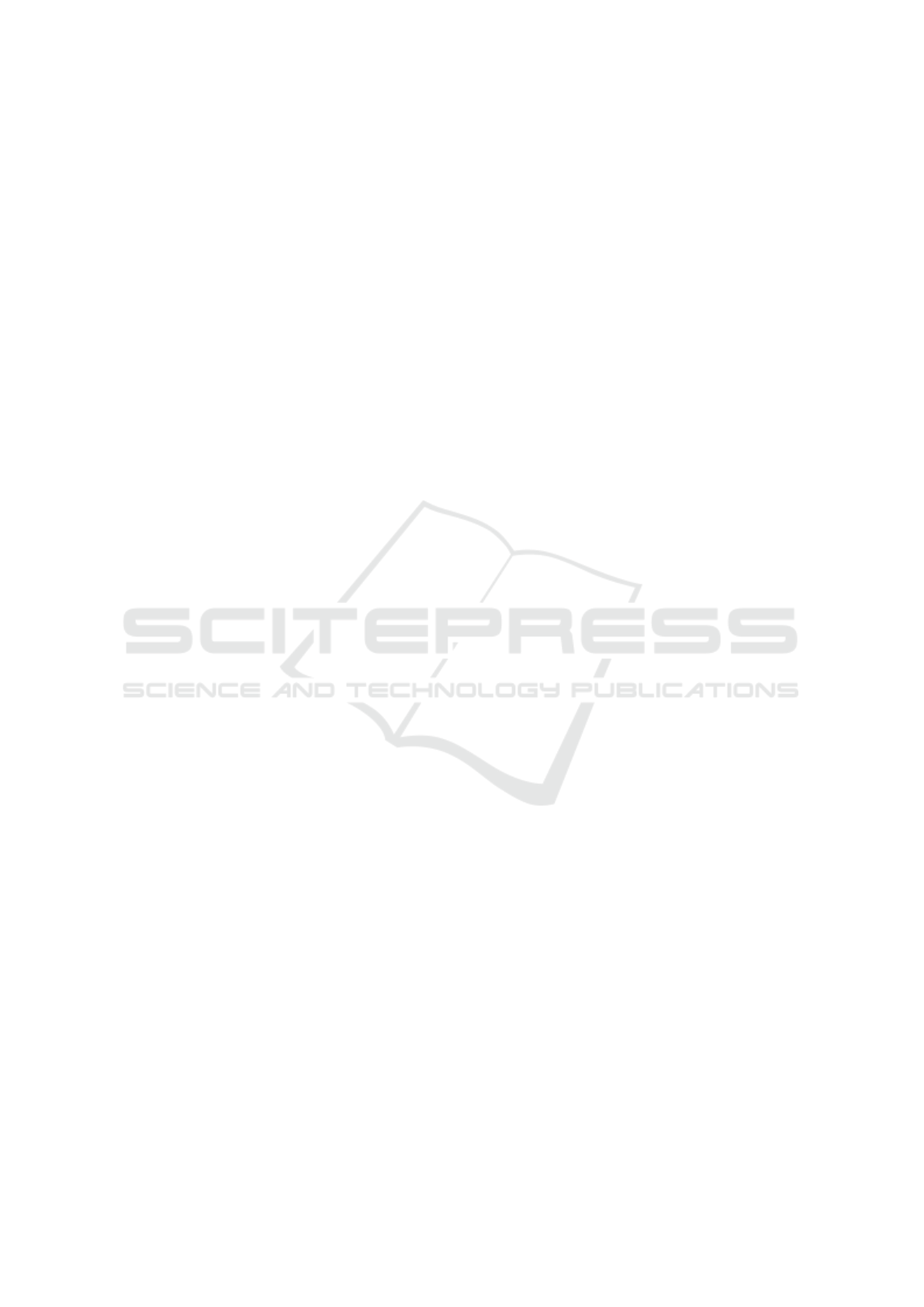
scheduler, which can be executed in parallel, unlike
those from (Bleyer et al., 2011; Besse et al., 2012;
Zhang et al., 2015). The proposed similarity function
is also capable of reducing some of the loss of detail
and error caused by the popular adaptive window ap-
proach.
ACKNOWLEDGEMENTS
This research was done with the support of the Mex-
ican CONACYT programme and the European Com-
mission.
REFERENCES
Achanta, R., Shaji, A., Smith, K., Lucchi, A., Fua, P.,
and S
¨
usstrunk, S. (2012). Slic superpixels com-
pared to state-of-the-art superpixel methods. PAMI,
34(11):2274–2282.
Besse, F., Rother, C., Fitzgibbon, A., and Kautz, J. (2012).
Pmbp: Patchmatch belief propagation for correspon-
dence field estimation. International Journal of Com-
puter Vision, 110(1):2–13.
