameterized fuzzy rough set approach will be in-
vestigated.
REFERENCES
Dubois, D. and Prade, H. (1992). Putting rough sets and
fuzzy sets together. In Słowi
´
nski, R., editor, Intel-
ligent Decision Support: Handbook of Applications
and Advances of the Rough Sets Theory, pages 203–
232, Boston Dordrecht London. Kluwer Academic
Publishers.
Mieszkowicz-Rolka, A. and Rolka, L. (2004). Fuzzy im-
plication operators in variable precision fuzzy rough
sets model. In Rutkowski, L. et al., editors, Ar-
tificial Intelligence and Soft Computing — ICAISC
2004. Lecture Notes in Artificial Intelligence, volume
3070, pages 498–503, Berlin Heidelberg New York.
Springer-Verlag.
Mieszkowicz-Rolka, A. and Rolka, L. (2006). Flow
graphs and decision tables with fuzzy attributes. In
Rutkowski, L. et al., editors, Artificial Intelligence and
Soft Computing — ICAISC 2006. Lecture Notes in
Artificial Intelligence, volume 4029, pages 268–277,
Berlin Heidelberg New York. Springer-Verlag.
Mieszkowicz-Rolka, A. and Rolka, L. (2014). Flow graph
approach for studying fuzzy inference systems. Pro-
cedia Computer Science, 35:681–690. sciencedi-
rect.com/science/journal/18770509/35.
Mieszkowicz-Rolka, A. and Rolka, L. (2016). A novel
approach to fuzzy rough set-based analysis of infor-
mation systems. In
´
Swi ˛atek, J. et al., editors, Infor-
mation Systems Architecture and Technology. Knowl-
edge Based Approach to the Design, Control and De-
cision Support, volume 432 of Advances in Intelligent
Systems and Computing, pages 173–183, Switzerland.
Springer International Publishing.
Pawlak, Z. (1991). Rough Sets: Theoretical Aspects of
Reasoning about Data. Kluwer Academic Publishers,
Boston Dordrecht London.
Pawlak, Z. (2005a). Flow graphs and data mining. In Pe-
ters, J. F. et al., editors, Transactions on Rough Sets
III. Lecture Notes in Computer Science (Journal Sub-
line), volume 3400, pages 1–36, Berlin Heidelberg
New York. Springer-Verlag.
Pawlak, Z. (2005b). Rough sets and flow graphs. In
´
Sl˛ezak,
D. et al., editors, Rough Sets, Fuzzy Sets, Data Mining,
and Granular Computing. Lecture Notes in Artificial
Intelligence, volume 3641, pages 1–11, Berlin Heidel-
berg New York. Springer-Verlag.
Radzikowska, A. M. and Kerre, E. E. (2002). A comparative
study of fuzzy rough sets. Fuzzy Sets and Systems,
126:137–155.
Zadeh, L. (1965). Fuzzy sets. Information and Control,
8:338–353.
FCTA 2016 - 8th International Conference on Fuzzy Computation Theory and Applications
120