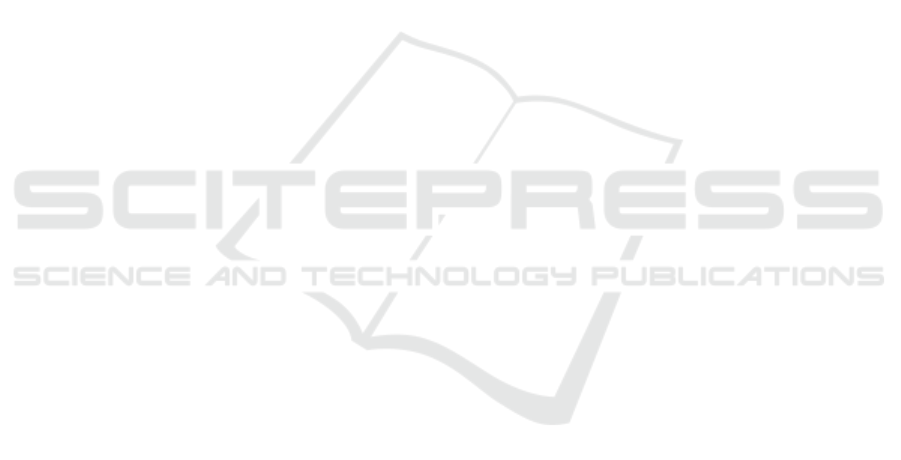
tured with a traditional image sensor. On the other
hand, a CIS modulates the temporal change of the
light strength at each pixel with the sinusoidal refer-
ence signals and records the temporal change with its
Fourier coefficients. Using these coefficients, one can
compute optical flow, v(x), and the difference of the
boundary values, F
0
(x), from a single image captured
by a CIS. Once one obtains v(x) and F
0
(x), one can
restore the higher temporal frequency components,
g
n
(x) (n ≥ 2), based mainly on the optical flow con-
straint, which represents the temporal invariance of
the strength of an incident light coming from an ob-
ject point.
The biggest limitation of the proposed method is
that the optical flow constraint (5) used in the pro-
posed method assumes that a flow observed at each
pixel, v(x), is constant with respect to time during
the exposure time. This is not true especially when
the motion blurs are generated with a high-frequency
motion such as a hand shake. Many blind motion de-
blurring methods can estimate spatial blurring kernels
from a single blurred image by introducing the prior
knowledge on natural images and/or on kernels. The
future works would include the use of the strategies
employed by those blind motion deblurring methods
for estimating the spatial blurring kernels that are con-
sistent not only with the blurred image g
0
(x) but also
with the Fourier coefficient image, g
1
(x), so that one
can restore more accurate and crisp images that rep-
resent the temporal change during the exposure time.
REFERENCES
Agrawal, A. and Raskar, R. (2009). Optimal single image
capture for motion deblurring. In Computer Vision
and Pattern Recognition, 2009. CVPR 2009. IEEE
Conference on , pages 2560–2567. IEEE.
Ando, S. and Kimachi, A. (2003). Correlation im-
age sensor: Two-dimensional matched detection of
amplitude-modulated light. Electron Devices, IEEE
Transactions on, 50(10):2059–2066.
Ando, S., Nakamura, T., and Sakaguchi, T. (1997). Ul-
trafast correlation image sensor: concept, design,
and applications. In Solid State Sensors and Actu-
ators, 1997. TRANSDUCERS’97 Chicago., 1997 In-
ternational Conference on, volume 1, pages 307–310.
IEEE.
Ayvaci, A., Raptis, M., and Soatto, S. (2012). Sparse occlu-
sion detection with optical flow. International Journal
of Computer Vision, 97(3):322–338.
Cai, J.-F., Ji, H., Liu, C., and Shen, Z. (2012). Framelet-
based blind motion deblurring from a single image.
Image Processing, IEEE Transactions on, 21(2):562–
572.
Cho, S. and Lee, S. (2009). Fast motion deblurring. In
ACM Transactions on Graphics (TOG), volume 28,
page 145. ACM.
Deshpande, A. M. and Patnaik, S. (2014). Uniform and non-
uniform single image deblurring based on sparse rep-
resentation and adaptive dictionary learning. The In-
ternational Journal of Multimedia & Its Applications
(IJMA), 6(01):47–60.
Fergus, R., Singh, B., Hertzmann, A., Roweis, S. T., and
Freeman, W. T. (2006). Removing camera shake from
a single photograph. ACM Transactions on Graphics
(TOG), 25(3):787–794.
Field, D. J. (1994). What is the goal of sensory coding?
Neural computation, 6(4):559–601.
Gupta, A., Joshi, N., Zitnick, C. L., Cohen, M., and Cur-
less, B. (2010). Single image deblurring using motion
density functions. In Computer Vision–ECCV 2010,
pages 171–184. Springer.
Heide, F., Hullin, M. B., Gregson, J., and Heidrich, W.
(2013). Low-budget transient imaging using photonic
mixer devices. ACM Transactions on Graphics (ToG),
32(4):45.
Hontani, H., Oishi, G., and Kitagawa, T. (2014). Local es-
timation of high velocity optical flow with correlation
image sensor. In Computer Vision–ECCV 2014, pages
235–249. Springer.
Jia, J. (2007). Single image motion deblurring using trans-
parency. In Computer Vision and Pattern Recogni-
tion, 2007. CVPR’07. IEEE Conference on, pages 1–
8. IEEE.
Joshi, N., Kang, S. B., Zitnick, C. L., and Szeliski, R.
(2010). Image deblurring using inertial measurement
sensors. In ACM Transactions on Graphics (TOG),
volume 29, page 30. ACM.
Kadambi, A., Whyte, R., Bhandari, A., Streeter, L., Barsi,
C., Dorrington, A., and Raskar, R. (2013). Coded
time of flight cameras: sparse deconvolution to ad-
dress multipath interference and recover time profiles.
ACM Transactions on Graphics (TOG), 32(6):167.
Levin, A. (2006). Blind motion deblurring using image
statistics. In Advances in Neural Information Process-
ing Systems, pages 841–848.
McCloskey, S., Ding, Y., and Yu, J. (2012). Design and esti-
mation of coded exposure point spread functions. Pat-
tern Analysis and Machine Intelligence, IEEE Trans-
actions on, 34(10):2071–2077.
Nayar, S. K. and Ben-Ezra, M. (2004). Motion-based mo-
tion deblurring. Pattern Analysis and Machine Intelli-
gence, IEEE Transactions on, 26(6):689–698.
Raskar, R., Agrawal, A., and Tumblin, J. (2006). Coded
exposure photography: motion deblurring using flut-
tered shutter. ACM Transactions on Graphics (TOG),
25(3):795–804.
Shan, Q., Jia, J., and Agarwala, A. (2008). High-quality mo-
tion deblurring from a single image. In ACM Transac-
tions on Graphics (TOG), volume 27, page 73. ACM.
Shan, Q., Xiong, W., and Jia, J. (2007). Rotational motion
deblurring of a rigid object from a single image. In
Computer Vision, 2007. ICCV 2007. IEEE 11th Inter-
national Conference on, pages 1–8. IEEE.
VISAPP 2017 - International Conference on Computer Vision Theory and Applications
190