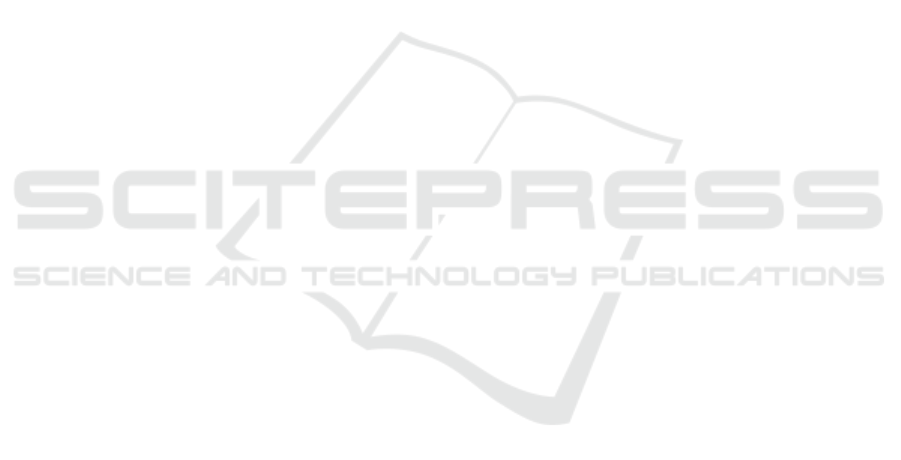
inatively trained part based models. IEEE Transac-
tions on Pattern Analysis and Machine Intelligence,
32(9):1627–1645.
Girshick, R., Donahue, J., Darrell, T., and Malik, J. (2014).
Rich feature hierarchies for accurate object detection
and semantic segmentation. In Computer Vision and
Pattern Recognition.
Grabner, H., Leistner, C., and Bischof, H. (2008). Semi-
supervised on-line boosting for robust tracking. In
Proceedings of the 10th European Conference on
Computer Vision: Part I, ECCV ’08, pages 234–247.
Han, B., Comaniciu, D., Zhu, Y., and Davis, L. S. (2008).
Sequential kernel density approximation and its ap-
plication to real-time visual tracking. IEEE Transac-
tions on Pattern Analysis and Machine Intelligence,
30(7):1186–1197.
Hare, S., Saffari, A., and Torr, P. H. S. (2011). Struck:
Structured output tracking with kernels. In 2011 Inter-
national Conference on Computer Vision, pages 263–
270.
Henriques, J. a. F., Caseiro, R., Martins, P., and Batista, J.
(2012). Exploiting the circulant structure of tracking-
by-detection with kernels. In Proceedings of the 12th
European Conference on Computer Vision - Volume
Part IV, ECCV’12, pages 702–715.
Henriques, J. F., Caseiro, R., Martins, P., and Batista, J.
(2015). High-speed tracking with kernelized corre-
lation filters. Pattern Analysis and Machine Intelli-
gence, IEEE Transactions on.
Idrees, H., Warner, N., and Shah, M. (2014). Tracking
in dense crowds using prominence and neighborhood
motion concurrence. Image and Vision Computing,
32(1):14 – 26.
Jia, X., Lu, H., and Yang, M. H. (2012). Visual tracking
via adaptive structural local sparse appearance model.
In Computer Vision and Pattern Recognition (CVPR),
2012 IEEE Conference on, pages 1822–1829.
Kalal, Z., Mikolajczyk, K., and Matas, J. (2012). Tracking-
learning-detection. IEEE Transactions on Pattern
Analysis and Machine Intelligence, 34(7):1409–1422.
Kratz, L. and Nishino, K. (2012). Tracking pedestrians us-
ing local spatio-temporal motion patterns in extremely
crowded scenes. IEEE Transactions on Pattern Anal-
ysis and Machine Intelligence, 34(5):987–1002.
Li, Y. and Zhu, J. (2015). A Scale Adaptive Kernel Corre-
lation Filter Tracker with Feature Integration, chapter
Computer Vision - ECCV 2014 Workshops: Zurich,
Switzerland, September 6-7 and 12, 2014, Proceed-
ings, Part II, pages 254–265. Cham.
