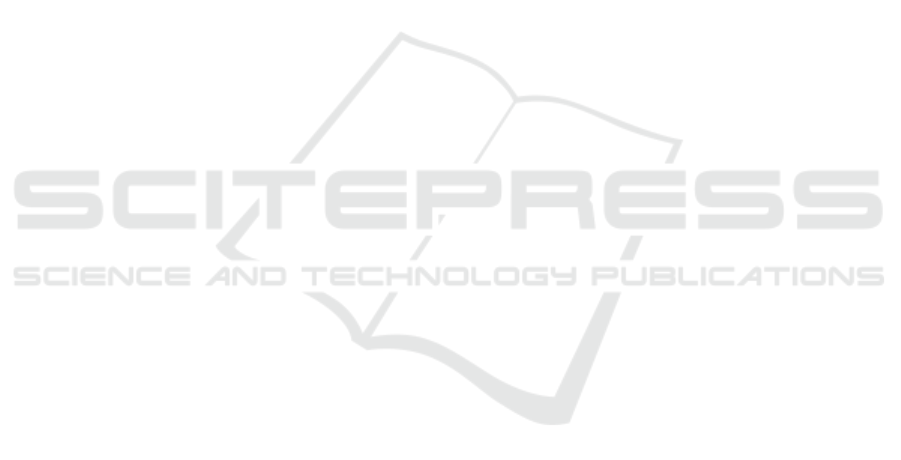
In Proceedings of the 12th ACM international confer-
ence on Ubiquitous computing, pages 311–320. ACM.
Babyak, M. A. (2004). What you see may not be what you
get: a brief, nontechnical introduction to overfitting
in regression-type models. Psychosomatic medicine,
66(3):411–421.
Bao, L. and Intille, S. S. (2004). Activity recognition from
user-annotated acceleration data. In Pervasive com-
puting, pages 1–17. Springer.
Baumann, K. (2003). Cross-validation as the objective
function for variable-selection techniques. TrAC
Trends in Analytical Chemistry, 22(6):395–406.
Brezmes, T., Gorricho, J.-L., and Cotrina, J. (2009). Ac-
tivity recognition from accelerometer data on a mo-
bile phone. In Distributed computing, artificial intel-
ligence, bioinformatics, soft computing, and ambient
assisted living, pages 796–799. Springer.
Campbell, A. and Choudhury, T. (2012). From smart to
cognitive phones. IEEE Pervasive Computing, (3):7–
11.
Chang, K.-H., Chen, M. Y., and Canny, J. (2007). Tracking
free-weight exercises. Springer.
Esterman, M., Tamber-Rosenau, B. J., Chiu, Y.-C., and
Yantis, S. (2010). Avoiding non-independence in fmri
data analysis: leave one subject out. Neuroimage,
50(2):572–576.
John, G. H. and Langley, P. (1995). Estimating continuous
distributions in bayesian classifiers. In Proceedings
of the Eleventh conference on Uncertainty in artificial
intelligence, pages 338–345. Morgan Kaufmann Pub-
lishers Inc.
Juan, A. and Ney, H. (2002). Reversing and smoothing the
multinomial naive bayes text classifier. In PRIS, pages
200–212.
Krishnan, N. C., Colbry, D., Juillard, C., and Panchanathan,
S. (2008). Real time human activity recognition us-
ing tri-axial accelerometers. In Sensors, signals and
information processing workshop.
Kuntze, G., Pias, M., Bezodis, I., Kerwin, D., Coulouris, G.,
and Irwin, G. (2009). Use of on-body sensors to sup-
port elite sprint coaching. International Association
of Computer Science in Sport, pages 71–75.
Langley, P., Iba, W., and Thompson, K. (1992). An analy-
sis of bayesian classifiers. In Aaai, volume 90, pages
223–228.
Lee, M. (2009). Physical activity recognition using a single
tri-axis accelerometer. In Proceedings of the world
congress on engineering and computer science, vol-
ume 1.
