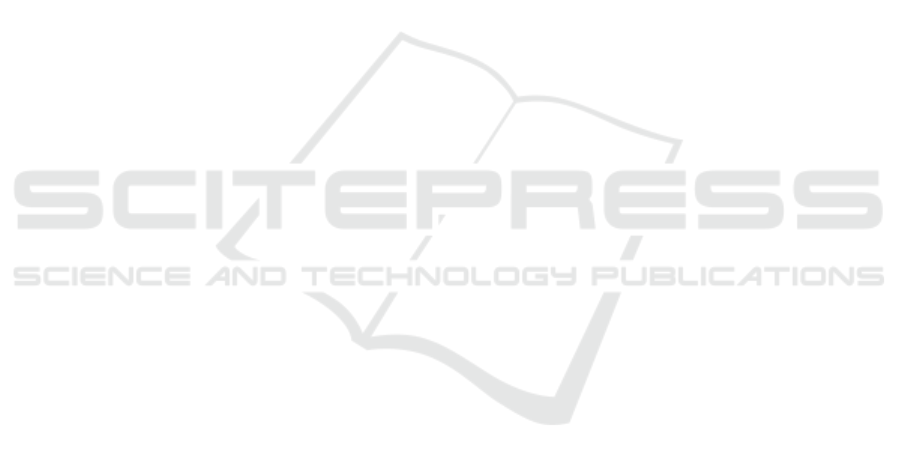
art algorithm VBM4D (Maggioni et al., 2011). It
must be remarked that this algorithm is not designed
for real image noise but for the denoising of white
and uniform noise. Moreover, an estimation of the
noise variance must be provided. In our exam-
ples we tested with different noise variances (σ =
{10,15,20,25,30}). We display the best results for
each video sequence. The figure also displays the im-
ages after enhancement. This enhancement permits
a better visualization of the noise removal but is not
part of the proposed chain. These examples show that
the proposed denoising method outperforms state of
the art techniques and illustrates the need of such a
complex chain with multi-scale and signal dependent
noise estimation and stabilization.
One short movie displaying the results of the de-
noising chain can be found in the supplementary ma-
terials accompanying this paper.
6 CONCLUSIONS
We have proposed a denoising algorithm for real
video comprising all stages, multi-scale, noise esti-
mation and denoising. The proposed algorithm has
shown to effectively remove highly correlated noise
from dark and compressed movie sequences with
weak signal-to-noise ratio.
ACKNOWLEDGEMENTS
The authors gratefully acknowledge support by grant
TIN2014-53772-R. The second author was also sup-
ported by grant TIN2014-58662-R.
REFERENCES
Boulanger, J., Kervrann, C., and Bouthemy, P. (2007).
Space-time adaptation for patch-based image se-
quence restoration. IEEE Trans. PAMI, 29(6):1096–
1102.
Buades, A., Coll, B., and Morel, J. (2011). Self-similarity-
based image denoising. Communications of the ACM,
54(5):109–117.
Buades, A., Lisani, J., and Miladinovi
´
c, M. (2016). Patch
Based Video Denoising with Optical Flow Estimation.
IEEE Transactions on Image Processing, 25(6).
Colom, M., Buades, A., and Morel, J.-M. (2014). Nonpara-
metric noise estimation method for raw images. JOSA
A, 31(4):863–871.
Dabov, K., Foi, A., Katkovnik, V., and Egiazarian, K.
(2007). Image denoising by sparse 3D transform-
domain collaborative filtering. IEEE Transactions on
image processing, 16:2007.
Dabov, K., Foi, A., Katkovnik, V., Egiazarian, K., et al.
(2009). Bm3d image denoising with shape-adaptive
principal component analysis. Proc. of the Workshop
on Signal Processing with Adaptive Sparse Structured
Representations, Saint-Malo, France.
Drago, F., Myszkowski, K., Annen, T., and Chiba, N.
(2003). Adaptive logarithmic mapping for display-
ing high contrast scenes. In Proceedings of EURO-
GRAPHICS, volume 22.
Lebrun, M., Buades, A., and Morel, J.-M. (2013). A nonlo-
cal bayesian image denoising algorithm. SIAM Jour-
nal on Imaging Sciences, 6(3):1665–1688.
Liu, C., Szeliski, R., Kang, S., Zitnick, C., and Freeman, W.
(2008). Automatic estimation and removal of noise
from a single image. IEEE transactions on pattern
analysis and machine intelligence, 30(2):299–314.
Lou, Y., Zhang, X., Osher, S., and Bertozzi, A. (2010). Im-
age recovery via nonlocal operators. Journal of Scien-
tific Computing, 42(2):185–197.
Maggioni, M., Boracchi, G., Foi, A., and Egiazarian, K.
(2011). Video denoising using separable 4D nonlocal
spatiotemporal transforms. In IS&T/SPIE Electronic
Imaging, pages 787003–787003. International Society
for Optics and Photonics.
Orchard, J., Ebrahimi, M., and Wong, A. (2008). Effi-
cient Non-Local-Means Denoising using the SVD. In
Proceedings of The IEEE International Conference on
Image Processing.
Ponomarenko, N. N., Lukin, V. V., Zriakhov, M. S., Kaarna,
A., and Astola, J. T. (2007). An automatic approach
to lossy compression of AVIRIS images. IEEE Inter-
national Geoscience and Remote Sensing Symposium.
Tomasi, C. and Manduchi, R. (1998). Bilateral filtering for
gray and color images. In Computer Vision, 1998.
Sixth International Conference on, pages 839–846.
Zhang, L., Dong, W., Zhang, D., and Shi, G. (2010). Two-
stage image denoising by principal component anal-
ysis with local pixel grouping. Pattern Recognition,
43(4):1531–1549.
Denoising of Noisy and Compressed Video Sequences
157