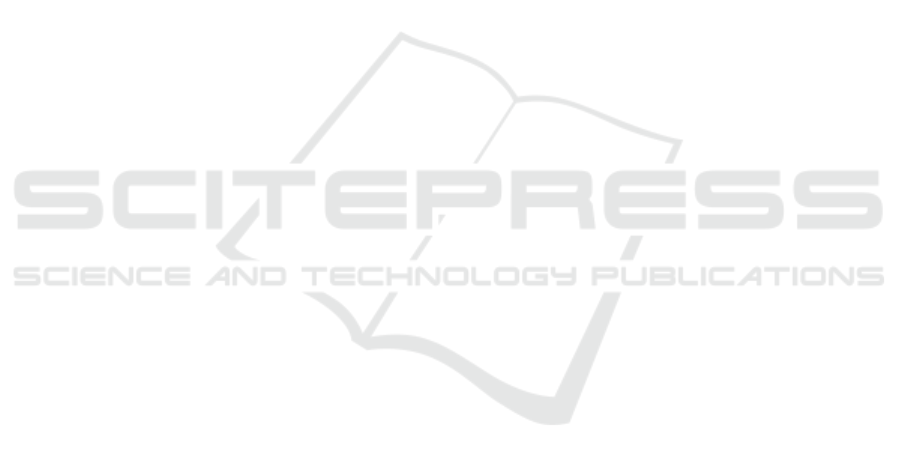
ordinates, to a central unit for deriving the estimate.
Other solutions could involve the sending of query
messages, e.g. from cellular base stations or WLAN
access points. The mobile devices would receive the
messages, execute the measurement and transmit the
result and their position to the sender.
The method should also be checked for its effi-
ciency when the movements of the mobile devices are
described by other mobility models or are not inde-
pendent, as in the cases where they move in groups,
or move towards popular places. The RWP model has
been shown to be a good approximation for modeling
the motion of vehicles in a road (Saha and Johnson,
2004). Models for better approximating human mo-
bility have been described in (Gonzalez et al., 2008;
Rhee et al., 2011), while non-independence of mobile
movements through group mobility models has been
studied in (Musolesi et al., 2004),(Lee et al., 2009).
The RWP model is a super-diffusive model, which
means that there is a higher probability of longer dis-
placements; hence the mobile locations are likely to
be even more concentrated towards the center in a
more realistic model. Clustered user movements will
skew the location distribution, so that the estimated
average is farther from the true value. Neverthe-
less, the method with stratification has the effect of
smoothing out the skewness and therefore could still
produce a significant improvement. As a matter of
fact, the higher the skewness, the higher the expected
bias reduction by the stratification method. Therefore
we expect even more gains by applying the stratifi-
cation method under more realistic mobility models,
than under the RWP model.
Furthermore, we have not been concerned with the
accuracy of single user measurements, or the effect of
noise in such measurements. The authors in (Fiore
et al., 2013) showed that the accuracy of the mea-
surements collected by the users plays a more critical
role than the number of users participating in crowd-
sensing, and an accurate overall estimate can be ob-
tained with a relatively small number of accurate user
measurements. Hence it is important to examine the
accuracy of user measurements, and filter out mea-
surements that are suspected to be inaccurate. In the
application scenario examined in this paper, devices
in vehicles could more accurately measure ambient
temperature than devices carried by humans, as direct
contact with ambient air is always achieved. In both
cases, filtering of measurements would be required to
eliminate possible sources of bias: indoor environ-
ments (detection of indoor/outdoor environment as in
(Krumm and Hariharan, 2004)), human contact with
the sensor, exhaustion gas from other vehicles, etc.
Finally, there exist many other challenges for con-
ducting crowdsensing measurements, such as provid-
ing participation incentives to the users, or protecting
from malicious users who may “pollute” the data. In-
terested readers are referred to the surveys (Ma et al.,
2014; Ganti et al., 2011) for basic information.
REFERENCES
Antonic, A., Bilas, V., Marjanovic, M., Matijasevic, M.,
Oletic, D., Pavelic, M., Zarko, I. P., Pripuzic, K.,
and Skorin-Kapov, L. (2014). Urban crowd sensing
demonstrator: Sense the Zagreb air. In Software,
Telecommunications and Computer Networks (Soft-
COM), 2014 22nd International Conference on, pages
423–424. IEEE.
Bash, B. A., Byers, J. W., and Considine, J. (2004). Approx-
imately uniform random sampling in sensor networks.
In Proceeedings of the 1st international workshop on
Data management for sensor networks: in conjunc-
tion with VLDB 2004, pages 32–39. ACM.
Bettstetter, C. and Wagner, C. (2002). The spatial node
distribution of the random waypoint mobility model.
WMAN, 11:41–58.
Cochran, W. G. (1946). Relative accuracy of systematic
and stratified random samples for a certain class of
populations. The Annals of Mathematical Statistics,
pages 164–177.
Considine, J., Li, F., Kollios, G., and Byers, J.
(2004). Approximate aggregation techniques for sen-
sor databases. In Data Engineering, 2004. Proceed-
ings. 20th International Conference on, pages 449–
460. IEEE.
Datta, S. and Kargupta, H. (2007). Uniform data sampling
from a peer-to-peer network. In Distributed Com-
puting Systems, 2007. ICDCS’07. 27th International
Conference on, pages 1–8. IEEE.
Fiore, M., Nordio, A., and Chiasserini, C.-F. (2013). In-
vestigating the accuracy of mobile urban sensing. In
Wireless On-demand Network Systems and Services
(WONS), 2013 10th Annual Conference on, pages 25–
28. IEEE.
Ganesan, D., Ratnasamy, S., Wang, H., and Estrin, D.
(2004). Coping with irregular spatio-temporal sam-
pling in sensor networks. ACM SIGCOMM Computer
Communication Review, 34(1):125–130.
Ganti, R. K., Ye, F., and Lei, H. (2011). Mobile crowdsens-
ing: current state and future challenges. Communica-
tions Magazine, IEEE, 49(11):32–39.
Glynn, P. and Sigman, K. (1998). Independent sampling of
a stochastic process. Stochastic processes and their
applications, 74(2):151–164.
Gonzalez, M. C., Hidalgo, C. A., and Barabasi, A.-L.
(2008). Understanding individual human mobility
patterns. Nature, 453(7196):779–782.
Hyyti
¨
a, E., Lassila, P., and Virtamo, J. (2006). Spatial node
distribution of the random waypoint mobility model
with applications. IEEE Transactions on Mobile Com-
puting, 5(6):680–694.
Estimating Spatial Averages of Environmental Parameters based on Mobile Crowdsensing
25