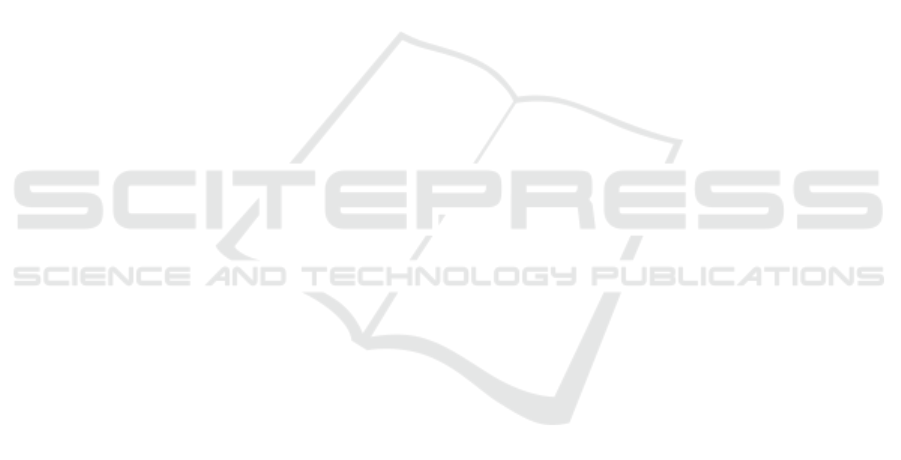
ACKNOWLEDGEMENTS
The authors were supported by the Ministerio de
Ciencia e Innovaci
´
on of the Spanish Government un-
der grant TIN2014-53772-R. During this work, J. Du-
ran had a fellowship of the Obra Social La Caixa.
REFERENCES
Alvarez, L., Deriche, R., Papadopoulo, T., and S
´
anchez, J.
(2002). Symmetrical dense optical flow estimation
with occlusions detection. In Proc. European Conf.
Computer Vision (ECCV), volume 2350 of Lecture
Notes in Comp. Sci., pages 721–735, Copenhagen,
Denmark.
Alvarez, L., Weickert, J., and S
´
anchez, J. (2000). Reliable
estimation of dense optical flow fields with large dis-
placements. Int. J. Comput. Vis., 39(1):41–56.
Barker, S., Scharstein, D., Lewis, J., Roth, S., Black, M.,
and Szeliski, R. (2011). A database and evaluaton
methodology for optical flow. Int. J. Comput. Vis.,
92(1):1–31.
Black, M. and Anandan, P. (1996). The robust estimation of
multiple motions: Parametric and piecewise smooth
flow fields. Comput. Vis. Image Underst., 63(1):75–
104.
Brox, T., Bruhn, A., Papenberg, N., and Weickert, J. (2004).
High accuracy optical flow estimation based on a the-
ory for warping. In Proc. European Conf. Com-
puter Vision (ECCV), volume 3024 of Lecture Notes
in Comp. Sci., pages 25–36, Prague, Czech Republic.
Brox, T. and Malik, J. (2011). Large displacement opti-
cal flow: Descriptor matching in variational motion
estimation. IEEE Trans. Pattern Anal. Mach. Intell.,
33(3):500–513.
Bruhn, A., Weickert, J., and Schn
¨
orr, C. (2005). Lu-
cas/Kanade meets Horn/Schunck: Combining local
and global optic flow methods. Int. J. Comput. Vis.,
61(3):211–231.
Buades, A., Coll, B., and Morel, J.-M. (2005). A review of
image denoising algorithms, with a new one. SIAM
Multiscale Model. Simul., 4(2):490–530.
Chambolle, A. (2004). An algorithm for total variation
minimization and applications. J Math. Imaging Vis.,
20(1–2):89–97.
Horn, B. and Schunck, B. (1981). Determining optical flow.
In Technical Symposium East, pages 319–331. Inter-
national Society for Optics and Photonics.
