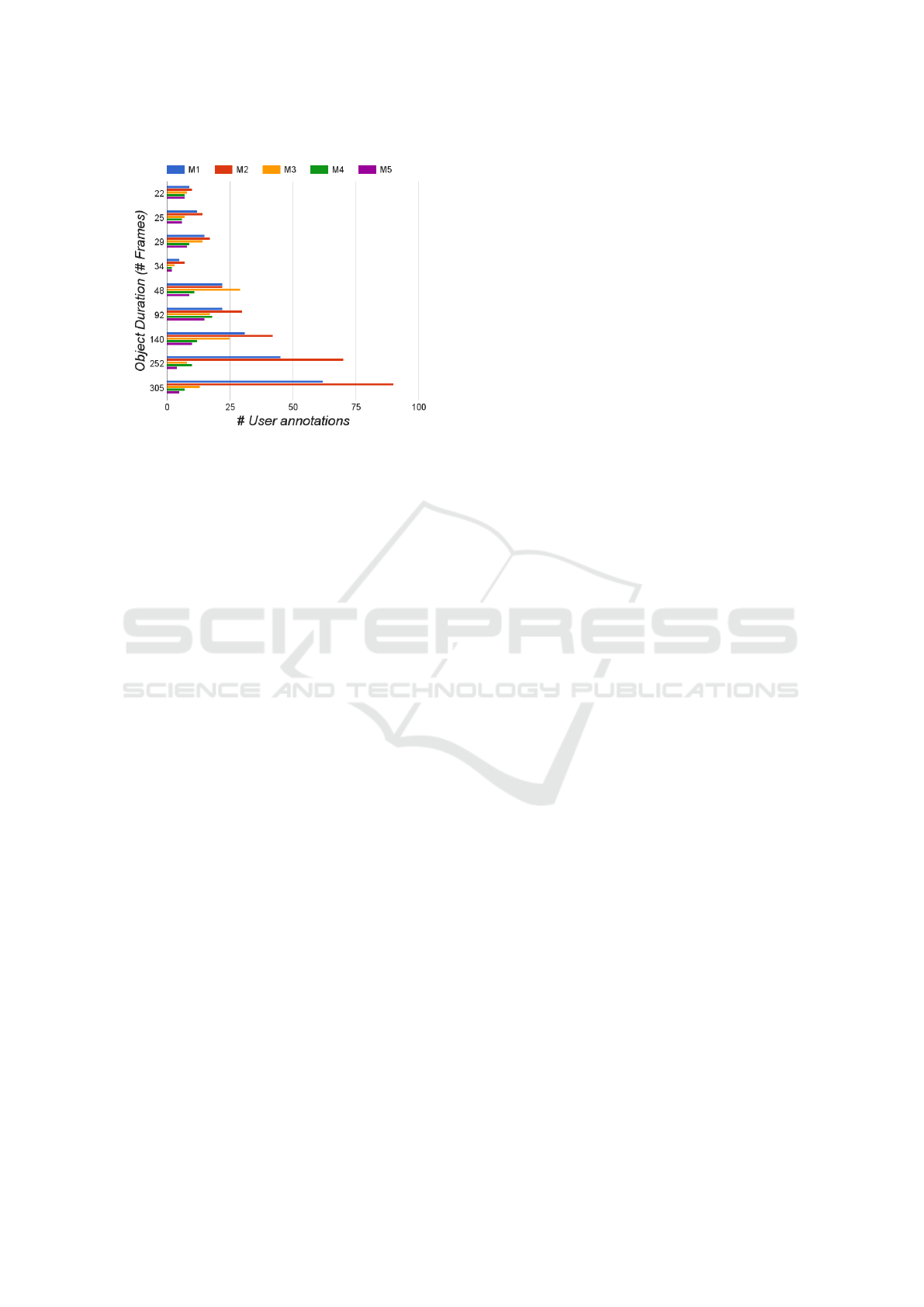
Figure 6: The number of user annotations required by ob-
jects of different length to achieve average error less than 5
pixel per frame. Clearly, M4 and M5 (proposed methods)
requires significantly less user efforts especially for objects
with longer video sequences.
long video sequences. This shows that the proposed
approach is highly scalable.
