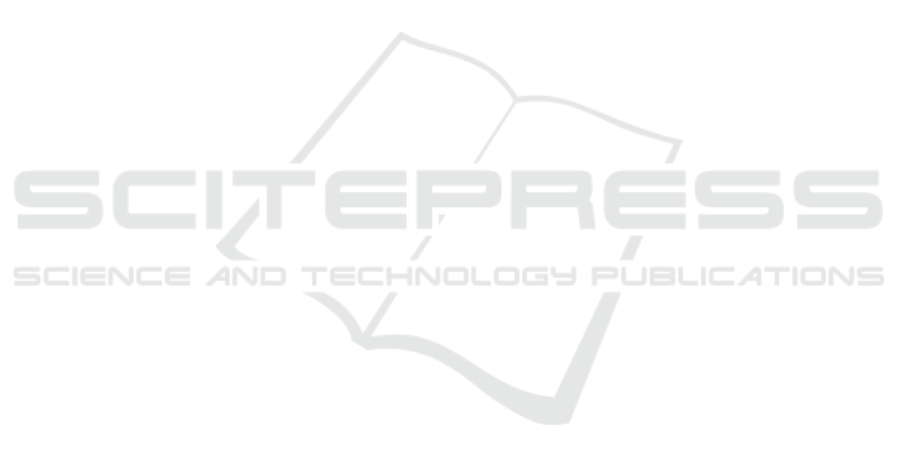
period no register data are digitally available in the ex-
tend needed. One has to take into account the exten-
sive migration movements during the last 100 years.
A possible solution might be given by aggregated dig-
ital administrative data of health and care insurances.
But precise resolution (day) is rarely available after
aggregation has been done for other reasons. The
first discussion of the influence of the weekday of
birth on a large data base was given in (Macfarlane,
1978) and (Mathers, 1983) using birth data of the
seventies, our data focuses on some decades before.
Furthermore the number of births with respect to the
weekday differs much from the current pattern. Re-
lated backgrounds are discussed in the stated refer-
ences (cf.(Kibele et al., 2013), (Klein et al., 2001),
(Klein and Unger, 2002), (Lampert and Kroll, 2014),
(Ma et al., 2012), (Mackenbach, 2006), (Schnell and
Trappmann, 2006), (Schuster and Emcke, 2016), (Os-
termann and Schuster, 2015)).
2 MATERIAL AND METHODS
We use health and care insurance data from a German
federal state. With respect to sufficient statistical sig-
nificance in the care insurance field we can go as far
back as people born in 1905 by using data from 1998
till 2006, in the health insurance data from 2006 one
can track back until 1920. Although we only need
aggregated data, such data with a weekday resolution
are rarely available.
We use the script language perl in order to aggregate
data and for the association of day of the week and
date. If we refer to birth rates with respect to months
we have to take into account their different lengths.
Gender was only available for the care insurance data.
The detailed insurance can be identified by a 9-digit
identification code (IK-number). We used a reference
table containing the insurance type in order to get a
known social indication.
If we use drug data, there is information about addi-
tional private payment of patients. Patients with low
social status have an additional payment exemption.
There is also a mixed status in which patients get an
additional payment exemption after having payed a
certain amount themselves. We are interested in the
social circumstances during birth, but we measure the
social status many years later. A Markov model for
transition of states would be useful. But there is no
real information about transition rates. If we assume
that the states are stable, we underestimate social ef-
fects.
Another type of analysis could combine low and high
risk at birth with a survival in the following cate-
gories: first three days after birth and mothers with
an age under or over 50 years. A derived, more de-
tailed refinement could lead to mortality tables in de-
pendence of the day and month of birth. Due to the
low availability of historical information this remains
a modeling challenge.
The time from the last menstrual period (LMP) to
childbirth is usually taken as 40 weeks or 280 days.
Pregnancy from conception to childbirth is 38 weeks
or 266 days long. But there are no large scale mea-
surements for mean values and standard deviations
and in particular about deviations from normal dis-
tribution. We can divide the population into two sub-
sets with respect to high and low pregnancy risk: X =
X
1
+X
2
as random variables. Let s(X) be the standard
deviation of X. We use s(X
1
) < s(X
2
). It is known
from literature that we have 9 < s(X) < 13. We use
s(X
1
) = 1,2, 3. X
1
leads to increasing peaks, X
2
gives
a nearly uniform variation to all days. If fertilization
data would be given, the distribution of the random
variable length of pregnancy would be a smoothing
parameter on cyclic space (with discretization to days
of week). But if we have given the birth data and
want to derive the weekday distribution of the fertil-
ization we get an inversion operator which tends to be
instable. Constraints lead to numerical stabilization.
We start with a quadratic-deviations model. Let f(i)
be the observed deviation from 1/7 for likelihood of
birth at day i (i = 0, 1,...,6) and w(i) the fertilization
deviation pattern at day i (i = 0, 1,..., 6). Than d
s
( j)
shall be the translation of j days by normal distribu-
tion with standard deviation s using integer intervals.
We look for the quadratic minimum:
6
∑
i=0
f (i) −
30
∑
j=−30
d(i − j)w( j)
!
2
−→ Min!
with the constraints −1 < −a < w(i) < b < 1. Prac-
tically we use a = b = 1/(7 ∗ 5) in order to limit the
deviation for each day with respect to the mean of the
week to 20 %. Alternatively we could use linear pro-
gramming:
f (i) −
30
∑
j=−30
d(i − j)w( j)
< s,s −→ Min!
For calculations we use Microsoft Excel and Mathe-
matica from Wolfram Research.
In order consider the different deviations during the
considered time period we use the concept of Shan-
non entropie
∑
6
i=0
− p
i
ln(p
i
) for the birth rates p
i
at day i. The same considerations we can adopt to
months instead of the weekdays. Alternative mea-
sures of the inequality are given by the Lorenz Curve
and the related Gini coefficient. In order to quan-
HEALTHINF 2017 - 10th International Conference on Health Informatics
42