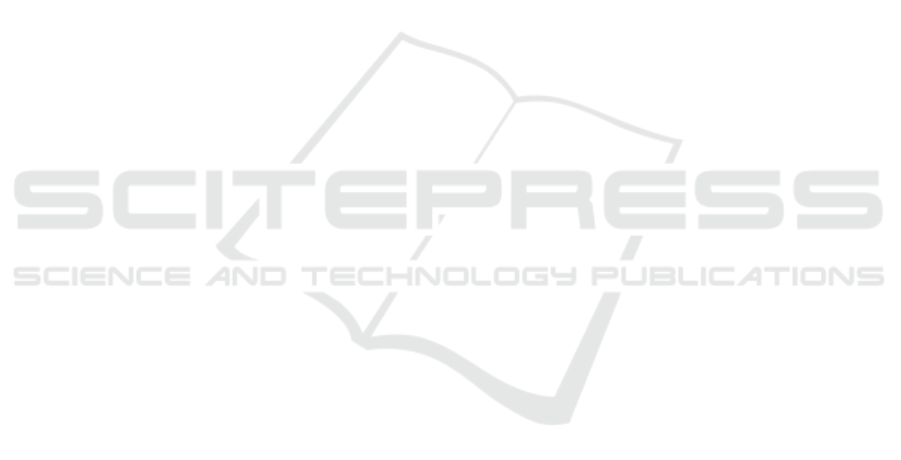
Dalal, N. and Triggs, B. (2005). Histograms of oriented
gradients for human detection. In CVPR.
Dollar, P., Appel, R., Belongie, S., and Perona, P. (2014).
Fast feature pyramids for object detection. TPAMI,
36(8):1532–1545.
Doll
´
ar, P., Tu, Z., Perona, P., and Belongie, S. (2009). Inte-
gral channel features. In BMVC.
Doll
´
ar, P., Wojek, C., Schiele, B., and Perona, P. (2009).
Pedestrian detection: A benchmark. In CVPR.
Doll
´
ar, P., Wojek, C., Schiele, B., and Perona, P. (2012).
Pedestrian detection: An evaluation of the state of the
art. TPAMI, 34.
Drayer, B. and Brox, T. (2014). Training deformable object
models for human detection based on alignment and
clustering. In ECCV.
Felzenszwalb, P. F., Girshick, R. B., McAllester, D., and
Ramanan, D. (2010). Object detection with dis-
criminatively trained part-based models. TPAMI,
32(9):1627–1645.
Geiger, A., Lenz, P., and Urtasun, R. (2012). Are we ready
for autonomous driving? the kitti vision benchmark
suite. In CVPR.
Hartley, R. and Zisserman, A. (2004). Multiple View Geom-
etry in Computer Vision. Cambridge University Press.
Hosang, J., Benenson, R., Omran, M., and Schiele, B.
(2015). Taking a deeper look at pedestrians. In CVPR.
Idrees, H., Soomro, K., and Shah, M. (2015). Detecting hu-
mans in dense crowds using locally-consistent scale
prior and global occlusion reasoning. IEEE Transac-
tions on Pattern Analysis and Machine Intelligence,
37(10):1986–1998.
Kannala, J. and Brandt, S. S. (2006). A generic camera
model and calibration method for conventional, wide-
angle, and fish-eye lenses. IEEE Trans. Pattern Anal.
Mach. Intell, 28:1335–1340.
Kaur, R. and Singh, S. (2014). Background modelling, de-
tection and tracking of human in video surveillance
system. In Computational Intelligence on Power, En-
ergy and Controls with their impact on Humanity
(CIPECH), pages 54–58.
Paul, M., Haque, S. M. E., and Chakraborty, S. (2013). Hu-
man detection in surveillance videos and its applica-
tions - a review. EURASIP Journal on Advances in
Signal Processing, 2013(1):1–16.
Puig, L., Berm
´
udez, J., Sturm, P., and Guerrero, J. (2012).
Calibration of omnidirectional cameras in practice: A
comparison of methods. Comput. Vis. Image Underst.,
116(1):120–137.
Rodriguez, M., Ali, S., and Kanade, T. (2009). Tracking in
unstructured crowded scenes. In ICCV.
Rodriguez, M., Sivic, J., Laptev, I., and Audibert, J.-Y.
(2011). Density-aware person detection and tracking
in crowds. In ICCV.
Rodriguez, M. D. and Shah, M. (2007). Detecting and seg-
menting humans in crowded scenes. In ACM Multi-
media.
Roth, P., Sternig, S., Grabner, H., and Bischof, H. (2009).
Classifier grids for robust adaptive object detection. In
CVPR.
Sadeghi, M. A. and Forsyth, D. (2014). 30hz object detec-
tion with dpm v5. In European Conference on Com-
puter Vision.
Scaramuzza, D., Martinelli, A., and Siegwart, R. (2006). A
flexible technique for accurate omnidirectional cam-
era calibration and structure from motion. In In-
ternational Conference on Computer Vision Systems
(ICVS).
Solera, F., Calderara, S., and Cucchiara, R. (2016). Socially
constrained structural learning for groups detection in
crowd. IEEE Transactions on Pattern Analysis and
Machine Intelligence, 38(5):995–1008.
Stauffer, C. and Grimson, W. (1999). Adaptive background
mixture models for real-time tracking. In CVPR.
Sternig, S., Roth, P. M., and Bischof, H. (2012). On-line
inverse multiple instance boosting for classifier grids.
Pattern Recogn. Lett., 33(7):890–897.
Tang, S., Andriluka, M., and Schiele, B. (2014). Detection
and tracking of occluded people. Int. J. Comput. Vi-
sion.
Tasson, D., Montagnini, A., Marzotto, R., Farenzena, M.,
and Cristani, M. (2015). Fpga-based pedestrian detec-
tion under strong distortions. In CVPR Workshops.
Tian, Y., Luo, P., Wang, X., , and Tang, X. (2015a). Deep
learning strong parts for pedestrian detection. In
ICCV.
Tian, Y., Luo, P., Wang, X., and Tang, X. (2015b). Pedes-
trian detection aided by deep learning semantic tasks.
In CVPR.
Viola, P. and Jones, M. J. (2004). Robust real-time face
detection. Int. J. Comput. Vision, 57(2):137–154.
Yang, B., Yan, J., Lei, Z., and Li, S. Z. (2015). Convolu-
tional channel features. In ICCV.
Zhang, S., Benenson, R., Omran, M., Hosang, J., and
Schiele, B. (2016). How far are we from solving
pedestrian detection? In CVPR.
Zhang, S., Benenson, R., and Schiele, B. (2015). Filtered
channel features for pedestrian detection. In CVPR.
VISAPP 2017 - International Conference on Computer Vision Theory and Applications
148