Lester, J., Choudhury, T., and Borriello, G. (2006). A prac-
tical approach to recognizing physical activities. In
Pervasive Computing, pages 1–16. Springer.
Long, X., Yin, B., and Aarts, R. M. (2009). Single-
accelerometer-based daily physical activity classifica-
tion. In Engineering in Medicine and Biology Society,
2009. EMBC 2009. Annual International Conference
of the IEEE, pages 6107–6110. IEEE.
McClaran, S. R. (2001). The effectiveness of personal train-
ing on changing attitudes towards physical activity.
Medicine & Science in Sports & Exercise, 33(5):S211.
Michahelles, F. and Schiele, B. (2005). Sensing and mon-
itoring professional skiers. Pervasive Computing,
IEEE, 4(3):40–45.
M
¨
oller, A., Roalter, L., Diewald, S., Scherr, J., Kranz,
M., Hammerla, N., Olivier, P., and Pl
¨
otz, T. (2012).
Gymskill: A personal trainer for physical exercises.
In Pervasive Computing and Communications (Per-
Com), pages 213–220. IEEE.
Parkka, J., Ermes, M., Korpipaa, P., Mantyjarvi, J., Peltola,
J., and Korhonen, I. (2006). Activity classification
using realistic data from wearable sensors. Informa-
tion Technology in Biomedicine, IEEE Transactions
on, 10(1):119–128.
Rasekh, A., Chen, C.-A., and Lu, Y. (2014). Human ac-
tivity recognition using smartphone. arXiv preprint
arXiv:1401.8212.
Ravi, N., Dandekar, N., Mysore, P., and Littman, M. L.
(2005). Activity recognition from accelerometer data.
In AAAI, volume 5, pages 1541–1546.
Saponas, T., Lester, J., Froehlich, J., Fogarty, J., and Lan-
day, J. (2008). ilearn on the iphone: Real-time human
activity classification on commodity mobile phones.
University of Washington CSE Tech Report UW-CSE-
08-04-02, 2008.
Sefen, B., Baumbach, S., Dengel, A., and Abdennadher,
S. (2016). Human activity recognition - using sensor
data of smartphones and smartwatches. In Proceed-
ings of the 8th International Conference on Agents
and Artificial Intelligence, volume 2, pages 488–493.
SCITEPRESS.
Sharma, A., Purwar, A., Lee, Y.-D., Lee, Y.-S., and Chung,
W.-Y. (2008). Frequency based classification of activ-
ities using accelerometer data. In Multisensor Fusion
and Integration for Intelligent Systems. IEEE Interna-
tional Conference on, pages 150–153. IEEE.
Shoaib, M., Scholten, H., and Havinga, P. J. (2013). To-
wards physical activity recognition using smartphone
sensors. In Ubiquitous Intelligence and Computing,
pages 80–87. IEEE.
Subramanya, A., Raj, A., Bilmes, J. A., and Fox, D. (2012).
Recognizing activities and spatial context using wear-
able sensors. arXiv preprint arXiv:1206.6869.
Wang, W.-z., Guo, Y.-w., Huang, B.-Y., Zhao, G.-r., Liu, B.-
q., and Wang, L. (2011). Analysis of filtering methods
for 3d acceleration signals in body sensor network. In
Bioelectronics and Bioinformatics (ISBB), 2011 Inter-
national Symposium on, pages 263–266. IEEE.
Witten, I. H. and Frank, E. (2005). Data Mining: Practi-
cal machine learning tools and techniques. Morgan
Kaufmann.
Yang, J. (2009). Toward physical activity diary: motion
recognition using simple acceleration features with
mobile phones. In Proceedings of the 1st interna-
tional workshop on Interactive multimedia for con-
sumer electronics, pages 1–10. ACM.
Measuring the Performance of Push-ups - Qualitative Sport Activity Recognition
381