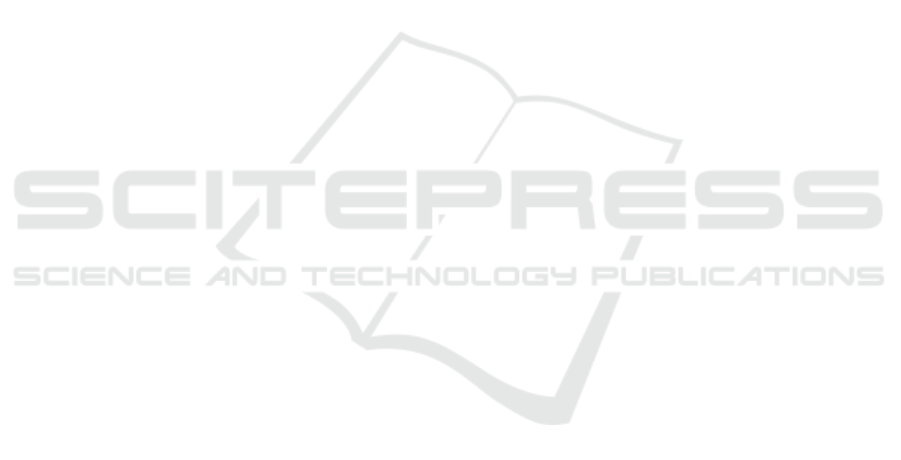
tion for each participant is needed. However, short
recording of free breathing, for various body posi-
tions, seemed to be enough to get the highest accuracy
of tidal volume estimation.
Raw impedance signal obtained from the chest
consists of both respiratory and cardiac components,
the second most commonly regarded as an element
to be removed. The study showed, that there is the
possibility to measure and extract each component
from impedance pneumography separately, with re-
liable accuracy, 86.5% and 97.3%, respectively for
tidal volume and heart rate estimation.
Simple moving average smoothing (with 1s win-
dow for respiratory analysis, and with 1.5s window
for cardiac one) were the best algorithm regarding
compromise between tidal volume and heart rate ac-
curacy, and time of processing. More sophisticated
adaptive filtering also provided good accuracy, how-
ever the processing time was 100-times higher, com-
paring to simple methods.
Cardiac component is not equally visible in ev-
ery participant, however obtained compatibility be-
tween ECG reference seems promising, particularly
concerning ambulatory long-term measurements.
ACKNOWLEDGEMENTS
This study was supported by the research programs of
institutions the authors are affiliated with.
REFERENCES
Ansari, S., Ward, K., and Najarian, K. (2016). Motion Ar-
tifact Suppression in Impedance Pneumography Sig-
nal for Portable Monitoring of Respiration: an Adap-
tive Approach. IEEE J. Biomed. Heal. Informatics,
11(4):1–1.
Barros, A. K., Yoshizawa, M., and Yasuda, Y. (1995). Fil-
tering noncorrelated noise in impedance cardiogra-
phy. IEEE Transactions on Biomedical Engineering,
42(3):324–327.
Houtveen, J. H., Groot, P. F. C., and De Geus, E. J. C.
(2006). Validation of the thoracic impedance derived
respiratory signal using multilevel analysis. Int. J.
Psychophysiol., 59(2):97–106.
Koivumaki, T., Vauhkonen, M., Kuikka, J. T., and Haku-
linen, M. a. (2012). Bioimpedance-based measure-
ment method for simultaneous acquisition of respi-
ratory and cardiac gating signals. Physiol. Meas.,
33(8):1323–1334.
Mathworks (2016). Wavelet Denoising.
http://www.mathworks.com/help/wavelet/ug/wavelet-
denoising.html. [Online; accessed 07-August-2016].
Mły
´
nczak, M. and Cybulski, G. (2012). Impedance pneu-
mography: Is it possible? In XXX Symp. Photonics
Appl. Astron. Commun. Ind. High-Energy Phys. Exp.
(Wilga 2012), volume 8454, pages 84541T–19.
Mły
´
nczak, M., Niewiadomski, W.,
˙
Zyli
´
nski, M., and Cybul-
ski, G. (2015). Verification of the Respiratory Param-
eters Derived from Impedance Pnumography during
Normal and Deep Breathing in Three Body Postures.
In IFMBE Proc., volume 45, pages 162–165.
Poupard, L., Mathieu, M., Sart
`
ene, R., and Goldman, M.
(2008). Use of thoracic impedance sensors to screen
for sleep-disordered breathing in patients with cardio-
vascular disease. Physiol. Meas., 29(2):255–267.
Reinsch, C. H. (1967). Smoothing by spline functions. Nu-
merische Mathematik, 10(3):177–183.
Savitzky, A. and Golay, M. J. E. (1964). Smoothing and
Differentiation of Data by Simplified Least Squares
Procedures. Anal. Chem., 36(8):1627–1639.
Schoenberg, I. J. (1964). Spline functions and the problem
of graduation. Proceedings of the National Academy
of Sciences, 52(4):947–950.
Schuessler, T. F., Gottfried, S. B., Goldberg, P., Kearney,
R. E., and Bates, J. H. T. (1998). An adaptive filter
to reduce cardiogenic oscillations on esophageal pres-
sure signals. Ann. Biomed. Eng., 26(2):260–267.
Seppa, V. P., Hyttinen, J., Uitto, M., Chrapek, W., and
Viik, J. (2013a). Novel electrode configuration for
highly linear impedance pneumography. Biomed. Eng.
/ Biomed. Tech., 58(1):35–38.
Seppa, V.-P., Hyttinen, J., and Viik, J. (2011). A method
for suppressing cardiogenic oscillations in impedance
pneumography. Physiol. Meas., 32(3):337–345.
Seppa, V. P., Uitto, M., and Viik, J. (2013b). Tidal breath-
ing flow-volume curves with impedance pneumogra-
phy during expiratory loading. In Proc. Annu. Int.
Conf. IEEE Eng. Med. Biol. Soc. EMBS, volume 2013,
pages 2437–2440.
Seppa, V.-P., Viik, J., and Hyttinen, J. (2010). Assessment
of pulmonary flow using impedance pneumography.
IEEE Trans. Biomed. Eng., 57(9):2277–2285.
Wang, Z., Wu, D., Chen, J., Ghoneim, A., and Hossain,
M. A. (2016). A Triaxial Accelerometer-Based Hu-
man Activity Recognition via EEMD-Based Features
and Game-Theory-Based Feature Selection. IEEE
Sens. J., 16(9):3198–3207.
Yasuda, Y., Umezu, A., Horihata, S., Yamamoto, K.,
Miki, R., and Koike, S. (2005). Modified thoracic
impedance plethysmography to monitor sleep apnea
syndromes. Sleep Med., 6(3):215–224.
Decomposition of the Cardiac and Respiratory Components from Impedance Pneumography Signals
33