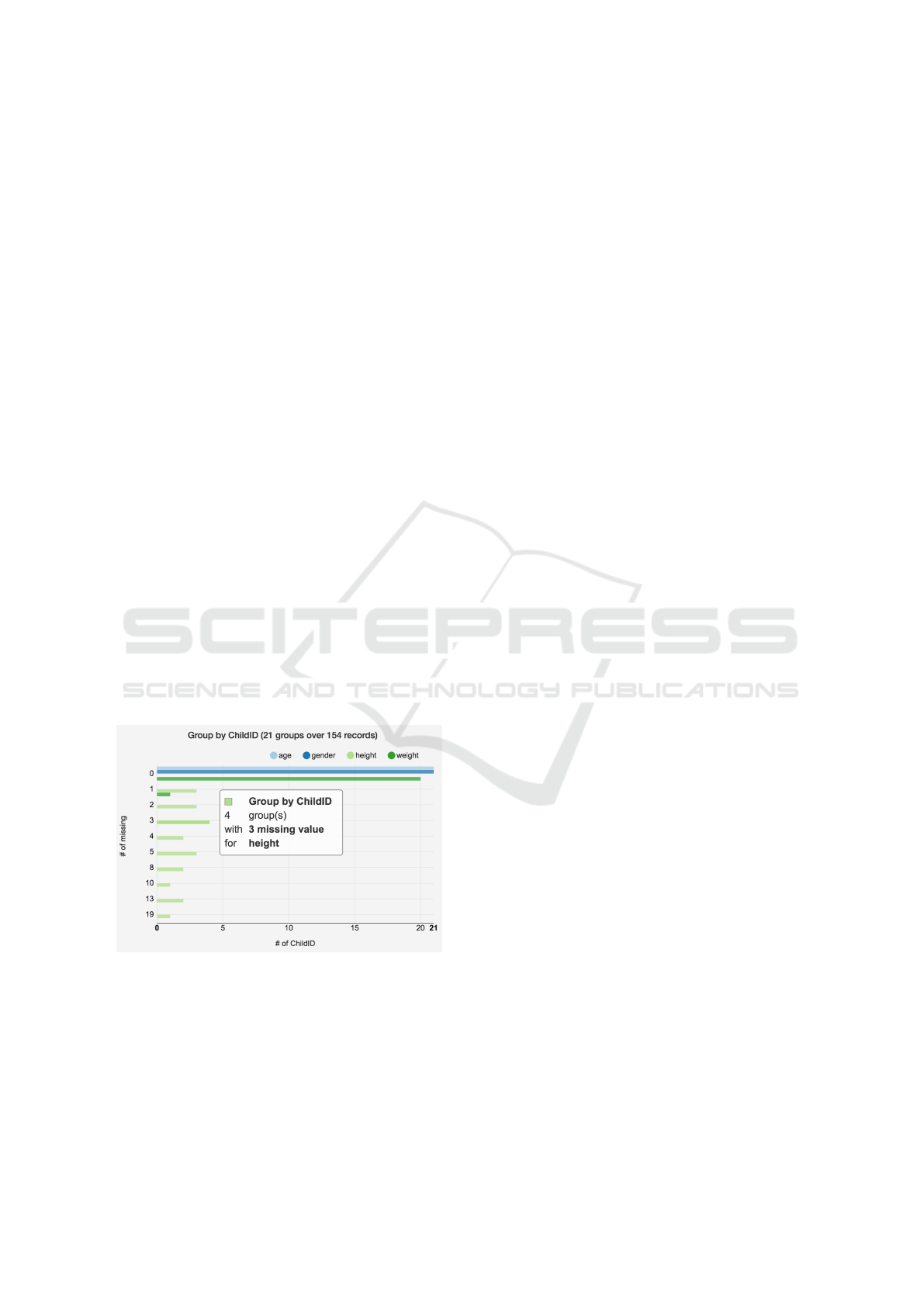
Overview. Then, they used the Group Filter to inves-
tigate missingness in the groups.
