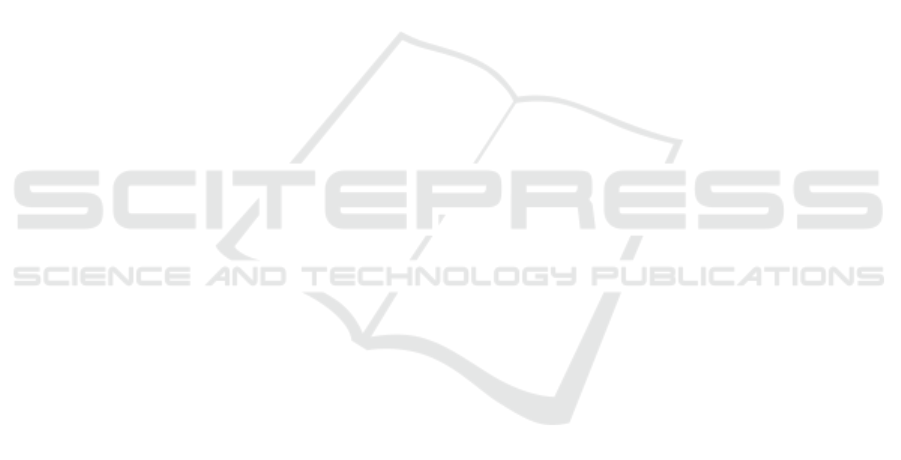
Proceedings of SPIE - The International Society for
Optical Engineering, 7150(10):2752–65.
Dalal, N. and Triggs, B. (2005). Histograms of oriented gra-
dients for human detection. In Proc. IEEE Int. Conf.
Comput. Vis. Pattern Recognit., volume 1, pages 886–
893. IEEE.
Gan, L., Liu, P., and Wang, L. (2015). Rotation sliding win-
dow of the hog feature in remote sensing images for
ship detection. In 2015 8th International Symposium
on Computational Intelligence and Design (ISCID),
volume 1, pages 401–404. IEEE.
Girshick, R. (2015). Fast r-cnn. In Proc. IEEE Int. Conf.
Comput. Vis., pages 1440–1448.
Girshick, R., Donahue, J., Darrell, T., and Malik, J. (2014).
Rich feature hierarchies for accurate object detection
and semantic segmentation. In Proc. IEEE Int. Conf.
Comput. Vis. Pattern Recognit., pages 580–587.
Guo, W., Xia, X., and Wang, X. (2014). A remote sensing
ship recognition method based on dynamic probability
generative model. Expert Systems with Applications,
41(14):6446–6458.
Han, J., Zhang, D., Cheng, G., Guo, L., and Ren, J. (2015).
Object detection in optical remote sensing images
based on weakly supervised learning and high-level
feature learning. IEEE Trans. Geosci. Remote Sens.,
53(6):3325–3337.
Krizhevsky, A., Sutskever, I., and Hinton, G. E. (2012). Im-
agenet classification with deep convolutional neural
networks. In Advances in neural information process-
ing systems, pages 1097–1105.
Liu, G., Zhang, Y., Zheng, X., Sun, X., Fu, K., and Wang,
H. (2014). A new method on inshore ship detection in
high-resolution satellite images using shape and con-
text information. IEEE Geosci. Remote Sens. Lett.,
11(3):617–621.
Liu, P. (2015). A survey of remote-sensing big data. Fron-
tiers in Environmental Science, 3:45.
Liu, Z., Wang, H., Weng, L., and Yang, Y. (2016). Ship
rotated bounding box space for ship extraction from
high-resolution optical satellite images with com-
plex backgrounds. IEEE Geosci. Remote Sens. Lett.,
13(8):1074–1078.
Otsu, N. (1975). A threshold selection method from gray-
level histograms. Automatica, 11(285-296):23–27.
