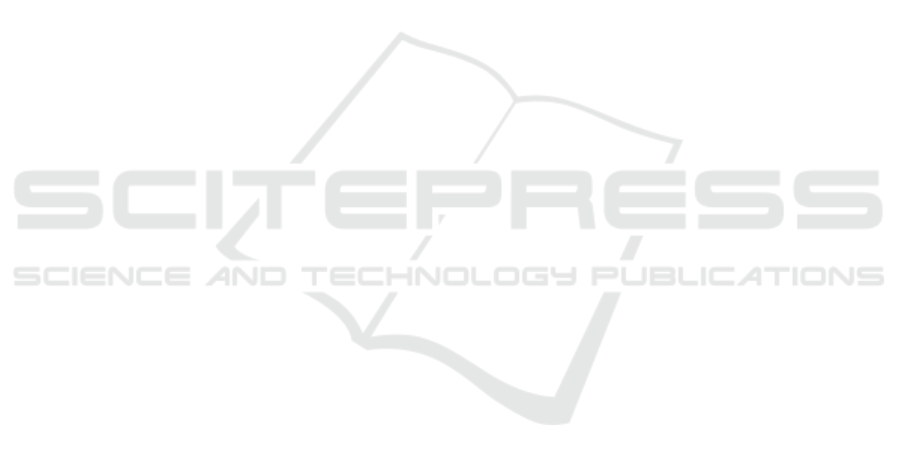
7 CONCLUSIONS AND FUTURE
WORK
In this paper we introduced an algorithm based on
contextual conditional preferences for rating predic-
tion tasks in cold-start situations. For our experiments
we used three configurations of the model: only indi-
vidual preferences, only general preferences and both
types of preferences. We performed tests on three
publicly available data sets and compared obtained re-
sults with those generated with several state-of-the-art
baselines. We showed that proposed approach works
at least as good as these baselines according to the
prediction accuracy measured with MAE and RMSE
for all data sets and configurations with one exception
for individual contextual conditional preferences and
Restaurant & consumer data set. However, this re-
sult is interesting. It showed that a user information
like drink level or dress preference, is not enough to
compute reasonable individual contextual conditional
preferences. Nevertheless, proposed algorithm with
general contextual conditional preferences decreased
a prediction error in comparison with baselines. It
could lead us to conclusion that the method is more
general and could be used also for a quasi-contextual
data.
The next steps that need to be taken are: (I) an
automation of a feature selection by using deep learn-
ing techniques, (II) testing our approach in the con-
tinuous cold start situations, (III) an adaptation of the
proposed method for a ranking task, and (IV) a com-
parison with other cold-start methods. Nevertheless,
preliminary results look promising.
REFERENCES
Adomavicius, G. and Tuzhilin, A. (2011). Context-aware
recommender systems. In Ricci, F., Rokach, L.,
Shapira, B., and Kantor, P. B., editors, Handbook on
Recommender Systems, pages 217–256. Springer.
Baltrunas, L. and Amatriain, X. (2009). Towards time-
dependant recommendation based on implicit feed-
back. In Proceedings of 1st Workshop on Context-
Aware Recommender Systems.
Bernardi, L., Kamps, J., Kiseleva, J., and Mller, M. J. I.
(2015). The continuous cold-start problem in e-
commerce recommender systems. In Bogers, T.
and Koolen, M., editors, CBRecSys@RecSys, volume
1448 of CEUR Workshop Proceedings, pages 30–33.
CEUR-WS.org.
Boutilier, C., Brafman, R. I., Domshlak, C., Hoos, H. H.,
and Poole, D. (2004). Cp-nets: A tool for representing
and reasoning with conditional ceteris paribus prefer-
ence statements. Journal of Artificial Intelligence Re-
search, 21:135–191.
Braunhofer, M., Elahi, M., Ricci, F., and Schievenin, T.
(2013). Context-aware points of interest suggestion
with dynamic weather data management. In Xiang, Z.
and Tussyadiah, I., editors, Information and Commu-
nication Technologies in Tourism 2014, pages 87–100.
Springer International Publishing.
Costa, H., Furtado, B., Pires, D., Macedo, L., and Cardoso,
A. (2012). Context and intention-awareness in pois
recommender systems. In Proceedings of 4th Work-
shop on Context-Aware Recommender Systems.
de Macedo, A. Q., Marinho, L. B., and Santos, R. L. T.
(2015). Context-aware event recommendation in
event-based social networks. In Werthner, H., Zanker,
M., Golbeck, J., and Semeraro, G., editors, RecSys,
pages 123–130. ACM.
Jannach, D., Zanker, M., Felfernig, A., and Friedrich, G.
(2010). Recommender Systems: An Introduction.
Cambridge University Press, New York, NY, USA, 1st
edition.
Karpus, A., di Noia, T., Tomeo, P., and Goczyła, K. (2016).
Using contextual conditional preferences for recom-
mendation tasks: a case study in the movie domain.
Studia Informatica, 37(1):7–18.
Kosir, A., Odic, A., Kunaver, M., Tkalcic, M., and Tasic,
J. F. (2011). Database for contextual personalization.
Elektrotehniski vestnik [English print ed.], 78(5):270–
274.
Kula, M. (2015). Metadata embeddings for user and
item cold-start recommendations. In Bogers, T.
and Koolen, M., editors, CBRecSys@RecSys, volume
1448 of CEUR Workshop Proceedings, pages 14–21.
CEUR-WS.org.
Lee, J. S. and Lee, J. C. (2007). Context awareness by case-
based reasoning in a music recommendation system.
In Proceedings of the 4th International Conference on
Ubiquitous Computing Systems, UCS’07, pages 45–
58, Berlin, Heidelberg. Springer-Verlag.
Lombardi, S., Anand, S. S., and Gorgoglione, M. (2009).
Context and customer behavior in recommendation.
In Proceedings of 1st Workshop on Context-Aware
Recommender Systems.
Odic, A., Tkalcic, M., Tasic, J. F., and Kosir, A. (2013).
Predicting and detecting the relevant contextual infor-
mation in a movie-recommender system. Interacting
with Computers, 25(1):74–90.
Satzger, B., Endres, M., and Kießling, W. (2006). A
Preference-Based Recommender System, pages 31–
40. Springer Berlin Heidelberg, Berlin, Heidelberg.
Stefanidis, K., Pitoura, E., and Vassiliadis, P. (2011). Man-
aging contextual preferences. in Info. Sys, pages
1158–1180.
Vargas-Govea, B., Gonzalez-Serna, G., and Ponce-
Medellin, R. (2011). Effects of relevant contextual
features in the performance of a restaurant recom-
mender system. In Proceedings of 3rd Workshop on
Context-Aware Recommender Systems.
KDIR 2016 - 8th International Conference on Knowledge Discovery and Information Retrieval
424