The next steps were to plot a chart, select some
variables in the Contextual Menu and evaluate the
differences between visualizing values in logic AND
and logic OR. Finally, they visualized the Distribution
charts.
The second set of exercises focused on the cor-
rectness functionality. Participants interacted with the
Correctness DQDM to dynamically create the visual-
izations and detect outliers.
5.2 Results
Overall, participants were impressed by the novel ca-
pabilities of MonAT. This section reports participants
main comments and suggestions.
Participants found the Present-Missing Overview
(Figure 1 VA.1) useful for understanding how miss-
ingness varied across variables, and especially having
the possibility to select a subset of the records. For ex-
ample, the chart shows that there is a similar amount
of missing data for the ethnicity variables (first five
black bars). By selecting one of these bars, the par-
ticipant can explore that similarity, and an example is
shown in Figure 2. Participants preferred stacked bars
for that rather than grouped bars.
The participants liked the Tables Overview (Fig-
ure 1 VA.2). However they recommended that it was
shown in a separate panel to leave the focus on the
visual chart.
Figure 4: The x axis shows the number of groups hav-
ing missing data for selected variables (age, gender, height,
weight). In this example there are 21 children (groups) over
154 records. The y axis shows the number of missing values
per variable. In this example, there are four children miss-
ing exactly three observations for the height. A mouse over
on bar displays a tooltip that shows related information.
The Grouped Present-Missing Data (Figure 1
VA.3 and Figure 4) shows the number of miss-
ing observations for selected variables (in this case
eth0eth9gp and height), grouped by the value of an-
other variable (in this case ‘childID’). Participants ini-
tially found it difficult to understand the meaning of
this visualization but, once they did, they considered
it to be useful for investigating whether groups had
sufficient values for a given task (Table 1.d and 1.e).
Participants suggested being able to interact with
bars in the Grouped Present-Missing Data (as it is
for the Present-Missing Overview) to show frequency
distributions of missing values. They also said they
would like to be able to select more than one group.
For example to include in the further analysis groups
having no missing values or not more than one value
missed for a given variable.
The scatterplot (Figures 3) shows missing and
present Data in Context. The contextual menu (at
the top of the Figure 3) allows users to include vari-
ables in the scatterplot. Participants found it useful to
visualize how categorical missing observations relate
to the scatterplot variables. For example (Figure 3a)
the three selected variables (eth0ethgr, ethgrp4 and
height) shown in AND, present missing data mostly
in early children days (from 0 to 300). Participants
considered it useful to be able to ‘AND’ or ‘OR’ the
selected variables, and suggested that ‘XOR’ (only
one of the selected variables is missing) would also
be useful.
Participants made a number of other comments,
which were as follows. The Distributions that are
shown in the bar charts of Figure 3 are difficult to see,
which could be addressed by binning the data to re-
duce the number of bars that are drawn.
Referring to the correctness exercises, the scatter-
plot and the scatterplot with lines (Figure 5) show
Data in Context to reveal outliers. Investigating the
data as single points may lead to some of them being
defined as outliers because they lie above the 99.9th
percentile (Figure 5a). However, a longitudinal visu-
alization indicates that some of those points are cor-
rect because they are from a single child and follow
a reasonable curve (Figure 5b). Participants found
these scatterplots useful to visualize the longitudinal
data to explore correctness. However, to improve the
legibility of a plot they suggested the use of bins to
visualize a subset of the data. The number of groups
(children) should be low to avoid confusion. More-
over, the longitudinal groups should be smartly se-
lected to avoid overlaps in the visualization.
Overall, participants found MonAT useful for pro-
filing the quality of the data. They suggested adding
‘print’ and ‘download’ function for each visualiza-
tion, so the visualizations can be easily included in
presentations and reports. Moreover, the tool can be
HEALTHINF 2017 - 10th International Conference on Health Informatics
32