Proia, N. and Page, V. (2010). Characterization of a
bayesian ship detection method in optical satellite im-
ages. IEEE Geosci. Remote Sens. Lett., 7(2):226–230.
Qi, S., Ma, J., Lin, J., and Li, Y. (2015). Unsupervised ship
detection based on saliency and s-hog descriptor from
optical satellite images. IEEE Geosci. Remote Sens.
Lett., 12(7):1–5.
Ren, S., He, K., Girshick, R., and Sun, J. (2015). Faster
r-cnn: Towards real-time object detection with region
proposal networks. In Advances in neural information
processing systems, pages 91–99.
Shi, Z., Yu, X., Jiang, Z., and Li, B. (2014). Ship detection
in high-resolution optical imagery based on anomaly
detector and local shape feature. IEEE Trans. Geosci.
Remote Sens., 52(8):4511–4523.
Tang, J., Deng, C., Huang, G. B., and Zhao, B. (2015).
Compressed-domain ship detection on spaceborne op-
tical image using deep neural network and extreme
learning machine. IEEE Trans. Geosci. Remote Sens.,
53(3):1174–1185.
Van Horn, G., Branson, S., Farrell, R., Haber, S., Barry,
J., Ipeirotis, P., Perona, P., and Belongie, S. (2015).
Building a bird recognition app and large scale dataset
with citizen scientists: The fine print in fine-grained
dataset collection. In Proc. IEEE Int. Conf. Comput.
Vis. Pattern Recognit., pages 595–604.
Xu, J., Sun, X., Zhang, D., and Fu, K. (2014). Auto-
matic detection of inshore ships in high-resolution re-
mote sensing images using robust invariant general-
ized hough transform. IEEE Geosci. Remote Sens.
Lett., 11(12):2070–2074.
Yang, G., Li, B., Ji, S., Gao, F., and Xu, Q. (2014). Ship
detection from optical satellite images based on sea
surface analysis. IEEE Geosci. Remote Sens. Lett.,
11(3):641–645.
Yang, L., Luo, P., Change Loy, C., and Tang, X. (2015). A
large-scale car dataset for fine-grained categorization
and verification. In Proc. IEEE Int. Conf. Comput. Vis.
Pattern Recognit., pages 3973–3981.
Yang, Y. and Newsam, S. (2010). Bag-of-visual-words and
spatial extensions for land-use classification. In Pro-
ceedings of the 18th SIGSPATIAL international con-
ference on advances in geographic information sys-
tems, pages 270–279. ACM.
Yokoya, N. and Iwasaki, A. (2015). Object detection based
on sparse representation and hough voting for opti-
cal remote sensing imagery. IEEE Journal of Selected
Topics in Applied Earth Observations & Remote Sens-
ing, 8(5):1–1.
You, X. and Li, W. (2011). A sea-land segmentation
scheme based on statistical model of sea. In Inter-
national Congress on Image and Signal Processing,
pages 1155–1159.
Yu, Y., Guan, H., and Ji, Z. (2015). Rotation-invariant
object detection in high-resolution satellite imagery
using superpixel-based deep hough forests. IEEE
Geosci. Remote Sens. Lett., 12(11):2183–2187.
Zhang, R., Yao, J., Zhang, K., Feng, C., and Zhang, J.
(2016). S-cnn ship detection from high-resolution re-
mote sensing images. ISPRS - International Archives
of the Photogrammetry, Remote Sensing and Spatial
Information Sciences.
Zhu, C., Zhou, H., Wang, R., and Guo, J. (2010). A novel
hierarchical method of ship detection from spaceborne
optical image based on shape and texture features.
IEEE Trans. Geosci. Remote Sens., 48(9):3446–3456.
Zou, Z. and Shi, Z. (2016). Ship detection in spaceborne
optical image with svd networks. IEEE Trans. Geosci.
Remote Sens., 54(10):5832–5845.
A High Resolution Optical Satellite Image Dataset for Ship Recognition and Some New Baselines
331