Bleyer, M., Rhemann, C., and Rother, C. (2011). Patch-
match stereo - stereo matching with slanted support
windows. In Proceedings of the British Machine Vi-
sion Conference, 11:1–11.
Geiger, A., Lenz, P., and R.Urtasun (2012). Are we ready
for autonomous driving? the kitti vision benchmark
suite. CVPR, pages 3354–336.
Guney, F. and Geiger, A. (2015). Displets: Resolving stereo
ambiguities using object knowledge. CVPR.
Heise, P., Klose, S., Jensen, B., and Knoll, A. (2013). Patch-
match with huber regularization for stereo matching.
ICCV, pages 2360–2367.
Klaus, A., Sormann, M., and Karner, K. (2006). Segment-
based stereo matching using belief propagation and a
self-adapting dissimilarity measure. ICPR, 3:15–18.
Kolmogorov, V. (2007). Convergent tree-reweighted mes-
sage passing for energy minimization. IEEE Trans-
actions on Pattern Analysis and Machine Intelligence,
28(10):1568–1583.
Kolmogorov, V. and Zabih, R. (2001). Computing vi-
sual correspondence with occlusions using graph cuts.
ICCV, 2:508–515.
Li, A., Chen, D., Liu, Y., and Yuan, Z. (2016a). Coordi-
nating multiple disparity proposals for stereo compu-
tation. CVPR.
Li, L., Zhang, S., Yu, X., and Zhang, L. (2016b). Pmsc:
Patchmatch-based superpixel cut for accurate stereo
matching. IEEE Transactions on Circuits and Systems
for Video Technology.
Li, Y., Min, D., Brown, M. S., Do, M. N., and Lu, J. (2015).
Sped-up patchmatch belief propagation. ICCV.
Menze, M. and Geiger, A. (2015). Object scene flow for
autonomous vehicles. CVPR.
Olsson, C., Ul
´
en, J., and Boykov, Y. (2013). In defense of
3d-label stereo. CVPR, pages 1730–1737.
Park, M.-G. and Yoon, K.-J. (2016). As-planar-as-possible
depth map estimation. Submitted to IEEE TPAMI.
Scharstein, D., Hirschm
¨
uller, H., Kitajima, Y., Krathwohl,
G., Nesic, N., Wang, X., and Westling, P. (2014).
High-resolution stereo datasets with subpixel-accurate
ground truth. In German Conference on Pattern
Recognition (GCPR), pages 31–42.
Sinha, S., Scharstein, D., and Szeliski, R. (2014). Ef-
ficient high-resolution stereo matching using local
plane sweeps. CVPR, pages 1582–1589.
Taniai, T., Matsushita, Y., and Naemura, T. (2014). Graph
cut based continuous stereo matching using locally
shared labels. CVPR, pages 1613–1620.
Vedaldi, A. and Fulkerson, B. (2008). VLFeat: An open
and portable library of computer vision algorithms.
http://www.vlfeat.org/.
Vedaldi, A. and Soatto, S. (2008). Quick shift and kernel
methods for mode seeking. ECCV, 5305:705–718.
Vogel, C., Schindler, K., Konrad, and Roth, S. (2015). 3d
scene flow estimation with a piecewise rigid scene
model. IJCV, pages 1–28.
ˇ
Zbontar, J. and LeCun, Y. (2015). Stereo matching by train-
ing a convolutional neural network to compare image
patches. Submitted to JMLR.
Woodford, O. J., Reid, I. D., Torr, P. H. S., and Fitzgibbon,
A. W. (2007). On new view synthesis using multiview
stereo. In Proceedings of the British Machine Vision
Conference, pages 1–10.
Yamaguchi, K., McAllester, D., and Urtasun, R. (2014). Ef-
ficient joint segmentation, occlusion labeling, stereo
and flow estimation. ECCV, pages 756–771.
Yoon, K. and Kweon, I. (2006). Adaptive support-weight
approach for correspondence search. IEEE Transac-
tions on Pattern Analysis and Machine Intelligence,
28(4):650–656.
Zabih, R. and Li, J. (1994). Non-parametric local trans-
forms for computing visual correspondence. ECCV,
12:151–158.
Zhang, C., Li, Z., Cheng, Y., Cai, R., Chao, H., and Rui,
Y. (2015). Meshstereo: A global stereo model with
mesh alignment regularization for view interpolation.
ICCV.
Zhang, K., Lu, J., and Lafruit, G. (2009). Cross-based lo-
cal stereo matching using orthogonal integral images.
IEEE Transactions on Circuits and Systems for Video
Technology, 19(7):1073–1079.
3D Plane Labeling Stereo Matching with Content Aware Adaptive Windows
171