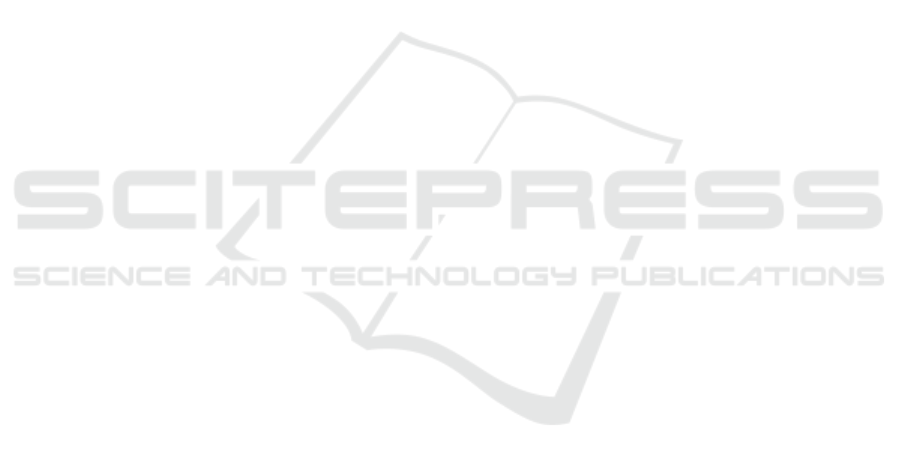
Horizon 2020 Research and Innovation Programme
under Grant Agreement N 690090 (ICT4Life project).
REFERENCES
Abrams, A., Tucek, J., Jacobs, N., and Pless, R.
(2012). LOST: Longterm Observation of Scenes (with
Tracks). In IEEE Workshop on Applications of Com-
puter Vision (WACV), pages 297–304.
Bermejo, E., Deniz, O., Bueno, G., and Sukthankar, R.
(2011). Violence detection in video using computer
vision techniques. In Int. Conf. on Computer Analysis
of Images and Patterns, pages 332–339.
Deng, Z.-A., Hu, Y., Yu, J., and Na, Z. (2015). Extended
Kalman filter for real time indoor localization by fus-
ing WiFi and smartphone inertial sensors. Microma-
chines, 6:523–543.
Efros, A., Berg, A., Mori, G., and Malik, J. (2003). Rec-
ognizing action at a distance. In IEEE Int. Conf. on
Computer Vision, pages 726–733.
Hoque, E., Dickerson, R. F., Preum, S. M., Hanson, M.,
Barth, A., and Stankovic, J. A. (2015). Holmes: A
comprehensive anomaly detection system for daily in-
home activities. In 11th IEEE Int. Conf. on Distributed
Computing in Sensor Systems.
Jiang, F., Wu, Y., and Katsaggelos, A. K. (2009). A dy-
namic hierarchical clustering method for trajectory-
based unusual video event detection. IEEE Trans. on
Image Processing, 18(4):907–913.
Kasteren, T. v., Englebienne, G., and Krse, B. (2010). Ac-
tivity recognition using semi-markov models on real
world smart home datasets. J. Ambient Intell. Smart
Environ., 2:311–325.
Li, W., Mahadevan, V., and Vasconcelos, N. (2014).
Anomaly detection and localization in crowded
scenes. IEEE Trans. on Pattern Analysis and Machine
Intelligence (PAMI), 36(1):18–32.
Masci, J., Meier, U., Ciresan, D., and Schmidhuber, J.
(2011). Stacked convolutional auto-encoders for hi-
erarchical feature extraction. In 21th Int. Conf. on Ar-
tificial Neural Networks (ICAN’11), pages 52–59.
Microsoft Kinect SDK. https://msdn.microsoft.com/en-
us/library/microsoft.kinect.kinect.jointtype.aspx.
Mousavi, H., M., Perina, A., Chellali, R., and Mur, V.
(2015). Analyzing tracklets for the detection of ab-
normal crowd behavior. In Proc. of the IEEE Win-
ter Conf. on Applications of Computer Vision (WACV
2015), pages 148–155.
Nef, T., Urwyler, P., Bchler, M., Tarnanas, I., Stucki, R.,
Cazzoli, D., Mri, R., and Mosimann, U. (2015). Eval-
uation of Three State-of-the-Art Classifiers for Recog-
nition of Activities of Daily Living from Smart Home
Ambient Data. Sensors, 15(5):11725–11740.
Ngiam, J., Khosla, A., and Kim, M. (2010). Multimodal
deep learning. NIPS 2010 Workshop on Deep Learn-
ing and Unsupervised Feature Learning, pages 1–9.
Nievas, E. B., Suarez, O. D., Garc
´
ıa, G. B., and Sukthankar,
R. (2011). Violence detection in video using computer
vision techniques. In Proc. of the 14th Int. Conf. on
Computer Analysis of Images and Patterns (CAIP’11),
pages 332–339.
Paliyawan, P., Nukoolkit, C., and Mongkolnam, P. (2014).
Prolonged Sitting Detection for Office Workers Syn-
drome Prevention Using Kinect. In 11th Int.
Conf. on Electrical Engineering/Electronics, Com-
puter, Telecommunications and Information Technol-
ogy (ECTI-CON), pages 1–6.
Raptis, M. and Soatto, S. (2010). Tracklet descriptors for
action modeling and video analysis. Lecture Notes in
Computer Science (LNCS), 6311:577–590.
Saenz-de Urturi, Z. and Soto, G. Z. B. (2016). Kinect-Based
Virtual Game for the Elderly that Detects Incorrect
Body Postures in Real Time. Sensors, 16(5).
See, J. and Tan, S. (2014). Lost World: Looking for
Anomalous Tracks in Long-term Surveillance Videos.
In Proc. of the Image and Vision Computing New
Zealand (IVCNZ), pages 224–229.
Shin, J., Kim, S., Kang, S., Lee, S.-W., Paik, J., Abidi, B.,
and Abidi, M. (2005). Optical flow-based real-time
object tracking using non-prior training active feature
model. RealTime Imaging, 11(3):204–218.
Uribe-Quevedo, A., Perez-Gutierrez, B., and Guerrero-
Rincon, C. (2013). Seated tracking for correcting
computer work postures. In 29th Southern Biomed-
ical Engineering Conf. (SBEC), pages 169–170.
Wong, K. B.-Y., Zhang, T., and Aghajan, H. (2014). Data
Fusion with a Dense Sensor Network for Anomaly
Detection in Smart Homes. Human Behavior Under-
standing in Networked Sensing, pages 45–73.
Yang, L., Ren, Y., and Zhang, W. (2016). 3D depth im-
age analysis for indoor fall detection of elderly people.
Digital Communications and Networks, 2(1):24–34.
Zhou, Z., Chen, X., Chung, Y.-C., He, Z., Han, T. X., and
Keller, J. M. (2008). Activity analysis, summariza-
tion, and visualization for indoor human activity mon-
itoring. IEEE Trans. on Circuits and Systems for Video
Technology, 18(11):1489–1498.
Unsupervised Discovery of Normal and Abnormal Activity Patterns in Indoor and Outdoor Environments
217