Thus, from above experiments it is clear that using
the proposed approach user efforts required for video
annotations can be reduced to 50%. Also, the method
is scalable and robust to challenges like occlusion.
6 CONCLUSION
In this paper, we propose an efficient and accurate
method to effectively annotate huge video sequences
with minimal user efforts. The approach is suitable
for generating large annotated datasets for mission
critical applications like surveillance and autonomous
driving. We effectively utilize the active learning ap-
proach to decide the best selection of key frames.
This makes our approach scalable to generate huge
annotations for large scale surveillance and automo-
tive related videos with substantial reduction in hu-
man efforts. We have verified that using the proposed
approach, annotation efforts can be reduced to half
while maintaining the track quality.
REFERENCES
Angela, Y., Juergen, G., Christian, L., and Luc, Van, G.
(2012). Interactive object detection. CVPR.
Bolme, a. S., Beveridge, J. R., Draper, B. A., and Lui, Y. M.
(2010). Visual object tracking using adaptive correla-
tion filters. CVPR.
Chatterjee, M. and Leuski, A. (2015). CRMActive: An ac-
tive learning based approach for effective Video anno-
tation and retrieval. ICMR.
Danelljan, M., Haumlger, G., Shahbaz Khan, F., and Fels-
berg, M. (2014). Accurate scale estimation for robust
visual tracking. BMVC.
Deng, J., Dong, W., Socher, R., Li, L.-J., Li, K., and Fei-
Fei, L. (2009). Imagenet: A large-scale hierarchical
image database. CVPR.
Fergus, R., Weiss, Y., and Torralba, A. (2009). Semi-
supervised learning in gigantic image collections.
NIPS.
Gray, D., Brennan, S., and Tao, H. (2007). Evaluating ap-
pearance models for recognition, reacquisition, and
tracking. PETSW.
H
¨
oferlin, B., Netzel, R., H
¨
oferlin, M., Weiskopf, D., and
Heidemann, G. (2012). Inter-active learning of ad-hoc
classifiers for video visual analytics. VAST.
Kavasidis, I., Palazzo, S., Di Salvo, R., Giordano, D., and
Spampinato, C. (2012). A semi-automatic tool for de-
tection and tracking ground truth generation in videos.
VIGTAW.
Lee, Jae, Y., and Grauman, K. (2011). Learning the
easy things first: Self-paced visual category discov-
ery. CVPR.
Oh, S. and et. al. (2011). A large-scale benchmark dataset
for event recognition in surveillance video. CVPR.
Russell, B. C., Torralba, A., Murphy, K. P., and Freeman,
W. T. (2008). Labelme: A database and web-based
tool for image annotation. IJCV.
Thomas, D., Bogdan, A., and Ferrari, V. (2010). Localizing
objects while learning their appearance. ECCV.
Vondrick, C., Patterson, D., and Ramanan, D. (2013). Ef-
ficiently scaling up crowdsourced video annotation.
IJCV.
Vondrick, C. and Ramanan, D. (2011). Video annotation
and tracking with active learning. NIPS.
Yuen, J., Russell, B., Liu, C., and Torralba, A. (2009). La-
belme video: Building a video database with human
annotations.
Zha, Z. J., Wang, M., Zheng, Y. T., Yang, Y., Hong, R., and
Chua, T. S. (2012). Interactive video indexing with
statistical active learning. Transactions on Multime-
dia.
Zhang, K. and Song, H. (2013). Real-time visual tracking
via online weighted multiple instance learning. Pat-
tern Recognition.
Zhong, D. and Chang, S.-F. (2001). Structure analysis of
sports video using domain models. International Con-
ference on Multimedia and Expo.
Zhong, H., Shi, J., and Visontai, M. (2004). Detecting un-
usual activity in video. CVPR.
Zhou, H., Yuan, Y., and Shi, C. (2009). Object tracking
using sift features and mean shift. Computer vision
and image understanding.
VISAPP 2017 - International Conference on Computer Vision Theory and Applications
360