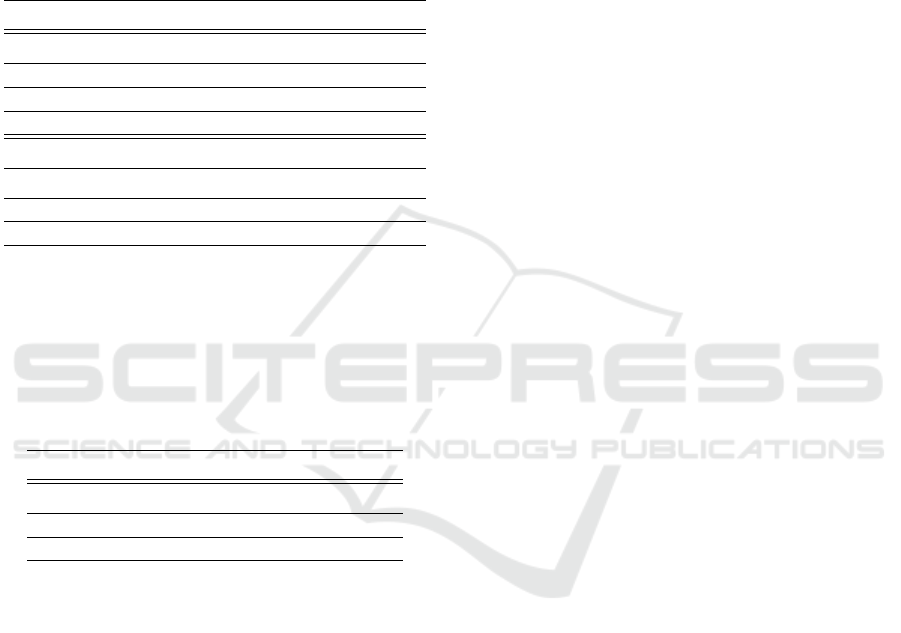
Table 1: Results in MPI-Sintel training set for the optical
flow (u, v) and for the disparity change δd. The first and
second set of results correspond, respectively, to the Final
and Clean frames. EPE means endpoint error over the com-
plete frames. EPE-M shows the endpoint error over regions
that remain visible in adjacent frames. EPE-U shows the
endpoint error over regions that are visible only in one of
the two adjacent frames. Notice that the ground truth δd
is not provided in the database. We have set δd(x, y) =
δd
t+1
(x + u, y + v) −d(x, y) using the (u, v, d
t
, d
t+1
) ground
truth values. Using that information we have obtained the
EPE-δd for all the image.
EPE EPE-M EPE-U EPE-δd
Final
Classic Wedel 9.1461 7.7189 17.7888 1.1234
Our Wedel 7.6287 5.3934 19.8561 0.8121
Our Proposal 7.5095 5.2406 18.9948 0.7997
Clean
Classic Wedel 8.6722 7.2324 17.2608 1.03522
Our Wedel 4.5097 2.2905 15.5042 0.5634
Our Proposal 4.3041 2.1558 15.1603 0.5521
Table 2: Results in KITTI 2015 training dataset for the op-
tical flow (u, v) and for the disparity change δd. Out-noc
(resp. Out-all) refers to the percentage of pixels where the
estimated optical flow presents an error above 3 pixels in
non-occluded areas (resp. all pixels). Out-δd refers to the
percentage of pixels where the estimated disparity change
presents an error above 3 pixels in the pixels where the dis-
parity is available.
Out-noc Out-all Out-δd
Classic Wedel 45.8745 55.4356 42.8971
Our Wedel 24.4237 33.2209 31.8971
Our Proposal 23.5233 32.8576 30.7532
terms for the occluded pixels, i.e., data terms that de-
pend on the views where these pixels might be visible.
We also have extended the optimization method for
optical flow problems presented in (Palomares et al.,
2016) to the scene flow case. Experimental results
show, both quantitative and qualitatively, the benefits
of the proposed energy functional and the minimiza-
tion strategy. As future work we plan to use regular-
ization and data terms that better preserve the image
boundaries and that are more robust to illumination
changes.
ACKNOWLEDGEMENTS
The authors acknowledge partial support by
TIN2015-70410-C2-1-R (MINECO/FEDER, UE)
and by GRC reference 2014 SGR 1301, Generalitat
de Catalunya.
REFERENCES
Ayvaci, A., Raptis, M., and Soatto, S. (2012). Sparse occlu-
sion detection with optical flow. International Journal
of Computer Vision, 97(3):322–338.
Ballester, C., Garrido, L., Lazcano, V., and Caselles, V.
(2012). A tv-l1 optical flow method with occlusion de-
tection. In Pinz, A., Pock, T., Bischof, H., and Leberl,
F., editors, DAGM/OAGM Symposium, volume 7476
of Lecture Notes in Computer Science, pages 31–40.
Springer.
Basha, T., Moses, Y., and Kiryati, N. (2013). Multi-view
scene flow estimation: A view centered variational
approach. International Journal of Computer Vision,
101(1):6–21.
Brox, T., Bruhn, A., Papenberg, N., and Weickert, J. (2004).
High accuracy optical flow estimation based on a the-
ory for warping. In European Conference on Com-
puter Vision (ECCV), volume 3024 of Lecture Notes
in Computer Science, pages 25–36. Springer.
Butler, D. J., Wulff, J., Stanley, G. B., and Black, M. J.
(2012). A naturalistic open source movie for optical
flow evaluation. In European Conference on Com-
puter Vision, pages 611–625.
Cech, J., Sanchez-Riera, J., and Horaud, R. P. (2011). Scene
flow estimation by growing correspondence seeds. In
Proceedings of the IEEE Conference on Computer Vi-
sion and Pattern Recognition, pages 3129–3136.
Geiger, A., Lenz, P., and Urtasun, R. (2012). Are we ready
for autonomous driving? the kitti vision benchmark
suite. In Conference on Computer Vision and Pattern
Recognition (CVPR).
Huguet, F. and Devernay, F. (2007). A variational method
for scene flow estimation from stereo sequences. In
Computer Vision, 2007. ICCV 2007. IEEE 11th Inter-
national Conference on, pages 1–7.
Ince, S. and Konrad, J. (2008). Occlusion-aware optical
flow estimation. IEEE Transactions on Image Process-
ing, 17(8):1443–1451.
Jaimez, M., Souiai, M., Stueckler, J., Gonzalez-Jimenez,
J., and Cremers, D. (2015). Motion coopera-
tion: Smooth piece-wise rigid scene flow from rgb-
d images. In Proc. of the Int. Conference on
3D Vision (3DV). ¡a href=”https://youtu.be/qjPsKb-
˙kvE”target=”˙blank”¿[video]¡/a¿.
Menze, M. and Geiger, A. (2015). Object scene flow for au-
tonomous vehicles. In The IEEE Conference on Com-
puter Vision and Pattern Recognition (CVPR).
Palomares, R. P., Meinhardt-Llopis, E., Ballester, C., and
Haro, G. (2016). Faldoi: A new minimization strategy
for large displacement variational optical flow. Journal
of Mathematical Imaging and Vision, pages 1–20.
Pons, J. P., Keriven, R., and Faugeras, O. (2007). Multi-
view stereo reconstruction and scene flow estimation
Joint Large Displacement Scene Flow and Occlusion Variational Estimation
179