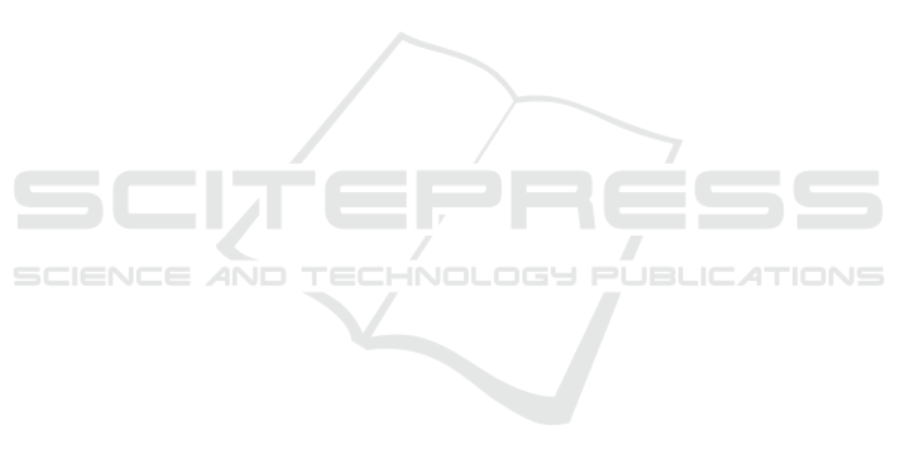
national Symposium on Biomedical Imaging (ISBI),
pages 896–899. IEEE.
Li, Q., Cai, W., Wang, X., Zhou, Y., Feng, D. D., and
Chen, M. (2014). Medical image classification with
convolutional neural network. In Control Automation
Robotics & Vision (ICARCV), 2014 13th International
Conference on, pages 844–848. IEEE.
Li, W., Jia, F., and Hu, Q. (2015). Automatic segmentation
of liver tumor in CT images with deep convolutional
neural networks. Journal of Computer and Communi-
cations, 3(11):146.
Liswoska, A., Beveridge, E., and Poole, I. (2016). False
positive reduction methods applied to dense vessel de-
tection in brain ct images. Poster presented at Medical
Imaging Summer School, Favigana, Italy.
O’Neil, A., Murphy, S., and Poole, I. (2015). Anatomical
landmark detection in ct data by learned atlas location
autocontext. In MIUA, pages 189–194.
Payan, A. and Montana, G. (2015). Predicting alzheimer’s
disease: a neuroimaging study with 3d convolutional
neural networks. arXiv preprint arXiv:1502.02506.
Pereira, S., Pinto, A., Alves, V., and Silva, C. A. (2015).
Deep convolutional neural networks for the segmen-
tation of gliomas in multi-sequence mri. In Inter-
national Workshop on Brainlesion: Glioma, Multiple
Sclerosis, Stroke and Traumatic Brain Injuries, pages
131–143. Springer.
Prasoon, A., Petersen, K., Igel, C., Lauze, F., Dam, E.,
and Nielsen, M. (2013). Deep feature learning for
knee cartilage segmentation using a triplanar convolu-
tional neural network. In Medical Image Computing
and Computer-Assisted Intervention–MICCAI 2013,
pages 246–253. Springer.
Qiu, W., Yuan, J., Ukwatta, E., Sun, Y., Rajchl, M., and Fen-
ster, A. (2013). Fast globally optimal segmentation
of 3d prostate mri with axial symmetry prior. In In-
ternational Conference on Medical Image Computing
and Computer-Assisted Intervention, pages 198–205.
Springer.
Rao, A., Ledig, C., Newcombe, V., Menon, D., and Rueck-
ert, D. (2014). Contusion segmentation from subjects
with traumatic brain injury: a random forest frame-
work. In 2014 IEEE 11th International Symposium
on Biomedical Imaging (ISBI), pages 333–336. IEEE.
Rao, V., Sarabi, M. S., and Jaiswal, A. (2015). Brain tu-
mor segmentation with deep learning. Proceedings of
BRATS-MICCAI.
Riedel, C. H., Zimmermann, P., Jensen-Kondering, U., Stin-
gele, R., Deuschl, G., and Jansen, O. (2011). The
importance of size successful recanalization by intra-
venous thrombolysis in acute anterior stroke depends
on thrombus length. Stroke, 42(6):1775–1777.
Roth, H. R., Lu, L., Seff, A., Cherry, K. M., Hoffman, J.,
Wang, S., Liu, J., Turkbey, E., and Summers, R. M.
(2014). A new 2.5 D representation for lymph node
detection using random sets of deep convolutional
neural network observations. In Medical Image Com-
puting and Computer-Assisted Intervention–MICCAI
2014, pages 520–527. Springer.
Shin, H.-C., Roth, H. R., Gao, M., Lu, L., Xu, Z., Nogues,
I., Yao, J., Mollura, D., and Summers, R. M. (2016).
Deep convolutional neural networks for computer-
aided detection: Cnn architectures, dataset charac-
teristics and transfer learning. IEEE transactions on
medical imaging, 35(5):1285–1298.
Urban, G., Bendszus, M., Hamprecht, F., and Kleesiek, J.
(2014). Multi-modal brain tumor segmentation using
deep convolutional neural networks. Proceedings of
BRATS-MICCAI.
Wang, J., MacKenzie, J. D., Ramachandran, R., and Chen,
D. Z. (2015). Neutrophils identification by deep learn-
ing and voronoi diagram of clusters. In Medical Im-
age Computing and Computer-Assisted Intervention–
MICCAI 2015, pages 226–233. Springer.
Wardlaw, J. M., Muir, K. W., Macleod, M.-J., Weir, C.,
McVerry, F., Carpenter, T., Shuler, K., Thomas, R.,
Acheampong, P., Dani, K., et al. (2013). Clinical rele-
vance and practical implications of trials of perfusion
and angiographic imaging in patients with acute is-
chaemic stroke: a multicentre cohort imaging study.
Journal of Neurology, Neurosurgery & Psychiatry,
pages jnnp–2012.
Wolterink, J. M., Leiner, T., Viergever, M. A., and I
ˇ
sgum,
I. (2015). Automatic coronary calcium scoring in car-
diac CT angiography using convolutional neural net-
works. In Medical Image Computing and Computer-
Assisted Intervention–MICCAI 2015, pages 589–596.
Springer.
Yang, D., Zhang, S., Yan, Z., Tan, C., Li, K., and Metaxas,
D. (2015). Automated anatomical landmark detection
on distal femur surface using convolutional neural net-
work. In Biomedical Imaging (ISBI), 2015 IEEE 12th
International Symposium on, pages 17–21. IEEE.
Zhao, L., Wu, W., and Corso, J. J. (2013). Semi-automatic
brain tumor segmentation by constrained mrfs using
structural trajectories. In International Conference on
Medical Image Computing and Computer-Assisted In-
tervention, pages 567–575. Springer.
Zikic, D., Glocker, B., Konukoglu, E., Criminisi, A., Demi-
ralp, C., Shotton, J., Thomas, O., Das, T., Jena, R.,
and Price, S. (2012). Decision forests for tissue-
specific segmentation of high-grade gliomas in multi-
channel mr. In International Conference on Medi-
cal Image Computing and Computer-Assisted Inter-
vention, pages 369–376. Springer.
Thrombus Detection in CT Brain Scans using a Convolutional Neural Network
33