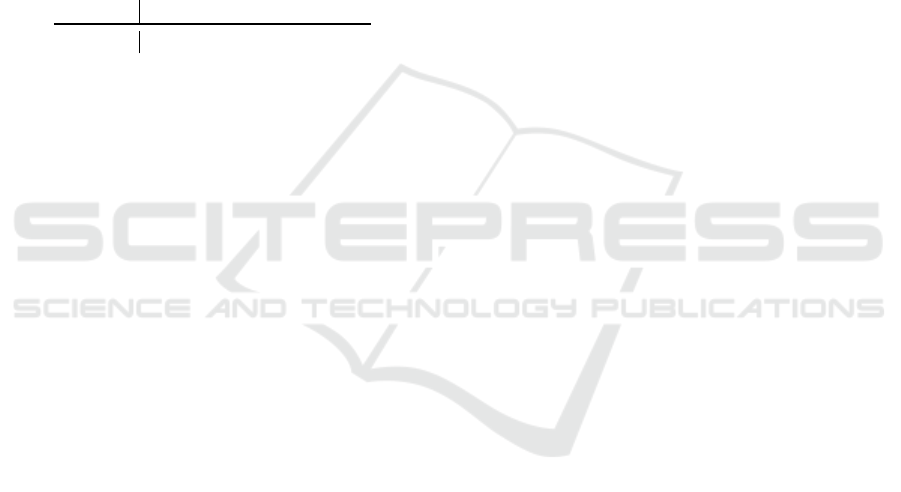
proband’s long hair, which covered the back of the up-
per body. The FFT and the Welch estimation method
were still able to predict the correct respiration rate.
4.6 Overall Comparison
Overall, 35 videos were considered for the testing. In
general, in all scenarios the respiration rate could be
classified correctly, see Table 6. While the peak de-
tection method was able to detect the correct respi-
ration rate only in 94.4 % of the cases, the FFT and
the Welch spectral estimation worked perfectly for all
scenarios. Therefore, it is recommended to use one of
those two methods to determine the frequency for the
respiration rate.
Table 6: Overall classification rates.
Method FFT Welch Peak
Overall 100 % 100 % 94.4 %
5 CONCLUSION AND FUTURE
WORK
In this study, we developed a new method for remote
respiration rate determination, which is based on four
ROIs, an optical flow based tracking, a PCA and a fre-
quency determination. Furthermore, an intense eval-
uation of different environmental parameters and sce-
narios was conducted. The results show that the pre-
sented method worked robustly in all scenarios. The
best frequency determination methods were the FFT
and the Welch spectral estimation. The results reveal
that it is possible to use such a respiration rate esti-
mation system in a domestic environment for AAL.
Further studies have to evaluate whether the accuracy
is sufficient for clinical use.
For future work, the influence of motion has to
be evaluated. If the respiration rate and the superim-
posed motion signal could be separated, this method
could be used as well in the field of e-rehabilitation
or in professional sports. Moreover, we intend to de-
velop a real-time working system to detect the respi-
ration rate immediately.
ACKNOWLEDGEMENTS
This project is funded by the European Social Fund
(ESF). We furthermore would like to express our
thanks to all the persons who contributed to this
project with their recordings.
REFERENCES
Balakrishnan, G. (2014). Analyzing pulse from head mo-
tions in video. PhD thesis, Massachusetts Institute of
Technology.
Bartula, M., Tigges, T., and Muehlsteff, J. (2013). Camera-
based system for contactless monitoring of respira-
tion. In Engineering in Medicine and Biology Society
(EMBC), 2013 35th Annual International Conference
of the IEEE, pages 2672–2675. IEEE.
Frigola, M., Amat, J., and Pags, J. (2002). Vision based
respiratory monitoring system. In Proceedings of the
10th Mediterranean Conference on Control and Au-
tomationMED2002 Lisbon, Portugal.
Horn, B. K. and Schunck, B. G. (1981). Determining optical
flow. Artificial intelligence, 17(1-3):185–203.
Jin Fei and Pavlidis, I. (2010). Thermistor at a Distance:
Unobtrusive Measurement of Breathing. IEEE Trans-
actions on Biomedical Engineering, 57(4):988–998.
Koolen, N., Decroupet, O., Dereymaeker, A., Jansen, K.,
Vervisch, J., Matic, V., Vanrumste, B., Naulaers, G.,
Van Huffel, S., and De Vos, M. (2015). Automated
Respiration Detection from Neonatal Video Data:. In
Proceedings of the International Conference on Pat-
tern Recognition Applications and Methods, pages
164–169. SCITEPRESS - Science and and Technol-
ogy Publications.
Li, M. H., Yadollahi, A., and Taati, B. (2014). A non-
contact vision-based system for respiratory rate esti-
mation. In Engineering in Medicine and Biology So-
ciety (EMBC), 2014 36th Annual International Con-
ference of the IEEE, pages 2119–2122. IEEE.
Lim, S. H., Golkar, E., Rahni, A., and Ashrani, A. (2014).
Respiratory motion tracking using the kinect camera.
In Biomedical Engineering and Sciences (IECBES),
2014 IEEE Conference on, pages 797–800. IEEE.
Lukac, T., Pucik, J., and Chrenko, L. (2014). Contactless
recognition of respiration phases using web camera.
In Radioelektronika (RADIOELEKTRONIKA), 2014
24th International Conference, pages 1–4. IEEE.
Martinez, M. and Stiefelhagen, R. (2012). Breath rate mon-
itoring during sleep using near-ir imagery and pca. In
Pattern Recognition (ICPR), 2012 21st International
Conference on, pages 3472–3475. IEEE.
Meinel, L., Richter, J., Schmidt, R., Findeisen, M., and
Hirtz, G. (2015). Opdemiva: An integrated assis-
tance and information system for elderly with demen-
tia. In Consumer Electronics (ICCE), 2015 IEEE In-
ternational Conference on, pages 76–77.
Nakajima, K., Matsumoto, Y., and Tamura, T. (2001).
Development of real-time image sequence analysis
for evaluating posture change and respiratory rate
of a subject in bed. Physiological Measurement,
22(3):N21.
Ostadabbas, S., Sebkhi, N., Zhang, M., Rahim, S., Ander-
son, L. J., Lee, F. E.-H., and Ghovanloo, M. (2015). A
Vision-Based Respiration Monitoring System for Pas-
sive Airway Resistance Estimation. IEEE Transac-
tions on Biomedical Engineering, pages 1–1.
VISAPP 2017 - International Conference on Computer Vision Theory and Applications
332