Ma, C., Huang, J. B., Yang, X., and Yang, M. H. (2015a).
Hierarchical convolutional features for visual track-
ing. In 2015 IEEE International Conference on Com-
puter Vision (ICCV), pages 3074–3082.
Ma, C., Yang, X., Zhang, C., and Yang, M. H. (2015b).
Long-term correlation tracking. In 2015 IEEE Con-
ference on Computer Vision and Pattern Recognition
(CVPR), pages 5388–5396.
Rifkin, R., Yeo, G., and Poggio, T. (2003). Regularized
least-squares classification. Nato Science Series Sub
Series III Computer and Systems Sciences, 190:131–
154.
Ristic, B., Clark, D. E., Vo, B.-N., and Vo, B.-T. (2012).
Adaptive target birth intensity for PHD and CPHD fil-
ters. IEEE Transactions on Aerospace and Electronic
Systems, 48(2):1656–1668.
Rodriguez, M., Sivic, J., Laptev, I., and Audibert, J.-Y.
(2011). Density-aware person detection and tracking
in crowds. In Proceedings of the International Con-
ference on Computer Vision (ICCV).
Ross, D. A., Lim, J., Lin, R.-S., and Yang, M.-H. (2008).
Incremental learning for robust visual tracking. Inter-
national Journal of Computer Vision, 77(1):125–141.
Simonyan, K. and Zisserman, A. (2015). Very deep con-
volutional networks for large-scale image recognition.
ICLR.
Smeulders, A. W. M., Chu, D. M., Cucchiara, R., Calder-
ara, S., Dehghan, A., and Shah, M. (2014). Visual
tracking: An experimental survey. IEEE Transac-
tions on Pattern Analysis and Machine Intelligence,
36(7):1442–1468.
van de Weijer, J., Schmid, C., Verbeek, J., and Larlus, D.
(2009). Learning color names for real-world applica-
tions. Trans. Img. Proc., 18(7):1512–1523.
Vedaldi, A. and Lenc, K. (2015). MatConvNet – convo-
lutional neural networks for matlab. In Proceedings
of the 25th annual ACM international conference on
Multimedia.
Vo, B.-N. and Ma, W.-K. (2006). The Gaussian mixture
probability hypothesis density filter. Signal Process-
ing, IEEE Transactions on, 54(11):4091–4104.
Wang, L., Ouyang, W., Wang, X., and Lu, H. (2015). Visual
tracking with fully convolutional networks. In 2015
IEEE International Conference on Computer Vision
(ICCV), pages 3119–3127.
Wang, N. and Yeung, D.-Y. (2013). Learning a deep
compact image representation for visual tracking. In
Burges, C. J. C., Bottou, L., Welling, M., Ghahra-
mani, Z., and Weinberger, K. Q., editors, Advances
in Neural Information Processing Systems 26, pages
809–817.
Wu, Y., Lim, J., and Yang, M. H. (2013). Online object
tracking: A benchmark. In Computer Vision and Pat-
tern Recognition (CVPR), 2013 IEEE Conference on,
pages 2411–2418.
Zhang, J., Ma, S., and Sclaroff, S. (2014). MEEM: robust
tracking via multiple experts using entropy minimiza-
tion. In Proc. of the European Conference on Com-
puter Vision (ECCV).
Zhang, T., Ghanem, B., Liu, S., and Ahuja, N. (2012).
Robust visual tracking via multi-task sparse learning.
In Computer Vision and Pattern Recognition (CVPR),
2012 IEEE Conference on, pages 2042–2049.
Zhong, W., Lu, H., and Yang, M. H. (2012). Robust ob-
ject tracking via sparsity-based collaborative model.
In Computer Vision and Pattern Recognition (CVPR),
2012 IEEE Conference on, pages 1838–1845.
Long-term Correlation Tracking using Multi-layer Hybrid Features in Dense Environments
203