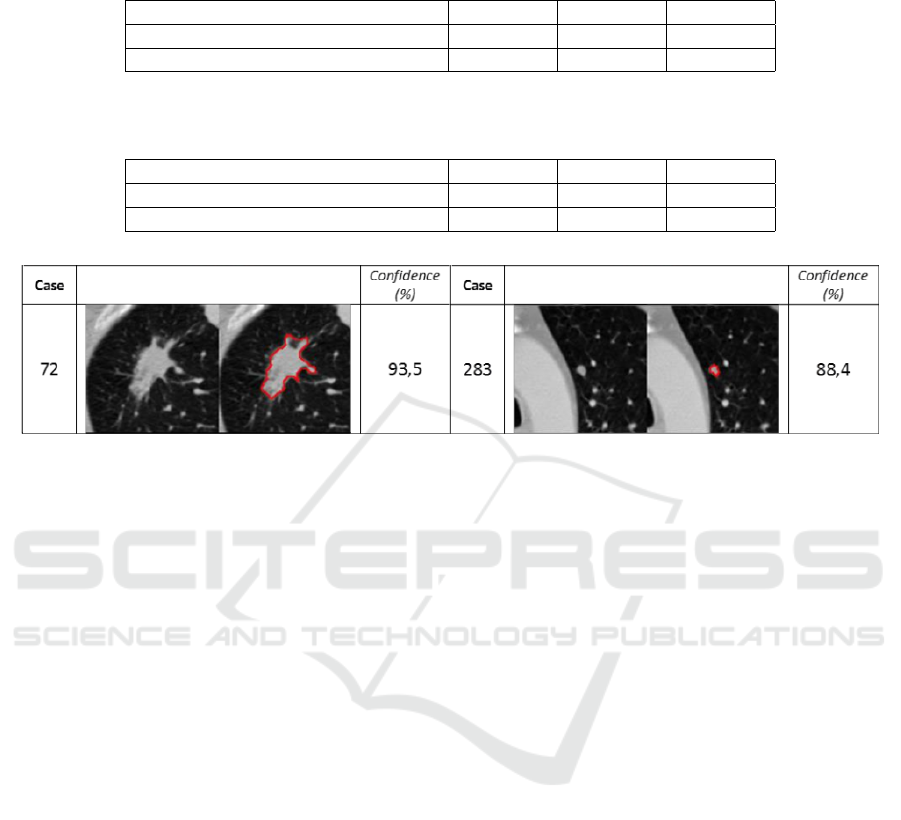
Table 2: Classification results presented as the mean and standard deviation of 50 AUC % values, for 12 features selected by
2 model searches and 3 KNNs.
Area under curve, AUC (%) 13-KNN 15-KNN 17-KNN
Correlation Feature Selection, CFS 93.2 ± 0.8 93.5 ± 0.9 94.1 ± 0.7
Relief F 94.7 ± 0.7 94.4 ± 0.8 94.4 ± 0.7
Table 3: Classification results presented as the mean and standard deviation of 50 AUC % values, for 12 features selected by
2 model searches and 3 SVMs.
Area under curve, AUC (%) 1-SVM 2-SVM 3-SVM
Correlation Feature Selection, CFS 96.2 ± 0.5 96.3 ± 0.6 96.4 ± 0.5
Relief F 96.0 ± 0.6 96.3 ± 0.6 96.2 ± 0.6
Figure 4: Examples of incorrect malignant12 (1
st
column) and malignant45 (2
nd
column) nodules class labels. Confidence is
presented as the posterior probability of a nodule belonging to a particular class (malignant12 or malignant45).
Also, other classifiers like neural networks should be
implemented for classification comparison.
ACKNOWLEDGEMENTS
This work is financed by the ERDF – European Re-
gional Development Fund through the Operational
Programme for Competitiveness and Internationalisa-
tion – COMPETE 2020 Programme, and by National
Funds through the Portuguese funding agency, FCT
– Fundac¸
˜
ao para a Ci
ˆ
encia e a Tecnologia, within
the project with code POCI-01-0145-FEDER-016673
and the grant contract SFRH/BPD/85663/2012 (J.
Novo).
REFERENCES
Akram, S., Javed, M., Hussain, A., Riaz, F., and Akram, M.
(2015). Intensity-based statistical features for classifi-
cation of lungs ct scan nodules using artificial intelli-
gence techniques. Journal of Experimental and Theo-
retical Artificial Intelligence, 27:737–751.
Armato, S., McLennan, G., Bidaut, L., McNitt-Gray, M.,
Meyer, C., Reeves, A., Zhao, B., Aberle, D., Hen-
schke, C., Hoffman, E., Kazerooni, E., MacMahon,
H., Beeke, E. V., Yankelevitz, D., Biancardi, A.,
Bland, P., Brown, M., Engelmann, R., Laderach, G.,
Max, D., Pais, R., Qing, D., Roberts, R., Smith, A.,
Starkey, A., Batrah, P., Caligiuri, P., Farooqi, A.,
Gladish, G., Jude, C., Munden, R., Petkovska, I.,
Quint, L., Schwartz, L., Sundaram, B., Dodd, L., Fen-
imore, C., Gur, D., Petrick, N., Freymann, J., Kirby,
J., Hughes, B., Casteele, A., Gupte, S., Sallamm, M.,
Heath, M., Kuhn, M., Dharaiya, E., Burns, R., Fryd,
D., Salganicoff, M., Anand, V., Shreter, U., Vastagh,
S., and Croft, B. (2011). The lung image database
consortium (LIDC) and image database resource ini-
tiative (IDRI): a completed reference database of lung
nodules on CT scans. Medical Physics, 38:915–931.
Breadsmoore, C. J. and Screaton, N. J. (2003). Classifica-
tion, staging and prognosis of lung cancer. European
Journal of Radiology, 45:8–17.
Gonc¸alves, L., Novo, J., and Campilho, A. (2016). Hessian
based approaches for 3D lung nodule segmentation.
Expert Systems with Applications, 61:1–15.
Hall, M. A. (1999). Correlation-based feature selection
for machine learning. PhD thesis, The University of
Waikato.
Kaya, A. and Can, A. (2015). A weighted rule based method
for predicting malignancy of pulmonary nodules by
nodule characteristics. Journal of Biomedical Infor-
matics, 56:69–79.
Liu, X., Ma, L., Song, L., Zhao, Y., Zhao, X., and Zhou,
C. (2015). Recognizing common ct imaging signs of
lung diseases through a new feature selection method
based on fisher criterion and genetic optimization.
IEEE Journal of Biomedical and Health Informatics,
19:635–647.
Novo, J., Gonc¸alves, L., Mendonc¸a, A., and Campilho, A.
(2015). 3D lung nodule candidates detection in mul-
Evaluation of the Degree of Malignancy of Lung Nodules in Computed Tomography Images
79