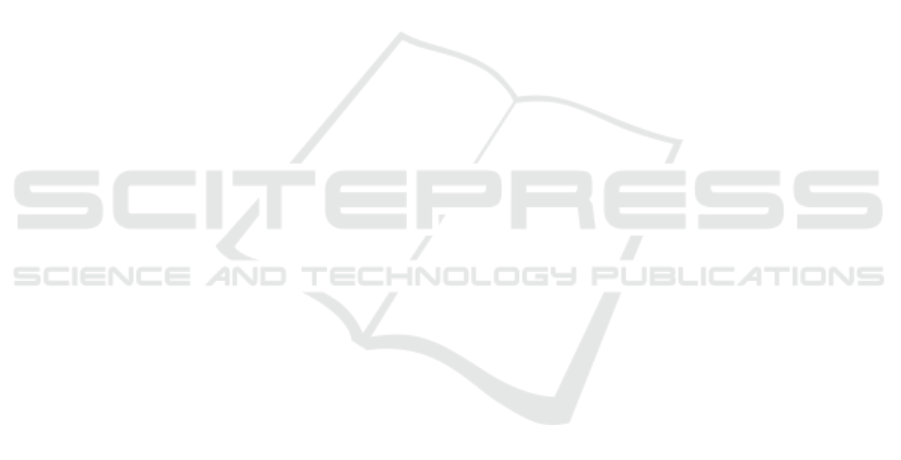
best of our knowledge, this study is unique since we
examine these three different variables.
We learned that tapered edges perform as well as
arrows for causal directions. Depictions with width
are preferred and rated higher than those with hue. De-
pictions with brightness and fuzziness showed higher
accuracy and understandability rating than granularity.
In general, depictions with hue and granularity should
be reconsidered to be used in causal representations.
Future work includes adding context to our de-
pictions and examining them with domain experts in
different application areas. It would be interesting to
see the effects of adding sequence, i.e., cause at the
top, effect at the bottom, and adding animated direc-
tion representations in a cause and effect relationship.
Another line of research is to investigate if the results
here presented are transferable to larger graphs.
ACKNOWLEDGMENTS
This research has been conducted within the "A
Big Data Analytics Framework for a Smart Society
(BIDAF 2014/32)" project, supported by the Swedish
Knowledge Foundation.
REFERENCES
Alimadadi Jani, S. (2013). Propagation of change and
visualization of causality in dependency structures
[Master thesis]. Simon Fraser University. British
Columbia, Canada.
Bisantz, A., Cao, D., Jenkins, M., Pennathur, M., P.and Farry,
Roth, E., Potter, S., & J., P. (2011). Comparing uncer-
tainty visualizations for a dynamic decision-making
task. In Journal of Cognitive Engineering and Deci-
sion Making 5(3) (pp. 277–293).
Bonneau, G.-P., Hege, H.-C., Johnson, C. R., Oliveira,
M. M., Potter, K., Rheingans, P., & Schultz, T. (2014).
Overview and state-of-the-art of uncertainty visual-
ization. In C. D. Hansen, M. Chen, C. R. Johnson,
A. E. Kaufman, & H. Hagen (Eds.), Scientific visu-
alization: Uncertainty, multifield, biomedical, and
scalable visualization (pp. 3–27). London: Springer
London.
Chen, M., Trefethen, A., Bañares Alcántara, R., Jirotka, M.,
Coecke, B., Ertl, T., & Schmidt, A. (2011). From
data analysis and visualization to causality discovery.
In Computer, 44(10) (pp. 84–87).
Elmqvist, N., & Tsigas, P. (2003). Growing squares: Ani-
mated visualization of causal relations. In Proceed-
ings of the 2003 ACM Symposium on Software Visu-
alization (pp. 17–26).
Fruchterman, T. M., & Reingold, E. M. (1991). Graph draw-
ing by force-directed placement. Software: Practice
and Experience, 21(11), 1129–1164.
Ghoniem, M., Fekete, J.-D., & Castagliola, P. (2004). A
comparison of the readability of graphs using node-
link and matrix-based representations. In Proc. of the
4th IEEE Symposium on Information Visualization
(INFOVIS’04) (p. 17-24).
Guo, H., Huang, J., & Laidlaw, D. (2015). Representing un-
certainty in graph edges: An evaluation of paired vi-
sual variables. In IEEE Transactions on Visualization
and Computer Graphics, 21(10) (pp. 1173–1186).
Holten, D., Isenberg, P., Van Wijk, J., & Fekete, J. (2011).
An extended evaluation of the readability of tapered,
animated, and textured directed-edge representations
in node-link graphs. In In 2011 IEEE Pacific Visual-
ization Symposium (pp. 195–202).
Holten, D., & van Wijk, J. (2009). A user study on visualiz-
ing directed edges in graphs. In In Proceedings of the
SIGCHI Conference on Human Factors in Computing
Systems (pp. 2299–2308).
Kadaba, N. R., Irani, P. P., & Leboe, J. (2007). Visualizing
causal semantics using animations. IEEE Transac-
tions on Visualization and Computer Graphics, 13(6),
1254-1261.
Kubí
ˇ
cek, P., & Šašinka,
ˇ
C. (2011). Thematic uncertainty
visualization usability–comparison of basic methods.
Annals of GIS, 17(4), 253–263.
MacEachren, A. M., Robinson, A., Hopper, S., Gardner,
S., Murray, R., Gahegan, M., & Hetzler, E. (2005).
Visualizing geospatial information uncertainty: What
we know and what we need to know. Cartography and
Geographic Information Science, 32(3), 139–160.
MacEachren, A. M., Roth, R. E., O’Brien, J., Li, B., Swing-
ley, D., & Gahegan, M. (2012). Visual semiotics &
uncertainty visualization: An empirical study. IEEE
Transactions on Visualization and Computer Graph-
ics, 18(12), 2496–2505.
Pane, J. F., Corbett, A. T., & John, B. E. (1996). Assessing
dynamics in computer-based instruction. In Proc. of
the SIGCHI Conference on Human Factors in Com-
puting Systems (pp. 197–204).
Potter, K., Rosen, P., & Johnson, C. R. (2012). From quan-
tification to visualization: A taxonomy of uncertainty
visualization approaches. In Uncertainty Quantifica-
tion in Scientific Computing (pp. 226–249). Springer.
Sanyal, J., Zhang, S., Bhattacharya, G., Amburn, P., & Moor-
head, R. (2009). A user study to compare four uncer-
tainty visualization methods for 1D and 2D datasets.
IEEE Trans. on Visualization and Computer Graph-
ics, 15, 1209–1218.
Scholz, R. W., & Lu, Y. (2014). Uncertainty in geographic
data on bivariate maps: An examination of visualiza-
tion preference and decision making. ISPRS Interna-
tional Journal of Geo-Information, 3(4), 1180–1197.
Tversky, B., Morrison, J. B., & Betrancourt, M. (2002).
Animation: can it facilitate? International Journal of
Human-Computer Studies, 57(4), 247–262.
Wang, J., & Mueller, K. (2016). The visual causality ana-
lyst: An interactive interface for causal reasoning. In
IEEE Transactions on Visualization and Computer
Graphics, 22(1) (pp. 230–239).
Zuk, T., & Carpendale, S. (2006). Theoretical analysis
of uncertainty visualizations. In Proceedings of the
SPIE-VDA (Vol. 6060).
IVAPP 2017 - International Conference on Information Visualization Theory and Applications
74