Kennedy, R. and Taylor, C. (2015). Optical flow with
geometric occlusion estimation and fusion of multi-
ple frames. In Proc. Int. Conf. Energy Minimization
Methods in Computer Vision and Pattern Recogni-
tion (EMMCVPR), volume 8932 of Lecture Notes in
Comp. Sci., pages 364–377, Hong Kong.
Lowe, D. (2004). Distinctive image features from scale-
invariant keypoints. Int. J. Comput. Vis., 60(2):91–
110.
Lucas, B. and Kanade, T. (1981). An iterative image regis-
tration technique with an application to stereo vision.
In Proc. Int. Joint Conf. Artificial Intelligence, pages
674–679, Vancouver, BC, Canada.
M
´
emin, E. and P
´
erez, P. (1998). Dense estimation and
object-based segmentation of the optical flow with
robust techniques. IEEE Trans. Image Process.,
7(5):703–719.
Mileva, Y., Bruhn, A., and Weickert, J. (2007).
Illumination-robust variational optical flow with pho-
tometric invariants. In Proc. DAGM Symposium on
Pattern Recognition, volume 4713 of Lecture Notes in
Comp. Sci., pages 152–162, Heidelberg, Germany.
Nagel, H.-H. and Enkelmann, W. (1986). An investigation
of smoothness constraints for the estimation of dis-
placement vector fields from image sequences. IEEE
Trans. Pattern Anal. Mach. Intell., 8(5):565–593.
Papenberg, N., Bruhn, A., Brox, T., Didas, S., and Weick-
ert, J. (2006). Highly accurate optic flow computation
with theoretically justified warping. Int. J. Comput.
Vis., 67(2):141–158.
Ranftl, R., Bredies, K., and Pock, T. (2014). Non-local total
generalized variation for optical flow estimation. In
Proc. European Conf. Computer Vision (ECCV), vol-
ume 8698 of Lecture Notes in Comp. Sci., pages 439–
454, Zurich, Switzerland. Springer Berlin Heidelberg.
Rudin, L., Osher, S., and Fatemi, E. (1992). Nonlinear total
variation based noise removal algorithms. Physica D,
60:259–268.
Steinbr
¨
ucker, F., Pock, T., and Cremers, D. (2009). Large
displacement optical flow computation without warp-
ing. In Proc. IEEE Int. Conf. Computer Vision (ICCV),
pages 1609–1614, Kyoto, Japan.
Trobin, W., Pock, T., Cremers, D., and Bischof, H. (2008).
An unbiased second-order prior for high-accuracy
motion estimation. In Proc. DAGM Symposium on
Pattern Recognition, volume 5096 of Lecture Notes
in Comp. Sci., pages 396–405, Munich, Germany.
Vogel, C., Roth, S., and Schindler, K. (2013). An eval-
uation of data costs for optical flow. In Proc. Ger-
man Conference Pattern Recognition (GCPR), vol-
ume 8142 of Lecture Notes in Comp. Sci., pages 343–
353, Saarbr
¨
ucken, Germany.
Wedel, A., Pock, T., Zach, C., Cremers, D., and Bischof,
H. (2009). An improved algorithm for TV-L1 optical
flow. In Proc. Statistical and Geometrical Approached
to Visual Motion Analysis, volume 5604 of Lecture
Notes in Comp. Sci., pages 23–45, Dagstuhl Castle,
Germany.
Werlberger, M., Pock, T., and Bischof, H. (2010). Mo-
tion estimation with non-local total variation regular-
ization. In Proc. IEEE Conf. Computer Vision and
Pattern Recognition (CVPR), pages 2464–2471, San
Francisco, CA, USA.
Werlberger, M., Trobin, W., Pock, T., Bischof, H., Wedel,
A., and Cremers, D. (2009). Anisotropic Huber-L1
optical flow. In Proc. British Machine Vision Confer-
ence (BMVC), pages 108.1–108.11, London, UK.
Xiao, J., Cheng, H., Sawhney, H., Rao, C., and Isnardi,
M. (2006). Bilateral filtering-based optical flow es-
VISAPP 2017 - International Conference on Computer Vision Theory and Applications
160