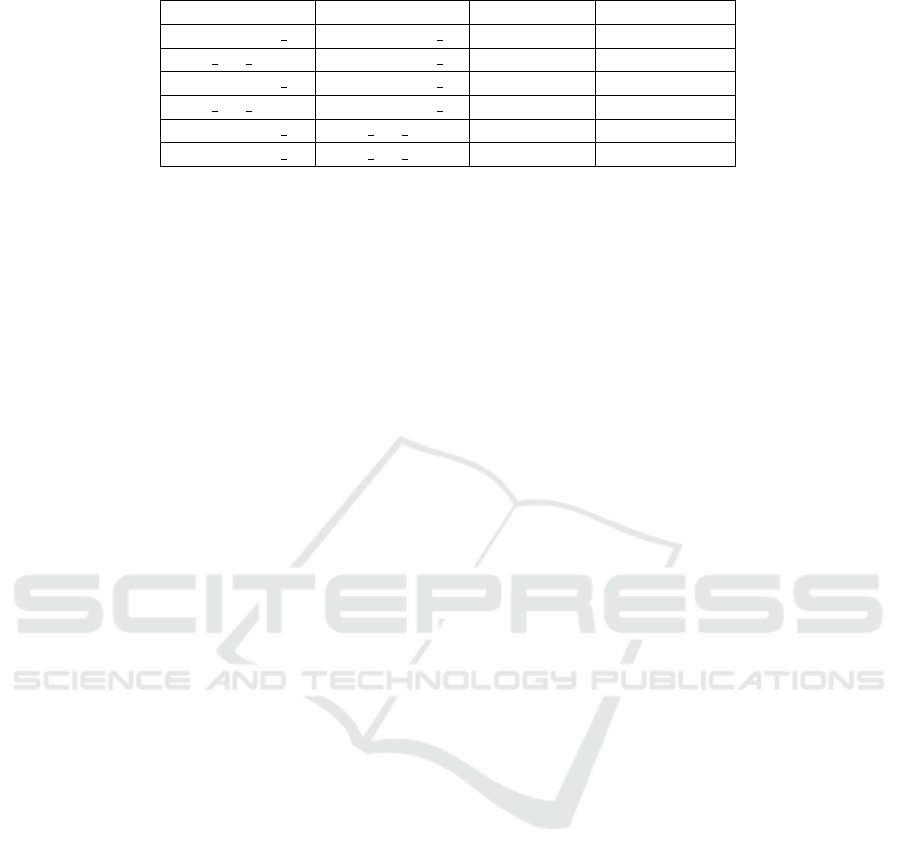
Table 6: Cross dataset performance of our method. The percentage increases in MAE and MSE are highlighted.
Source Domain Target Domain MAE MSE
Shanghaitech B Shanghaitech A 191(+52%) 337.5(+94%)
UCF CC 50 Shanghaitech A 269(+116%) 359.5(107%)
Shanghaitech A Shanghaitech B 68(+189%) 100.5(+200%)
UCF CC 50 Shanghaitech B 165(+614%) 215(+540%)
Shanghaitech A UCF CC 50 473(+40%) 680(+50%)
Shanghaitech B UCF CC 50 699(+100%) 866 (+105%)
ACKNOWLEDGMENTS
This publication has emanated from research con-
ducted with the financial support of Science
Foundation Ireland (SFI) under grant number
SFI/12/RC/2289.
REFERENCES
Chan, A. B. and Vasconcelos, N. (2012). Counting people
with low-level features and bayesian regression. IEEE
Transactions on Image Processing, 21(4):2160–2177.
Change Loy, C., Gong, S., and Xiang, T. (2013). From
semi-supervised to transfer counting of crowds. In
Proceedings of the IEEE International Conference on
Computer Vision, pages 2256–2263.
Chen, K., Loy, C. C., Gong, S., and Xiang, T. (2012). Fea-
ture mining for localised crowd counting. In BMVC,
volume 1, page 3.
Deng, J., Dong, W., Socher, R., Li, L.-J., Li, K., and Fei-
Fei, L. (2009). ImageNet: A Large-Scale Hierarchical
Image Database. In CVPR09.
Ge, W. and Collins, R. T. (2009). Marked point processes
for crowd counting. In Computer Vision and Pattern
Recognition, 2009. CVPR 2009. IEEE Conference on,
pages 2913–2920. IEEE.
Hu, Y., Chang, H., Nian, F., Wang, Y., and Li, T. (2016).
Dense crowd counting from still images with convolu-
tional neural networks. Journal of Visual Communica-
tion and Image Representation, 38:530–539.
Idrees, H., Saleemi, I., Seibert, C., and Shah, M. (2013).
Multi-source multi-scale counting in extremely dense
crowd images. In Proceedings of the IEEE Conference
on Computer Vision and Pattern Recognition, pages
2547–2554.
Jia, Y., Shelhamer, E., Donahue, J., Karayev, S., Long, J.,
Girshick, R., Guadarrama, S., and Darrell, T. (2014).
Caffe: Convolutional architecture for fast feature em-
bedding. In Proceedings of the 22nd ACM inter-
national conference on Multimedia, pages 675–678.
ACM.
Lempitsky, V. and Zisserman, A. (2010). Learning to count
objects in images. In Advances in Neural Information
Processing Systems, pages 1324–1332.
Lin, S.-F., Chen, J.-Y., and Chao, H.-X. (2001). Estimation
of number of people in crowded scenes using perspec-
tive transformation. IEEE Transactions on Systems,
Man, and Cybernetics-Part A: Systems and Humans,
31(6):645–654.
Liu, T. and Tao, D. (2014). On the robustness and gen-
eralization of cauchy regression. In 2014 4th IEEE
International Conference on Information Science and
Technology, pages 100–105. IEEE.
Long, J., Shelhamer, E., and Darrell, T. (2015). Fully convo-
lutional networks for semantic segmentation. In Pro-
ceedings of the IEEE Conference on Computer Vision
and Pattern Recognition, pages 3431–3440.
Pan, J., McGuinness, K., Sayrol, E., O’Connor, N., and Giro-
i Nieto, X. (2016). Shallow and deep convolutional
networks for saliency prediction.
Rodriguez, M., Laptev, I., Sivic, J., and Audibert, J.-Y.
(2011). Density-aware person detection and tracking
in crowds. In 2011 International Conference on Com-
puter Vision, pages 2423–2430. IEEE.
Wu, B. and Nevatia, R. (2005). Detection of multiple, par-
tially occluded humans in a single image by bayesian
combination of edgelet part detectors. In Tenth IEEE In-
ternational Conference on Computer Vision (ICCV’05)
Volume 1, volume 1, pages 90–97. IEEE.
Xie, W., Noble, J. A., and Zisserman, A. (2016). Microscopy
cell counting and detection with fully convolutional
regression networks. Computer Methods in Biome-
chanics and Biomedical Engineering: Imaging & Visu-
alization, pages 1–10.
Zhang, C., Li, H., Wang, X., and Yang, X. (2015). Cross-
scene crowd counting via deep convolutional neural
networks. In Proceedings of the IEEE Computer Soci-
ety Conference on Computer Vision and Pattern Recog-
nition, volume 07-12-June, pages 833–841.
Zhang, Y., Zhou, D., Chen, S., Gao, S., and Ma, Y. (2016).
Single-image crowd counting via multi-column convo-
lutional neural network. In Proceedings of the IEEE
Conference on Computer Vision and Pattern Recogni-
tion, pages 589–597.
Fully Convolutional Crowd Counting on Highly Congested Scenes
33