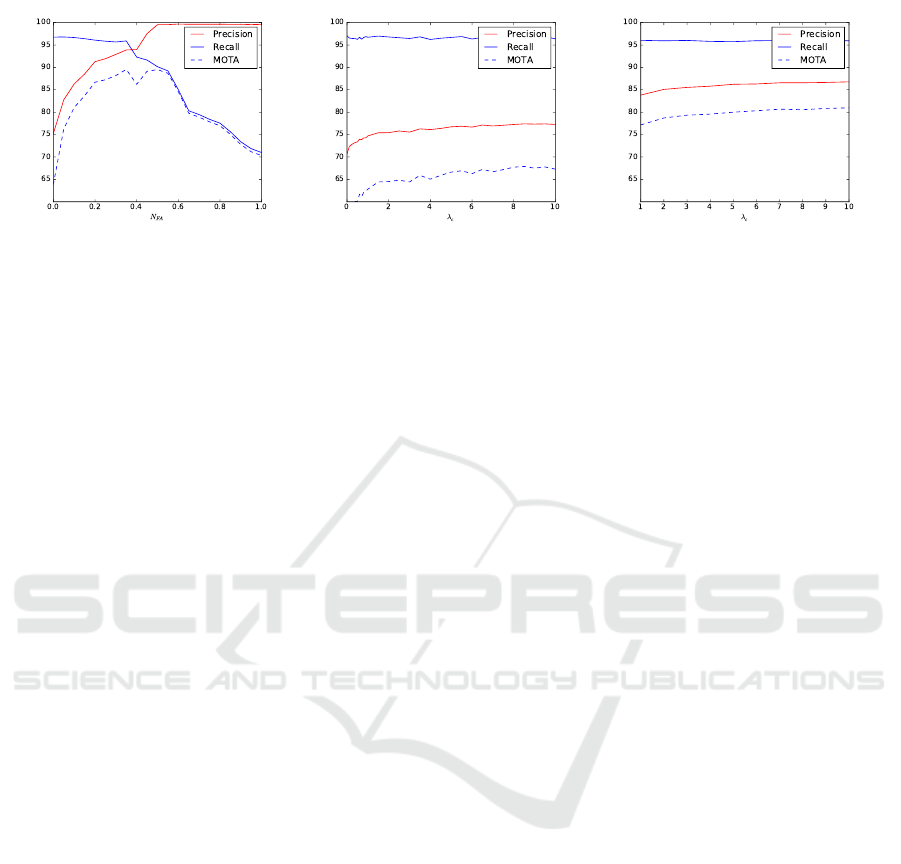
(a) N
FA
(without non maxima suppres-
sion)
(b) λ
c
(without non maxima suppres-
sion)
(c) λ
c
(with non maxima suppression)
Figure 1: Clutter parameter analysis on S2L1: (a) with detector confidence scores, (b), (c) without detector confidence scores.
tracking. In this paper, we have presented an adapted
PHD recursion that incorporates detector confidence
scores to mimic state-dependent false alarms as well
as a practical SMC implementation that can be in-
tegrated into the min-cost flow network formulation
of Wojke and Paulus (2016). Our experiments re-
vealed that integration of detector confidence scores
has considerable impact on overall applicability of
the PHD filter and, in general, our approach achieves
results competitive with the current state of the art.
FISST and the PHD filter may help to solve open
multi-object tracking problems and there is ample op-
portunity for future work, e.g., integration of appear-
ance information, application of more complex global
data association formulations, and object group track-
ing.
REFERENCES
Andriyenko, A., Schindler, K., and Roth, S. (2012).
Discrete-continuous optimization for multi-target
tracking. In CVPR, pages 1926–1933.
Berclaz, J., Fleuret, F., T
¨
uretken, E., and Fua, P. (2011).
Multiple object tracking using k-shortest paths opti-
mization. IEEE Trans. Pattern Anal. Mach. Intell.,
33(9):1806–1819.
Bernardin, K. and Stiefelhagen, R. (2008). Evaluating mul-
tiple object tracking performance: The CLEAR MOT
metrics. EURASIP J. Image Video Process, 2008.
Breitenstein, M. D., Reichlin, F., Leibe, B., Koller-Meier,
E., and Van Gool, L. (2011). Online multiper-
son tracking-by-detection from a single, uncalibrated
camera. IEEE Trans. Pattern Anal. Mach. Intell.,
33(9):1820–1833.
Dehghan, A., Tian, Y., Torr, P. H., and Mubarak, S. (2015).
Target identity-aware network flow for online multiple
target tracking. In CVPR, pages 1146–1154.
Ferryman, J. and Shahrokni, A. (2009). An overview of the
PETS 2009 challenge. In PETS.
Kim, C., Li, F., Ciptadi, A., and Rehg, J. M. (2015). Mul-
tiple hypothesis tracking revisited. In ICCV, pages
4696–4704.
Leal-Taix
´
e, L., Milan, A., Reid, I., Roth, S., and Schindler,
K. (2015). MOTChallenge 2015: Towards a bench-
mark for multi-target tracking. arXiv:1504.01942
[cs].
Maggio, E. and Cavallaro, A. (2009). Learning scene con-
text for multiple object tracking. IEEE Trans. Image
Process., 18(8):1873–1884.
Mahler, R. (2003). Multitarget Bayes filtering via first-order
multitarget moments. IEEE Trans. Aerosp. Electron.
Syst., 39(4):1152–1178.
Mahler, R. (2007). Statistical Multisource-Multitarget In-
formation Fusion. Artech House, Norwood, MA,
USA.
Mahler, R. (2014). Advances in statistical multisource-
multitarget information fusion. Artech House, Nor-
wood, MA, USA.
Mahler, R., Vo, B.-T., and Vo, B.-N. (2011). CPHD filtering
with unknown clutter rate and detection profile. IEEE
Trans. Signal Process., 59(8):3497–3513.
Milan, A., Schindler, K., and Roth, S. (2013). Detection-
and trajectory-level exclusion in multiple object track-
ing. In CVPR, pages 3682–3689.
Pirsiavash, H., Ramanan, D., and Fowlkes, C. C. (2011).
Globally-optimal greedy algorithms for tracking a
variable number of objects. In CVPR, pages 1201–
1208.
Poiesi, F., Mazzon, R., and Cavallaro, A. (2013). Multi-
target tracking on confidence maps: An applica-
tion to people tracking. Comput. Vis. Image Und.,
117(10):1257–1272.
Reid, D. B. (1979). An algorithm for tracking multiple tar-
gets. IEEE Trans. Autom. Control, 24(6):843–854.
Rezatofighi, S., Milan, A., Zhang, Z., Shi, Q., Dick, A., and
Reid, I. (2015). Joint probabilistic data association
revisited. In ICCV, pages 3047–3055.
Ristic, B., Clark, D., Vo, B.-N., and Vo, B.-T. (2012).
Adaptive target birth intensity for PHD and CPHD fil-
ters. IEEE Trans. Aerosp. Electron. Syst., 48(2):1656–
1668.
VISAPP 2017 - International Conference on Computer Vision Theory and Applications
138