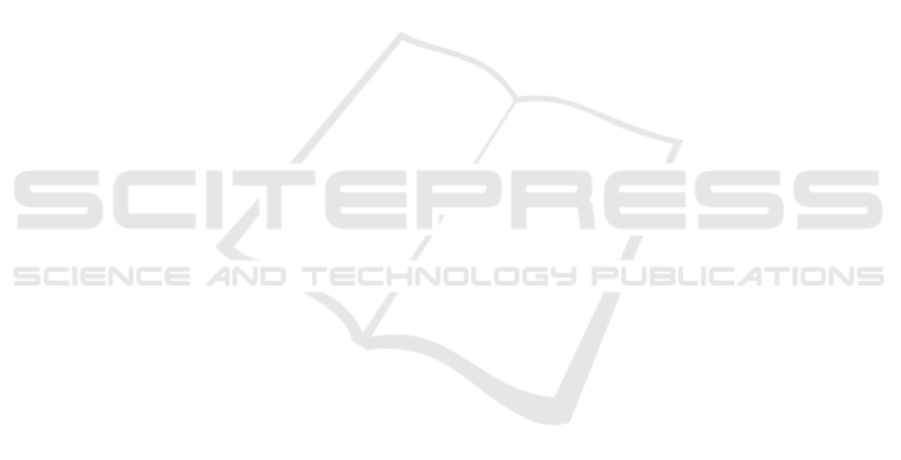
Enhancement on the predictions of our model arises
from selecting proper models to combine and the way
of combination. We select three distinctive models,
and combine them by using stacked generalization.
There are several extensions that we plan to study.
First, designed ensemble model can be extended by
the other learning models or the other ways to com-
bine models. As stated, these selections play a key
role in ensemble models. Besides that, after overcom-
ing the matching problems of drugs or cell lines aris-
ing from varied denomination, model can be trained
by using more balanced data sets for each drug by
unifying different data sets. Furthermore, used fea-
tures are important for models. For drug response pre-
diction, traditional way is using gene expression data
but features can be extended via integration of drugs’
chemical information and other genomic features.
ACKNOWLEDGEMENTS
This study is supported by The Scientific and Tech-
nological Research Council of Turkey, Grant no:
115E274.
REFERENCES
Bansal, M., Yang, J., et al. (2014). A community computa-
tional challenge to predict the activity of pairs of com-
pounds. Nature Biotechnology, 32(2):1–3.
Brem, G. J., Mylonas, I., et al. (2013). Eeyarestatin causes
cervical cancer cell sensitization to bortezomib treat-
ment by augmenting ER stress and CHOP expression.
Gynecologic Oncology, 128:383–390.
Caruana, R. (1998). Multitask Learning. In Learning to
Learn, pages 95–133. Springer US, Boston, MA.
Cort
´
es-Ciriano, I., van Westen, G. J. P., et al. (2015). Im-
proved large-scale prediction of growth inhibition pat-
terns using the NCI60 cancer cell line panel. Bioinfor-
matics, 32(1):btv529.
Costello, J. C., Heiser, L. M., et al. (2014). A community
effort to assess and improve drug sensitivity prediction
algorithms. Nature Biotechnology, 32(12):1202.
Cukier, H., Peralta, R., et al. (2012). Preclinical dose
scheduling studies of lor-253, a novel anticancer drug,
in combination with chemotherapeutics in lung and
colon cancers. In AACR; Cancer Res, volume 72.
Dong, Z., Zhang, N., et al. (2015). Anticancer drug sensitiv-
ity prediction in cell lines from baseline gene expres-
sion through recursive feature selection. BMC cancer,
15(1):489.
Friedman, H. (2002). Stochastic gradient boosting. Compu-
tational Statistics and Data Analysis, 38(4):367–378.
Gonen, M. and Margolin, A. A. (2014). Drug suscepti-
bility prediction against a panel of drugs using ker-
nelized Bayesian multitask learning. Bioinformatics,
30(17):i556–i563.
Haider, S., Rahman, R., et al. (2015). A Copula
Based Approach for Design of Multivariate Random
Forests for Drug Sensitivity Prediction. PLOS ONE,
10(12):e0144490.
Jackson, S. E. and Chester, J. D. (2015). Personalised
cancer medicine. International Journal of Cancer,
137(2):262–266.
Ji, S. and Ye, J. (2009). An accelerated gradient method for
trace norm minimization. In Proceedings of the 26th
annual ICML, pages 457–464. ACM.
Menden, M. P., Iorio, F., et al. (2013). Machine Learning
Prediction of Cancer Cell Sensitivity to Drugs Based
on Genomic and Chemical Properties. PLoS ONE,
8(4):e61318.
Qin, Y., Chen, M., et al. (2015). A network flow-based
method to predict anticancer drug sensitivity. PLoS
ONE, 10(5):1–14.
Rappaport, N., Nativ, N., et al. (2013). MalaCards: an inte-
grated compendium for diseases and their annotation.
Database : the journal of biological databases and
curation, 2013:bat018.
Rees, M. G., Seashore-Ludlow, B., et al. (2015). Correlat-
ing chemical sensitivity and basal gene expression re-
veals mechanism of action. Nature Chemical Biology,
12(2):109–116.
Riddick, G., Song, H., et al. (2011). Predicting in vitro drug
sensitivity using Random Forests. 27(2):220–22410.
Seashore-Ludlow, B., Rees, M. G., et al. (2015). Harnessing
Connectivity in a Large-Scale Small-Molecule Sensi-
tivity Dataset. Cancer discovery, 5(11):1210–23.
Sewell, M. (2008). Ensemble learning. RN, 11(02).
Shi, L., Song, X.-B., et al. (2016). Docetaxel-conjugated
monomethoxy-poly(ethylene glycol)-b-poly(lactide)
(mPEG-PLA) polymeric micelles to enhance the ther-
apeutic efficacy in oral squamous cell carcinoma. RSC
Adv., 6(49):42819–42826.
Wolpert, D. H. (1992). Stacked generalization. Neural Net-
works, 5(2):241–259.
Yang, W., Soares, J., et al. (2013). Genomics of Drug Sen-
sitivity in Cancer (GDSC): a resource for therapeutic
biomarker discovery in cancer cells. Nucleic Acids
Research, 41(D1):D955–D961.
Zhang, N., Wang, H., et al. (2015). Predicting Anticancer
Drug Responses Using a Dual-Layer Integrated Cell
Line-Drug Network Model. PLoS computational bi-
ology, 11(9):e1004498.
Zhao, Z., Fu, G., et al. (2013). Drug activity predic-
tion using multiple-instance learning via joint instance
and feature selection. BMC Bioinformatics, 14 Suppl
1(Suppl 14):S16.
Zhou, J., Chen, J., and Ye, J. (2012). User’s Manual MAL-
SAR: Multi-tAsk Learning via StructurAl Regulariza-
tion. Arizona State University.
KDIR 2016 - 8th International Conference on Knowledge Discovery and Information Retrieval
436