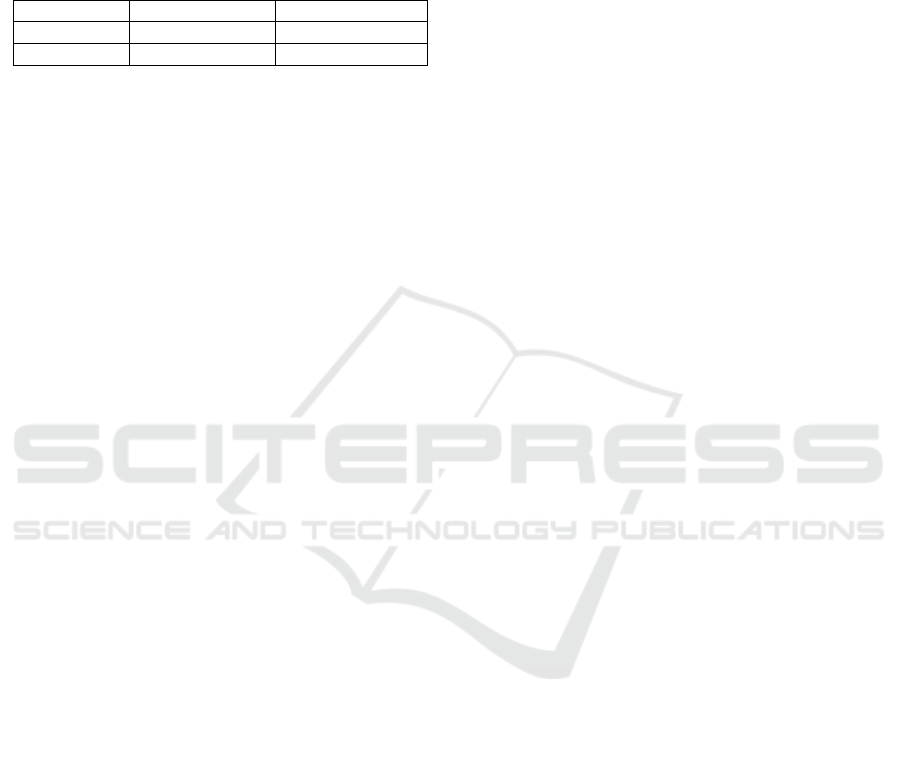
In terms of performance, we calculated the RMS
and NARE for each of the two scenarios. As we can
see in table 1, the performance of the HMM is
reasonably good with NARE values in the range of
0.20 – 0.26 and RMS from 0.58 – 0.88.
Table 2: RMS and NARE of the two scenarios.
Subsequently, we see that the events identification
does not show any peaks to indicate that there is a
state that has not been found. The above show us that
we may have a reasonable mechanism that will be
able to classify and predict traffic in a wireless
network.
7 CONCLUSIONS
In this paper, we addressed the classification and
prediction of wireless traffic using HMMs. We
employed two clustering techniques, in order to
clarify the states of the data to be input in the HMM.
The first one was the IBS index, which is usually
used in physiological signals. We performed three
experiments, obtaining the distances between three
types of network traffic, namely No Network, Full
Network and Bursty Traffic. We have seen that we
get a difference in two of the three experiments; thus,
resulting in a clear threshold identification for the
identification of different traffic states. However, two
of the three traffic classes exhibit a very similar
distance; hence, the recognition of a new state by the
HMM will be ambiguous. Furthermore, the nature of
the IBS require bunches of signal values to be
examined in order to locate the distance of the data to
be evaluated.
Hence, we decided to use the Euclidean distance,
which allows us to get a distance between the current
state and the incoming value at a single value level;
thus, identifying at a greater granularity the traffic
patterns.
We put our approach to the test using two traffic
files, one of the showing bursty traffic and a second
with no connection. The HMM was able to locate the
traffic patterns and identify the right number of states
and events. We believe that this is an efficient
approach for traffic pattern classification and
prediction.
For future work, we aim to put our approach to a
real network implementation, in order to obtain useful
information regarding the operation of the HMM to
low-power energy constraint devices.
REFERENCES
Alizai, M. H., Landsiedel, O., Link, J. A´ . B., Go¨tz, S.,and
Wehrle, K. (2009). Bursty traffic over bursty links. In
Proceedings of the 7th ACM Conference on Embedded
Networked Sensor Systems, pages 71–84. ACM.
Beran, J. (1994). Statistics for long-memory processes,
Volume 61. CRC press.
Dunham, M. H., Meng, Y., and Huang, J. (2004).Extensible
markov model. In Data Mining, 2004. ICDM’04. Fourth
IEEE International Conference on, pages 371– 374.
IEEE.
Eddy, S. R. (1996). Hidden markov models. Current
opinion in structural biology, 6(3):361–365.
Jiang, H. and Dovrolis, C. (2005). Why is the internettraffic
bursty in short time scales? In ACM SIGMETRICS
Performance Evaluation Review, volume 33, pages
241–252. ACM. Jiang, M.,
Nikolic, M., Hardy, S., and Trajkovic, L. (2001).Impact of
self-similarity on wireless data network performance.
In Communications, 2001. ICC 2001. IEEE
International Conference on, volume 2, pages 477–
481. IEEE.
Kosko, B. (1992). Neural networks and fuzzy systems: a
dynamical systems approach to machine intelligence/
book and disk. Prentice Hall, Upper Saddle River.
Li, R., Zhao, Z., Zhou, X., Palicot, J., and Zhang, H. (2014).
The prediction analysis of cellular radio access
network traffic: From entropy theory to networking
practice. IEEE Communications Magazine, 52(6):234–
240.
Loumiotis, I., Adamopoulou, E., Demestichas,
K.,Kosmides, P., and Theologou, M. (2014). Artificial
neural networks for traffic prediction in 4g networks. In
International Wireless Internet Conference, pages 141–
146. Springer.
Maheshwari, S., Mahapatra, S., Kumar, C. S., and Vasu,K.
(2013). A joint parametric prediction model for
wireless internet traffic using hidden markov model.
Wireless networks, 19(6):1171–1185.
MacQueen, J. (1967, June). Some methods for
classification and analysis of multivariate observations.
In Proceedings of the fifth Berkeley symposium on
mathematical statistics and probability (Vol. 1, No. 14,
pp. 281-297).
Ni, F., Zang, Y., and Feng, Z. (2015). A study on cellular
wireless traffic modeling and prediction using elman
neural networks. In 2015 4th International Conference
on Computer Science and Network Technology
(ICCSNT), volume 1, pages 490–494. IEEE.
Pan, T., Sumalee, A., Zhong, R.-X., and Indra-Payoong,N.
(2013). Short-term traffic state prediction based on
temporal–spatial correlation. IEEE Transactions on
Intelligent Transportation Systems, 14(3):1242–1254.
Papadopouli, M., Shen, H., Raftopoulos, E., Ploumidis,M.,
and Hernandez-Campos, F. (2005). Short-term traffic
forecasting in a campus-wide wireless network. In 2005
IEEE 16th International Symposium on Personal,
Indoor and Mobile Radio Communications, volume 3,
pages 1446–1452. IEEE.
Fifth International Conference on Telecommunications and Remote Sensing